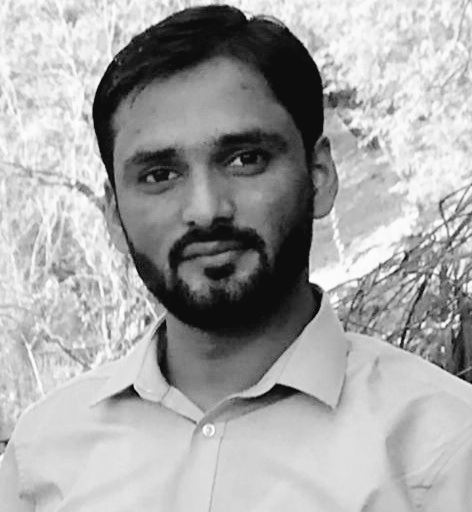
Ph.D. Student in Modeling and Data Science, University of Turin
Parallel Computing group
Via Pessinetto 12, 10149 Torino – Italy
Email: yasir.arfat@unito.it
Yasir Arfat from Kashmir, Pakistan is a holder of BS degree in Software Engineering from University of Azad Jammu & Kashmir, Muzaffarabad, Pakistan, 2011 and Master degree in computer science from King Abdulaziz University, Jeddah, Saudi Arabia, 2017. Now, he is currently pursuing his PhD degree from the University of Turin (UniTo). He was awarded “Shining Star” for his excellent performance in 2009 and was a “Gold Medallist” for the highest CGPA in bachelor degree.
He has four-year experience in software development. He was a software engineer with ActiveXoft and Lambda Technologies, Lahore from 2011-2014 and an iOS Mobile application developer with Dunay TV, Lahore from 2014 to 2015. He also has two years of research experience, working at high-performance computer centre (HPCC) KAU.
His main areas of interest are data analytics, distributed and parallel computing, machine learning, data science and software engineering.
Publications
2023
- I. Colonnelli, B. Casella, G. Mittone, Y. Arfat, B. Cantalupo, R. Esposito, A. R. Martinelli, D. Medić, and M. Aldinucci, “Federated Learning meets HPC and cloud,” in Astrophysics and Space Science Proceedings, Catania, Italy, 2023, p. 193–199. doi:10.1007/978-3-031-34167-0_39
[BibTeX] [Abstract] [Download PDF]
HPC and AI are fated to meet for several reasons. This article will discuss some of them and argue why this will happen through the set of methods and technologies that underpin cloud computing. As a paradigmatic example, we present a new federated learning system that collaboratively trains a deep learning model in different supercomputing centers. The system is based on the StreamFlow workflow manager designed for hybrid cloud-HPC infrastructures.
@inproceedings{22:ml4astro, abstract = {HPC and AI are fated to meet for several reasons. This article will discuss some of them and argue why this will happen through the set of methods and technologies that underpin cloud computing. As a paradigmatic example, we present a new federated learning system that collaboratively trains a deep learning model in different supercomputing centers. The system is based on the StreamFlow workflow manager designed for hybrid cloud-HPC infrastructures.}, address = {Catania, Italy}, author = {Iacopo Colonnelli and Bruno Casella and Gianluca Mittone and Yasir Arfat and Barbara Cantalupo and Roberto Esposito and Alberto Riccardo Martinelli and Doriana Medi\'{c} and Marco Aldinucci}, booktitle = {Astrophysics and Space Science Proceedings}, date-modified = {2024-04-01 11:12:57 +0200}, doi = {10.1007/978-3-031-34167-0_39}, editor = {Bufano, Filomena and Riggi, Simone and Sciacca, Eva and Schilliro, Francesco}, isbn = {978-3-031-34167-0}, keywords = {across, eupilot, streamflow}, pages = {193--199}, publisher = {Springer}, title = {Federated Learning meets {HPC} and cloud}, url = {https://iris.unito.it/retrieve/3ac66baa-9d9a-4e9f-94a5-13700694d8aa/ML4Astro.pdf}, volume = {60}, year = {2023}, bdsk-url-1 = {https://iris.unito.it/retrieve/3ac66baa-9d9a-4e9f-94a5-13700694d8aa/ML4Astro.pdf} }
- Y. Arfat, G. Mittone, I. Colonnelli, F. D’Ascenzo, R. Esposito, and M. Aldinucci, “Pooling critical datasets with Federated Learning,” in 31st Euromicro International Conference on Parallel, Distributed and Network-Based Processing, PDP 2023, Napoli, Italy, 2023, p. 329–337. doi:10.1109/PDP59025.2023.00057
[BibTeX] [Abstract] [Download PDF]
Federated Learning (FL) is becoming popular in different industrial sectors where data access is critical for security, privacy and the economic value of data itself. Unlike traditional machine learning, where all the data must be globally gathered for analysis, FL makes it possible to extract knowledge from data distributed across different organizations that can be coupled with different Machine Learning paradigms. In this work, we replicate, using Federated Learning, the analysis of a pooled dataset (with AdaBoost) that has been used to define the PRAISE score, which is today among the most accurate scores to evaluate the risk of a second acute myocardial infarction. We show that thanks to the extended-OpenFL framework, which implements AdaBoost.F, we can train a federated PRAISE model that exhibits comparable accuracy and recall as the centralised model. We achieved F1 and F2 scores which are consistently comparable to the PRAISE score study of a 16- parties federation but within an order of magnitude less time.
@inproceedings{23:praise-fl:pdp, abstract = {Federated Learning (FL) is becoming popular in different industrial sectors where data access is critical for security, privacy and the economic value of data itself. Unlike traditional machine learning, where all the data must be globally gathered for analysis, FL makes it possible to extract knowledge from data distributed across different organizations that can be coupled with different Machine Learning paradigms. In this work, we replicate, using Federated Learning, the analysis of a pooled dataset (with AdaBoost) that has been used to define the PRAISE score, which is today among the most accurate scores to evaluate the risk of a second acute myocardial infarction. We show that thanks to the extended-OpenFL framework, which implements AdaBoost.F, we can train a federated PRAISE model that exhibits comparable accuracy and recall as the centralised model. We achieved F1 and F2 scores which are consistently comparable to the PRAISE score study of a 16- parties federation but within an order of magnitude less time.}, address = {Napoli, Italy}, author = {Yasir Arfat and Gianluca Mittone and Iacopo Colonnelli and Fabrizio D'Ascenzo and Roberto Esposito and Marco Aldinucci}, booktitle = {31st Euromicro International Conference on Parallel, Distributed and Network-Based Processing, {PDP} 2023}, date-added = {2023-02-04 18:16:36 +0100}, date-modified = {2024-04-01 11:14:10 +0200}, doi = {10.1109/PDP59025.2023.00057}, keywords = {admire, hpc4ai, confidential}, pages = {329--337}, publisher = {IEEE}, title = {Pooling critical datasets with Federated Learning}, url = {https://iris.unito.it/retrieve/491e22ec-3db5-4989-a063-085a199edd20/23_pdp_fl.pdf}, year = {2023}, bdsk-url-1 = {https://iris.unito.it/retrieve/491e22ec-3db5-4989-a063-085a199edd20/23_pdp_fl.pdf} }
2022
- G. Agosta, M. Aldinucci, C. Alvarez, R. Ammendola, Y. Arfat, O. Beaumont, M. Bernaschi, A. Biagioni, T. Boccali, B. Bramas, C. Brandolese, B. Cantalupo, M. Carrozzo, D. Cattaneo, A. Celestini, M. Celino, I. Colonnelli, P. Cretaro, P. D’Ambra, M. Danelutto, R. Esposito, L. Eyraud-Dubois, A. Filgueras, W. Fornaciari, O. Frezza, A. Galimberti, F. Giacomini, B. Goglin, D. Gregori, A. Guermouche, F. Iannone, M. Kulczewski, F. Lo Cicero, A. Lonardo, A. R. Martinelli, M. Martinelli, X. Martorell, G. Massari, S. Montangero, G. Mittone, R. Namyst, A. Oleksiak, P. Palazzari, P. S. Paolucci, F. Reghenzani, C. Rossi, S. Saponara, F. Simula, F. Terraneo, S. Thibault, M. Torquati, M. Turisini, P. Vicini, M. Vidal, D. Zoni, and G. Zummo, “Towards EXtreme scale technologies and accelerators for euROhpc hw/Sw supercomputing applications for exascale: The TEXTAROSSA approach,” Microprocessors and Microsystems, vol. 95, p. 104679, 2022. doi:10.1016/j.micpro.2022.104679
[BibTeX] [Abstract]
In the near future, Exascale systems will need to bridge three technology gaps to achieve high performance while remaining under tight power constraints: energy efficiency and thermal control; extreme computation efficiency via HW acceleration and new arithmetic; methods and tools for seamless integration of reconfigurable accelerators in heterogeneous HPC multi-node platforms. TEXTAROSSA addresses these gaps through a co-design approach to heterogeneous HPC solutions, supported by the integration and extension of HW and SW IPs, programming models, and tools derived from European research.
@article{textarossa2022micpro:, abstract = {In the near future, Exascale systems will need to bridge three technology gaps to achieve high performance while remaining under tight power constraints: energy efficiency and thermal control; extreme computation efficiency via HW acceleration and new arithmetic; methods and tools for seamless integration of reconfigurable accelerators in heterogeneous HPC multi-node platforms. TEXTAROSSA addresses these gaps through a co-design approach to heterogeneous HPC solutions, supported by the integration and extension of HW and SW IPs, programming models, and tools derived from European research.}, author = {Giovanni Agosta and Marco Aldinucci and Carlos Alvarez and Roberto Ammendola and Yasir Arfat and Olivier Beaumont and Massimo Bernaschi and Andrea Biagioni and Tommaso Boccali and Berenger Bramas and Carlo Brandolese and Barbara Cantalupo and Mauro Carrozzo and Daniele Cattaneo and Alessandro Celestini and Massimo Celino and Iacopo Colonnelli and Paolo Cretaro and Pasqua D'Ambra and Marco Danelutto and Roberto Esposito and Lionel Eyraud-Dubois and Antonio Filgueras and William Fornaciari and Ottorino Frezza and Andrea Galimberti and Francesco Giacomini and Brice Goglin and Daniele Gregori and Abdou Guermouche and Francesco Iannone and Michal Kulczewski and Francesca {Lo Cicero} and Alessandro Lonardo and Alberto R. Martinelli and Michele Martinelli and Xavier Martorell and Giuseppe Massari and Simone Montangero and Gianluca Mittone and Raymond Namyst and Ariel Oleksiak and Paolo Palazzari and Pier Stanislao Paolucci and Federico Reghenzani and Cristian Rossi and Sergio Saponara and Francesco Simula and Federico Terraneo and Samuel Thibault and Massimo Torquati and Matteo Turisini and Piero Vicini and Miquel Vidal and Davide Zoni and Giuseppe Zummo}, doi = {10.1016/j.micpro.2022.104679}, issn = {0141-9331}, journal = {Microprocessors and Microsystems}, keywords = {textarossa}, pages = {104679}, title = {Towards EXtreme scale technologies and accelerators for euROhpc hw/Sw supercomputing applications for exascale: The TEXTAROSSA approach}, volume = {95}, year = {2022}, bdsk-url-1 = {https://doi.org/10.1016/j.micpro.2022.104679} }
2021
- G. Agosta, W. Fornaciari, A. Galimberti, G. Massari, F. Reghenzani, F. Terraneo, D. Zoni, C. Brandolese, M. Celino, F. Iannone, P. Palazzari, G. Zummo, M. Bernaschi, P. D’Ambra, S. Saponara, M. Danelutto, M. Torquati, M. Aldinucci, Y. Arfat, B. Cantalupo, I. Colonnelli, R. Esposito, A. R. Martinelli, G. Mittone, O. Beaumont, B. Bramas, L. Eyraud-Dubois, B. Goglin, A. Guermouche, R. Namyst, S. Thibault, A. Filgueras, M. Vidal, C. Alvarez, X. Martorell, A. Oleksiak, M. Kulczewski, A. Lonardo, P. Vicini, F. L. Cicero, F. Simula, A. Biagioni, P. Cretaro, O. Frezza, P. S. Paolucci, M. Turisini, F. Giacomini, T. Boccali, S. Montangero, and R. Ammendola, “TEXTAROSSA: Towards EXtreme scale Technologies and Accelerators for euROhpc hw/Sw Supercomputing Applications for exascale,” in Proc. of the 24th Euromicro Conference on Digital System Design (DSD), Palermo, Italy, 2021. doi:10.1109/DSD53832.2021.00051
[BibTeX] [Abstract]
To achieve high performance and high energy effi- ciency on near-future exascale computing systems, three key technology gaps needs to be bridged. These gaps include: en- ergy efficiency and thermal control; extreme computation effi- ciency via HW acceleration and new arithmetics; methods and tools for seamless integration of reconfigurable accelerators in heterogeneous HPC multi-node platforms. TEXTAROSSA aims at tackling this gap through a co-design approach to heterogeneous HPC solutions, supported by the integration and extension of HW and SW IPs, programming models and tools derived from European research.
@inproceedings{21:DSD:textarossa, abstract = {To achieve high performance and high energy effi- ciency on near-future exascale computing systems, three key technology gaps needs to be bridged. These gaps include: en- ergy efficiency and thermal control; extreme computation effi- ciency via HW acceleration and new arithmetics; methods and tools for seamless integration of reconfigurable accelerators in heterogeneous HPC multi-node platforms. TEXTAROSSA aims at tackling this gap through a co-design approach to heterogeneous HPC solutions, supported by the integration and extension of HW and SW IPs, programming models and tools derived from European research.}, address = {Palermo, Italy}, author = {Giovanni Agosta and William Fornaciari and Andrea Galimberti and Giuseppe Massari and Federico Reghenzani and Federico Terraneo and Davide Zoni and Carlo Brandolese and Massimo Celino and Francesco Iannone and Paolo Palazzari and Giuseppe Zummo and Massimo Bernaschi and Pasqua D'Ambra and Sergio Saponara and Marco Danelutto and Massimo Torquati and Marco Aldinucci and Yasir Arfat and Barbara Cantalupo and Iacopo Colonnelli and Roberto Esposito and Alberto Riccardo Martinelli and Gianluca Mittone and Olivier Beaumont and Berenger Bramas and Lionel Eyraud-Dubois and Brice Goglin and Abdou Guermouche and Raymond Namyst and Samuel Thibault and Antonio Filgueras and Miquel Vidal and Carlos Alvarez and Xavier Martorell and Ariel Oleksiak and Michal Kulczewski and Alessandro Lonardo and Piero Vicini and Francesco Lo Cicero and Francesco Simula and Andrea Biagioni and Paolo Cretaro and Ottorino Frezza and Pier Stanislao Paolucci and Matteo Turisini and Francesco Giacomini and Tommaso Boccali and Simone Montangero and Roberto Ammendola}, booktitle = {Proc. of the 24th Euromicro Conference on Digital System Design ({DSD})}, date-added = {2021-09-04 12:07:42 +0200}, date-modified = {2021-09-04 12:23:41 +0200}, doi = {10.1109/DSD53832.2021.00051}, keywords = {textarossa, streamflow}, month = aug, publisher = {IEEE}, title = {{TEXTAROSSA}: Towards EXtreme scale Technologies and Accelerators for euROhpc hw/Sw Supercomputing Applications for exascale}, year = {2021}, bdsk-url-1 = {https://doi.org/10.1109/DSD53832.2021.00051} }
- Y. Arfat, G. Mittone, R. Esposito, B. Cantalupo, G. M. De Ferrari, and M. Aldinucci, “A Review of Machine Learning for Cardiology,” Minerva cardiology and angiology, 2021. doi:10.23736/s2724-5683.21.05709-4
[BibTeX] [Abstract] [Download PDF]
This paper reviews recent cardiology literature and reports how Artificial Intelligence Tools (specifically, Machine Learning techniques) are being used by physicians in the field. Each technique is introduced with enough details to allow the understanding of how it works and its intent, but without delving into details that do not add immediate benefits and require expertise in the field. We specifically focus on the principal Machine Learning based risk scores used in cardiovascular research. After introducing them and summarizing their assumptions and biases, we discuss their merits and shortcomings. We report on how frequently they are adopted in the field and suggest why this is the case based on our expertise in Machine Learning. We complete the analysis by reviewing how corresponding statistical approaches compare with them. Finally, we discuss the main open issues in applying Machine Learning tools to cardiology tasks, also drafting possible future directions. Despite the growing interest in these tools, we argue that there are many still underutilized techniques: while Neural Networks are slowly being incorporated in cardiovascular research, other important techniques such as Semi-Supervised Learning and Federated Learning are still underutilized. The former would allow practitioners to harness the information contained in large datasets that are only partially labeled, while the latter would foster collaboration between institutions allowing building larger and better models.
@article{21:ai4numbers:minerva, abstract = {This paper reviews recent cardiology literature and reports how Artificial Intelligence Tools (specifically, Machine Learning techniques) are being used by physicians in the field. Each technique is introduced with enough details to allow the understanding of how it works and its intent, but without delving into details that do not add immediate benefits and require expertise in the field. We specifically focus on the principal Machine Learning based risk scores used in cardiovascular research. After introducing them and summarizing their assumptions and biases, we discuss their merits and shortcomings. We report on how frequently they are adopted in the field and suggest why this is the case based on our expertise in Machine Learning. We complete the analysis by reviewing how corresponding statistical approaches compare with them. Finally, we discuss the main open issues in applying Machine Learning tools to cardiology tasks, also drafting possible future directions. Despite the growing interest in these tools, we argue that there are many still underutilized techniques: while Neural Networks are slowly being incorporated in cardiovascular research, other important techniques such as Semi-Supervised Learning and Federated Learning are still underutilized. The former would allow practitioners to harness the information contained in large datasets that are only partially labeled, while the latter would foster collaboration between institutions allowing building larger and better models.}, author = {Yasir Arfat and Gianluca Mittone and Roberto Esposito and Barbara Cantalupo and Gaetano Maria {De Ferrari} and Marco Aldinucci}, date-added = {2021-08-09 23:00:12 +0200}, date-modified = {2021-08-09 23:05:36 +0200}, doi = {10.23736/s2724-5683.21.05709-4}, journal = {Minerva cardiology and angiology}, keywords = {deephealth, hpc4ai}, title = {A Review of Machine Learning for Cardiology}, url = {https://iris.unito.it/retrieve/handle/2318/1796298/780512/21_AI4numbers-preprint.pdf}, year = {2021}, bdsk-url-1 = {https://iris.unito.it/retrieve/handle/2318/1796298/780512/21_AI4numbers-preprint.pdf}, bdsk-url-2 = {https://doi.org/10.23736/s2724-5683.21.05709-4} }
- F. D’Ascenzo, O. De Filippo, G. Gallone, G. Mittone, M. A. Deriu, M. Iannaccone, A. Ariza-Solé, C. Liebetrau, S. Manzano-Fernández, G. Quadri, T. Kinnaird, G. Campo, J. P. Simao Henriques, J. M. Hughes, A. Dominguez-Rodriguez, M. Aldinucci, U. Morbiducci, G. Patti, S. Raposeiras-Roubin, E. Abu-Assi, G. M. De Ferrari, F. Piroli, A. Saglietto, F. Conrotto, P. Omedé, A. Montefusco, M. Pennone, F. Bruno, P. P. Bocchino, G. Boccuzzi, E. Cerrato, F. Varbella, M. Sperti, S. B. Wilton, L. Velicki, I. Xanthopoulou, A. Cequier, A. Iniguez-Romo, I. Munoz Pousa, M. Cespon Fernandez, B. Caneiro Queija, R. Cobas-Paz, A. Lopez-Cuenca, A. Garay, P. F. Blanco, A. Rognoni, G. Biondi Zoccai, S. Biscaglia, I. Nunez-Gil, T. Fujii, A. Durante, X. Song, T. Kawaji, D. Alexopoulos, Z. Huczek, J. R. Gonzalez Juanatey, S. Nie, M. Kawashiri, I. Colonnelli, B. Cantalupo, R. Esposito, S. Leonardi, W. Grosso Marra, A. Chieffo, U. Michelucci, D. Piga, M. Malavolta, S. Gili, M. Mennuni, C. Montalto, L. Oltrona Visconti, and Y. Arfat, “Machine learning-based prediction of adverse events following an acute coronary syndrome (PRAISE): a modelling study of pooled datasets,” The Lancet, vol. 397, iss. 10270, p. 199–207, 2021. doi:10.1016/S0140-6736(20)32519-8
[BibTeX] [Abstract] [Download PDF]
Background The accuracy of current prediction tools for ischaemic and bleeding events after an acute coronary syndrome (ACS) remains insufficient for individualised patient management strategies. We developed a machine learning-based risk stratification model to predict all-cause death, recurrent acute myocardial infarction, and major bleeding after ACS. Methods Different machine learning models for the prediction of 1-year post-discharge all-cause death, myocardial infarction, and major bleeding (defined as Bleeding Academic Research Consortium type 3 or 5) were trained on a cohort of 19826 adult patients with ACS (split into a training cohort [80%] and internal validation cohort [20%]) from the BleeMACS and RENAMI registries, which included patients across several continents. 25 clinical features routinely assessed at discharge were used to inform the models. The best-performing model for each study outcome (the PRAISE score) was tested in an external validation cohort of 3444 patients with ACS pooled from a randomised controlled trial and three prospective registries. Model performance was assessed according to a range of learning metrics including area under the receiver operating characteristic curve (AUC). Findings The PRAISE score showed an AUC of 0.82 (95% CI 0.78-0.85) in the internal validation cohort and 0.92 (0.90-0.93) in the external validation cohort for 1-year all-cause death; an AUC of 0.74 (0.70-0.78) in the internal validation cohort and 0.81 (0.76-0.85) in the external validation cohort for 1-year myocardial infarction; and an AUC of 0.70 (0.66-0.75) in the internal validation cohort and 0.86 (0.82-0.89) in the external validation cohort for 1-year major bleeding. Interpretation A machine learning-based approach for the identification of predictors of events after an ACS is feasible and effective. The PRAISE score showed accurate discriminative capabilities for the prediction of all-cause death, myocardial infarction, and major bleeding, and might be useful to guide clinical decision making.
@article{21:lancet, abstract = {Background The accuracy of current prediction tools for ischaemic and bleeding events after an acute coronary syndrome (ACS) remains insufficient for individualised patient management strategies. We developed a machine learning-based risk stratification model to predict all-cause death, recurrent acute myocardial infarction, and major bleeding after ACS. Methods Different machine learning models for the prediction of 1-year post-discharge all-cause death, myocardial infarction, and major bleeding (defined as Bleeding Academic Research Consortium type 3 or 5) were trained on a cohort of 19826 adult patients with ACS (split into a training cohort [80%] and internal validation cohort [20%]) from the BleeMACS and RENAMI registries, which included patients across several continents. 25 clinical features routinely assessed at discharge were used to inform the models. The best-performing model for each study outcome (the PRAISE score) was tested in an external validation cohort of 3444 patients with ACS pooled from a randomised controlled trial and three prospective registries. Model performance was assessed according to a range of learning metrics including area under the receiver operating characteristic curve (AUC). Findings The PRAISE score showed an AUC of 0.82 (95% CI 0.78-0.85) in the internal validation cohort and 0.92 (0.90-0.93) in the external validation cohort for 1-year all-cause death; an AUC of 0.74 (0.70-0.78) in the internal validation cohort and 0.81 (0.76-0.85) in the external validation cohort for 1-year myocardial infarction; and an AUC of 0.70 (0.66-0.75) in the internal validation cohort and 0.86 (0.82-0.89) in the external validation cohort for 1-year major bleeding. Interpretation A machine learning-based approach for the identification of predictors of events after an ACS is feasible and effective. The PRAISE score showed accurate discriminative capabilities for the prediction of all-cause death, myocardial infarction, and major bleeding, and might be useful to guide clinical decision making.}, author = {Fabrizio D'Ascenzo and Ovidio {De Filippo} and Guglielmo Gallone and Gianluca Mittone and Marco Agostino Deriu and Mario Iannaccone and Albert Ariza-Sol\'e and Christoph Liebetrau and Sergio Manzano-Fern\'andez and Giorgio Quadri and Tim Kinnaird and Gianluca Campo and Jose Paulo {Simao Henriques} and James M Hughes and Alberto Dominguez-Rodriguez and Marco Aldinucci and Umberto Morbiducci and Giuseppe Patti and Sergio Raposeiras-Roubin and Emad Abu-Assi and Gaetano Maria {De Ferrari} and Francesco Piroli and Andrea Saglietto and Federico Conrotto and Pierluigi Omed\'e and Antonio Montefusco and Mauro Pennone and Francesco Bruno and Pier Paolo Bocchino and Giacomo Boccuzzi and Enrico Cerrato and Ferdinando Varbella and Michela Sperti and Stephen B. Wilton and Lazar Velicki and Ioanna Xanthopoulou and Angel Cequier and Andres Iniguez-Romo and Isabel {Munoz Pousa} and Maria {Cespon Fernandez} and Berenice {Caneiro Queija} and Rafael Cobas-Paz and Angel Lopez-Cuenca and Alberto Garay and Pedro Flores Blanco and Andrea Rognoni and Giuseppe {Biondi Zoccai} and Simone Biscaglia and Ivan Nunez-Gil and Toshiharu Fujii and Alessandro Durante and Xiantao Song and Tetsuma Kawaji and Dimitrios Alexopoulos and Zenon Huczek and Jose Ramon {Gonzalez Juanatey} and Shao-Ping Nie and Masa-aki Kawashiri and Iacopo Colonnelli and Barbara Cantalupo and Roberto Esposito and Sergio Leonardi and Walter {Grosso Marra} and Alaide Chieffo and Umberto Michelucci and Dario Piga and Marta Malavolta and Sebastiano Gili and Marco Mennuni and Claudio Montalto and Luigi {Oltrona Visconti} and Yasir Arfat}, date-modified = {2021-03-26 23:53:19 +0100}, doi = {10.1016/S0140-6736(20)32519-8}, issn = {0140-6736}, journal = {The Lancet}, keywords = {deephealth, hpc4ai}, number = {10270}, pages = {199--207}, title = {Machine learning-based prediction of adverse events following an acute coronary syndrome {(PRAISE)}: a modelling study of pooled datasets}, url = {https://www.researchgate.net/profile/James_Hughes3/publication/348501148_Machine_learning-based_prediction_of_adverse_events_following_an_acute_coronary_syndrome_PRAISE_a_modelling_study_of_pooled_datasets/links/6002a81ba6fdccdcb858b6c2/Machine-learning-based-prediction-of-adverse-events-following-an-acute-coronary-syndrome-PRAISE-a-modelling-study-of-pooled-datasets.pdf}, volume = {397}, year = {2021}, bdsk-url-1 = {https://www.researchgate.net/profile/James_Hughes3/publication/348501148_Machine_learning-based_prediction_of_adverse_events_following_an_acute_coronary_syndrome_PRAISE_a_modelling_study_of_pooled_datasets/links/6002a81ba6fdccdcb858b6c2/Machine-learning-based-prediction-of-adverse-events-following-an-acute-coronary-syndrome-PRAISE-a-modelling-study-of-pooled-datasets.pdf}, bdsk-url-2 = {https://doi.org/10.1016/S0140-6736(20)32519-8} }