Samuele Fonio
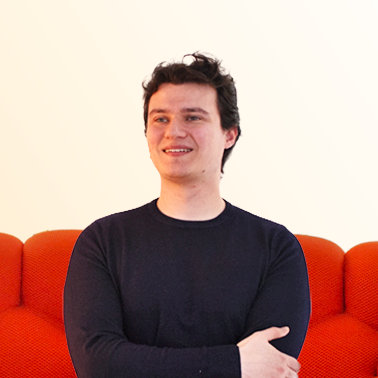
PhD Student
Computer Science Department, University of Turin
Parallel Computing group
Via Pessinetto 12, 10149 Torino – Italy
E-mail: samuele.fonio@unito.it
Samuele Fonio is a PhD student from Modeling and Data Science at UniTo funded by Leonardo Company.
He graduated in Mathematics in 2020 and in Stochastics and Data Science in 2022 with a thesis in Deep Learning comparing the behaviour of different geometries in Image classification task using prototype learning.
Fields of interest:
- Deep Learning
- Geometric Deep Learning
- Federated Learning
Publications
2023
Samuele Fonio, Lorenzo Paletto, Mattia Cerrato, Dino Ienco, Roberto Esposito
Hierarchical priors for Hyperspherical Prototypical Networks Proceedings Article
In: 31th European Symposium on Artificial Neural Networks, Computational Intelligence and Machine Learning, ESANN, Bruges, Belgium, 2023, (In print).
Abstract | Links | BibTeX | Tags: ai, icsc
@inproceedings{23:esann:fonio,
title = {Hierarchical priors for Hyperspherical Prototypical Networks},
author = {Samuele Fonio and Lorenzo Paletto and Mattia Cerrato and Dino Ienco and Roberto Esposito},
url = {https://www.esann.org/sites/default/files/proceedings/2023/ES2023-65.pdf},
year = {2023},
date = {2023-10-01},
booktitle = {31th European Symposium on Artificial Neural Networks, Computational Intelligence and Machine Learning, ESANN},
address = {Bruges, Belgium},
abstract = {In this paper, we explore the usage of hierarchical priors to improve learning in contexts where the number of available examples is extremely low. Specifically, we consider a Prototype Learning setting where deep neural networks are used to embed data in hyperspherical geometries.In this scenario, we propose an innovative way to learn the prototypes by combining class separation and hierarchical information. In addition, we introduce a contrastive loss function capable of balancing the exploitation of prototypes through a prototype pruning mechanism. We compare the proposed method with state-of-the-art approaches on two public datasets.},
note = {In print},
keywords = {ai, icsc},
pubstate = {published},
tppubtype = {inproceedings}
}
Samuele Fonio
Benchmarking Federated Learning Frameworks for Medical Imaging Tasks Proceedings Article
In: Image Analysis and Processing - ICIAP 2023 - 22th International Conference - FedMed, Springer LNCS, Udine, Italy, 2023, (In print).
Abstract | Links | BibTeX | Tags: ai, confidential, eupilot, icsc
@inproceedings{23:iciap:fedmed:ws:fonio,
title = {Benchmarking Federated Learning Frameworks for Medical Imaging Tasks},
author = {Samuele Fonio},
url = {https://iris.unito.it/retrieve/c6be8be7-3980-4c4c-874e-68b6fd855ebc/FedMed23-3.pdf},
year = {2023},
date = {2023-09-01},
booktitle = {Image Analysis and Processing - ICIAP 2023 - 22th International Conference - FedMed},
publisher = {Springer LNCS},
address = {Udine, Italy},
abstract = {This paper presents a comprehensive benchmarking study of various Federated Learning (FL) frameworks applied to the task of Medical Image Classification. The research specifically addresses the often neglected and complex aspects of scalability and usability in off-the-shelf FL frameworks. Through experimental validation using real case deployments, we provide empirical evidence of the performance and practical relevance of open source FL frameworks. Our findings contribute valuable insights for anyone interested in deploying a FL system, with a particular focus on the healthcare domain—an increasingly attractive field for FL applications.},
note = {In print},
keywords = {ai, confidential, eupilot, icsc},
pubstate = {published},
tppubtype = {inproceedings}
}
Gianluca Mittone, Samuele Fonio
Benchmarking Federated Learning Scalability Proceedings Article
In: Proceedings of the 2nd Italian Conference on Big Data and Data Science, ITADATA 2023, September 11-13, 2023, CEUR, Naples, Italy, 2023.
Abstract | Links | BibTeX | Tags: confidential, eupilot, HPC, icsc
@inproceedings{23:itadata:extabstract:mittone:fonio,
title = {Benchmarking Federated Learning Scalability},
author = {Gianluca Mittone and Samuele Fonio},
url = {https://hdl.handle.net/2318/1933852},
year = {2023},
date = {2023-09-01},
booktitle = {Proceedings of the 2nd Italian Conference on Big Data and Data Science, ITADATA 2023, September 11-13, 2023},
publisher = {CEUR},
address = {Naples, Italy},
abstract = {Federated Learning (FL) is a widespread Machine Learning paradigm handling distributed Big Data. In this work, we demonstrate that different FL frameworks expose different scaling performances despite adopting the same technologies, highlighting the need for a more comprehensive study on the topic.},
keywords = {confidential, eupilot, HPC, icsc},
pubstate = {published},
tppubtype = {inproceedings}
}
Talks
2023
Samuele Fonio
Benchmarking Federated Learning Frameworks for Medical Imaging Tasks Miscellaneous
Image Analysis and Processing - ICIAP 2023 - 22th International Conference - FedMed, 2023.
Abstract | Links | BibTeX | Tags: eupilot, icsc
@misc{23:iciap:benchmed,
title = {Benchmarking Federated Learning Frameworks for Medical Imaging Tasks},
author = {Samuele Fonio},
url = {https://datacloud.di.unito.it/index.php/s/sR7YeTGgfH4DtCR},
year = {2023},
date = {2023-09-01},
address = {Udine, Italy},
abstract = {This paper presents a comprehensive benchmarking study of various Federated Learning (FL) frameworks applied to the task of Medical Image Classification. The research specifically addresses the often neglected and complex aspects of scalability and usability in off-the-shelf FL frameworks. Through experimental validation using real case deployments, we provide empirical evidence of the performance and practical relevance of open source FL frameworks. Our findings contribute valuable insights for anyone interested in deploying a FL system, with a particular focus on the healthcare domain—an increasingly attractive field for FL applications.},
howpublished = {Image Analysis and Processing - ICIAP 2023 - 22th International Conference - FedMed},
keywords = {eupilot, icsc},
pubstate = {published},
tppubtype = {misc}
}
Gianluca Mittone, Samuele Fonio
Benchmarking Federated Learning Scalability Miscellaneous
2nd Italian Conference on Big Data and Data Science (ITADATA 2023), 2023.
Abstract | Links | BibTeX | Tags: eupilot, icsc
@misc{23:itadata:fl_scaling,
title = {Benchmarking Federated Learning Scalability},
author = {Gianluca Mittone and Samuele Fonio},
url = {https://datacloud.di.unito.it/index.php/s/QZGxC4X3s5LG5oT},
year = {2023},
date = {2023-09-01},
address = {Naples, Italy},
abstract = {Federated Learning (FL) is a widespread Machine Learning paradigm handling distributed Big Data. In this work, we demonstrate that different FL frameworks expose different scaling performances despite adopting the same technologies, highlighting the need for a more comprehensive study on the topic.},
howpublished = {2nd Italian Conference on Big Data and Data Science (ITADATA 2023)},
keywords = {eupilot, icsc},
pubstate = {published},
tppubtype = {misc}
}
Bruno Casella, Samuele Fonio
Architecture-Based FedAvg for Vertical Federated Learning Miscellaneous
2023, (https://iris.unito.it/bitstream/2318/1949730/1/HALF_HVL_for_DML_ICC23___Taormina-2.pdf).
Abstract | Links | BibTeX | Tags: ai, epi, fl, icsc
@misc{23:casella:architecturalfedavg,
title = {Architecture-Based FedAvg for Vertical Federated Learning},
author = {Bruno Casella and Samuele Fonio},
url = {https://datacloud.di.unito.it/index.php/s/kJQxnqG4d2ZSicK},
doi = {10.1109/ICCVW60793.2023.00362},
year = {2023},
date = {2023-01-01},
booktitle = {Proceedings of the 3rd Workshop on Distributed Machine Learning for the Intelligent Computing Continuum (DML-ICC), IEEE/ACM UCC 2023, Taormina, Italy, 4 December 2023},
abstract = {Federated Learning (FL) has emerged as a promising solution to address privacy concerns by collaboratively training Deep Learning (DL) models across distributed parties. This work proposes an architecture-based aggregation strategy in Vertical FL, where parties hold data with different attributes but shared instances. Our approach leverages the identical architectural parts, i.e. neural network layers, of different models to selectively aggregate weights, which is particularly relevant when collaborating with institutions holding different types of datasets, i.e., image, text, or tabular datasets. In a scenario where two entities train DL models, such as a Convolutional Neural Network (CNN) and a Multi-Layer Perceptron (MLP), our strategy computes the average only for architecturally identical segments. This preserves data-specific features learned from demographic and clinical data. We tested our approach on two clinical datasets, i.e., the COVID-CXR dataset and the ADNI study. Results show that our method achieves comparable results with the centralized scenario, in which all the data are collected in a single data lake, and benefits from FL generalizability. In particular, compared to the non-federated models, our proposed proof-of-concept model exhibits a slight performance loss on the COVID-CXR dataset (less than 8%), but outperforms ADNI models by up to 12%. Moreover, communication costs between training rounds are minimized by exchanging only the dense layer parameters.},
note = {https://iris.unito.it/bitstream/2318/1949730/1/HALF_HVL_for_DML_ICC23___Taormina-2.pdf},
keywords = {ai, epi, fl, icsc},
pubstate = {published},
tppubtype = {misc}
}