Iacopo Colonnelli
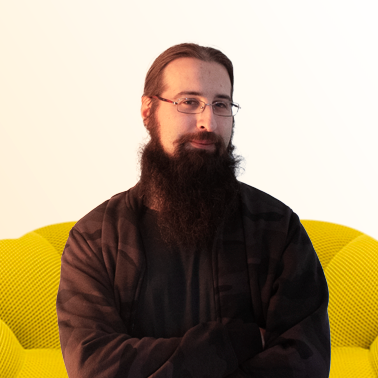
Assistant Professor (RTDA)
Alpha Research Group (Parallel Computing)
University of Turin, Computer Science Dept.
Via Pessinetto 12, 10149 Torino – Italy
Email: iacopo.colonnelli@unito.it
ORCID: 0000-0001-9290-2017
Scopus Author ID: 57208128139
Researcher ID: AAK-3784-2021
Google Scholar ID: yFutTsMAAAAJ
Short Bio
Iacopo Colonnelli is a Computer Science assistant professor (RTDA) and a member of the CINI HPC Key Technologies and Tools lab. He received his Ph.D. with honours in Modeling and Data Science at Università di Torino with a thesis on novel workflow models for heterogeneous distributed systems, and his master’s degree in Computer Engineering from Politecnico di Torino with a thesis on a high-performance parallel tracking algorithm for the ALICE experiment at CERN.
His research focuses on both statistical and computational aspects of data analysis at large scale and on workflow modeling and management in heterogeneous distributed architectures. He is a member of the CWL Technical Team.
Open Source Software
- Creator and maintainer of StreamFlow, a container-native workflow management system which supports hybrid workflows and their executions on cloud-HPC infrastructures.
- Creator and maintainer of Jupyter Workflow, an extension of the IPython kernel designed to support distributed literate workflows and to execute them in a distributed fashion on cloud-HPC architectures.
Research Projects
- Space Center of Excellence (EC HE, HORIZON-EUROHPC-JU-2021-COE-01): Scalable Parallel and distributed Astrophysical Codes for Exascale (2023, 48 months, total cost 8M€, G.A. n. 101093441).
- European Pilot (EC H2020 RIA, EuroHPC-02-2020, 42 months, total cost 30M€, G.A. n. 101034126).
- EUPEX (EC H2020 RIA, EuroHPC-02-2020): European Pilot for Exascale (2021, 48 months, total cost 41M€, G.A. n. 101033975).
Read More
- TEXTAROSSA (EC H2020 RIA, EuroHPC-01-2019): Towards EXtreme scale Technologies and Accelerators for euROhpc hw/Sw Supercomputing Applications for exascale (2021, 36 months, total cost 6M€, G.A. n. 956831).
- ACROSS (EC H2020 IA, EuroHPC-01-2019): HPC Big Data Artificial Intelligence cross-stack platform toward exascale (2021, 36 months, total cost 8M€, G.A. n. 955648).
- DeepHealth (EC H2020 IA, ICT-2018-11): Deep-Learning and HPC to Boost Biomedical Applications for Health (2019, 36 months, total cost 14.8M€, G.A. 825111).
- HPC4AI (Regione Piemonte, POR FESR Regione Piemonte): Turin’s centre in High-Performance Computing for Artificial Intelligence (2018, 24 months, total cost 4.5M€).
Achievements
- [2023] The Ph.D. thesis “Workflow models for heterogeneous distributed systems” has been awarded the ITADATA 2023 Best PhD Thesis Award.
- [2023] StreamFlow has been selected as one of the three exploitable results of the EUPEX European project
- [2023] StreamFlow has been selected as an exploring technology by the EC Innovation Radar initiative.
Read More
- [2022] The article “Distributed Workflows With Jupyter” has been selected by the Future Generation Computer Systems journal as Fall 2022 Editor’s Choice.
- [2019] Selected as HPC-Europa3 grant recipient for a 13 weeks visit to Barcelona Supercomputing Center, Spain (then reduced to 56 days due to COVID-19 pandemic).
- [2019] Selected as HiPEAC grant recipient for attending the ACACES 2019 Summer School in Fiuggi, Italy.
Standardization Committee Memberships
- Member of the CWL Technical Team, responsible for approving new versions of the CWL standards before that proposal goes to the CWL Leadership Team for the final vote.
- Founder of the CWL4HPC Working Group, which aims to identify patterns capable of modeling large-scale scientific applications and implementing the related CWL enhancement proposals.
Service as Chairperson
- HiPES 2024 (1st Workshop about High-Performance eScience), Madrid, Spain, 2024. Role: organizer.
- WiDE 2024 (2nd International Workshop on Workflows in Distributed Environments), Athens, Greece, 2024. Role: organizer and session chair.
Read More
- ITADATA 2023 (2nd Italian Conference on Big Data and Data Science), Napoli, Italy, 2023. Role: session chair.
- WiDE 2023 (1st International Workshop on Workflows in Distributed Environments), Torino, Italy, 2023. Role: organizer and session chair.
- COMPSAC 2023 (47th Annual Computers, Software, and Applications Conference), Torino, Italy, 2023. Role: local organizer.
Program Committee Memberships
- SC24 (International Conference for High Performance Computing, Networking, Storage, and Analysis), Atlanta, GA, USA, 2024.
- FRAME 2024 (4th Workshop on Flexible Resource and Application Management on the Edge), Pisa, Italy, 2024.
- IWAHPCE 2024 (International Workshop on Arm-based HPC: Practice & Experience), Nagoya, Japan, 2024.
Read More
- SBAC-PAD 2023 (35th International Symposium on Computer Architecture and High Performance Computing), Porto Alegre, Brazil, 2023.
- PAW-ATM 2023 (6th Annual Parallel Applications Workshop, Alternatives To MPI+X), Denver, Colorado, USA, 2023.
- HLPP 2023 (16th International Symposium on High-level Parallel Programming and Applications), Cluj-Napoca, Romania, 2023.
- HPCMALL 2023 (2nd International Workshop on Malleability Techniques Applications in High-Performance Computingg), Hamburg, Germany, 2023.
- EURO-PAR 2023 (29th International European Conference on Parallel and Distributed Computing), Limassol, Cyprus, 2023.
- PDP 2023 (31st Euromicro International Conference on Parallel, Distributed, and Network-Based Processing), Napoli, Italy, 2023.
- HLPP 2022 (15th International Symposium on High-level Parallel Programming and Applications), Porto, Portugal, 2022.
- EURO-PAR 2022 (28th International European Conference on Parallel and Distributed Computing), Glasgow, Scotland, United Kingdom, 2022.
- HPCMALL 2022 (Malleability Techniques Applications in High-Performance Computing), Hamburg, Germany, 2022.
- IPTA 2022 (11th International Conference on Image Processing Theory, Tools and Applications), Salzburg, Austria, 2022.
- EURO-PAR 2021 (27th International European Conference on Parallel and Distributed Computing), Lisbon, Portugal, 2021.
- HiPC 2020 (27th IEEE International Conference on High Performance Computing, Data, and Analytics), Pune, India, 2020.
- IPTA 2019 (9th International Conference on Image Processing Theory, Tools and Applications), Istanbul, Turkey, 2019.
Publications
2024
Bruno Casella, Iacopo Colonnelli, Gianluca Mittone, Robert Birke, Walter Riviera, Antonio Sciarappa, Carlo Cavazzoni, Marco Aldinucci
A Performance Model for Confidential Federated Learning Proceedings Article
In: Proceedings of the 2024 Deep Learning Security and Privacy Workshop, IEEE Symposium on Security and Privacy 2024, San Francisco, CA, 2024.
Abstract | Links | BibTeX | Tags: confidential, epi, icsc
@inproceedings{24:casella:sgx,
title = {A Performance Model for Confidential Federated Learning},
author = {Bruno Casella and Iacopo Colonnelli and Gianluca Mittone and Robert Birke and Walter Riviera and Antonio Sciarappa and Carlo Cavazzoni and Marco Aldinucci},
url = {https://iris.unito.it/retrieve/b5877a97-2d8d-4e95-8791-0aa4a1b953b3/DLSP___CONFIDENTIAL_FL.pdf},
year = {2024},
date = {2024-05-01},
booktitle = {Proceedings of the 2024 Deep Learning Security and Privacy Workshop, IEEE Symposium on Security and Privacy 2024},
address = {San Francisco, CA},
abstract = {Federated Learning (FL) has emerged as a solution to preserve data privacy by keeping the data locally on each participant's device. However, FL alone is still vulnerable to attacks that can cause privacy leaks. Therefore, it becomes necessary to take additional security measures at the cost of increasing runtimes. The Trusted Execution Environment (TEE) approach promises to offer the highest degree of security during execution. However, TEEs suffer from memory limits which prevent safe end-to-end FL training of modern deep models. State-of- the-art approaches limit secure training to selected layers, failing to avert the full spectrum of attacks or adopt layer-wise training affecting model performance. We benchmark the usage of a library OS (LibOS) to run the full, unmodified end-to-end FL training inside the TEE. We extensively evaluate and model the overhead of the different security mechanisms needed to protect the data and model during computation (TEE), communication (TLS), and storage (disk encryption). The obtained results across three datasets and two models demonstrate that LibOSes are a viable way to seamlessly inject security into FL with limited overhead (at most 2x), offering valuable guidance for researchers and developers aiming to apply FL in data-security-focused contexts.},
keywords = {confidential, epi, icsc},
pubstate = {published},
tppubtype = {inproceedings}
}
2023
Alberto Riccardo Martinelli, Massimo Torquati, Marco Aldinucci, Iacopo Colonnelli, Barbara Cantalupo
CAPIO: a Middleware for Transparent I/O Streaming in Data-Intensive Workflows Proceedings Article
In: 2023 IEEE 30th International Conference on High Performance Computing, Data, and Analytics (HiPC), IEEE, Goa, India, 2023.
Abstract | Links | BibTeX | Tags: admire, capio, eupex, icsc
@inproceedings{23:hipc:capio,
title = {CAPIO: a Middleware for Transparent I/O Streaming in Data-Intensive Workflows},
author = {Alberto Riccardo Martinelli and Massimo Torquati and Marco Aldinucci and Iacopo Colonnelli and Barbara Cantalupo},
url = {https://iris.unito.it/retrieve/27380f37-0978-409e-a9d8-2b5e95a4bb85/CAPIO-HiPC23-preprint.pdf},
doi = {10.1109/HiPC58850.2023.00031},
year = {2023},
date = {2023-12-01},
booktitle = {2023 IEEE 30th International Conference on High Performance Computing, Data, and Analytics (HiPC)},
publisher = {IEEE},
address = {Goa, India},
abstract = {With the increasing amount of digital data available for analysis and simulation, the class of I/O-intensive HPC workflows is fated to quickly expand, further exacerbating the performance gap between computing, memory, and storage technologies. This paper introduces CAPIO (Cross-Application Programmable I/O), a middleware capable of injecting I/O streaming capabilities into file-based workflows, improving the computation-I/O overlap without the need to change the application code. The contribution is twofold: 1) at design time, a new I/O coordination language allows users to annotate workflow data dependencies with synchronization semantics; 2) at run time, a user-space middleware automatically and transparently to the user turns a workflow batch execution into a streaming execution according to the semantics expressed in the configuration file. CAPIO has been tested on synthetic benchmarks simulating typical workflow I/O patterns and two real-world workflows. Experiments show that CAPIO reduces the execution time by 10% to 66% for data-intensive workflows that use the file system as a communication medium.},
keywords = {admire, capio, eupex, icsc},
pubstate = {published},
tppubtype = {inproceedings}
}
Marco Aldinucci, Elena Maria Baralis, Valeria Cardellini, Iacopo Colonnelli, Marco Danelutto, Sergio Decherchi, Giuseppe Di Modica, Luca Ferrucci, Marco Gribaudo, Francesco Iannone, Marco Lapegna, Doriana Medic, Giuseppa Muscianisi, Francesca Righetti, Eva Sciacca, Nicola Tonellotto, Mauro Tortonesi, Paolo Trunfio, Tullio Vardanega
A Systematic Mapping Study of Italian Research on Workflows Proceedings Article
In: Proceedings of the SC '23 Workshops of The International Conference on High Performance Computing, Network, Storage, and Analysis, SC-W 2023, pp. 2065–2076, ACM, Denver, CO, USA, 2023.
Abstract | Links | BibTeX | Tags: icsc, jupyter-workflow, streamflow
@inproceedings{WORKS2023,
title = {A Systematic Mapping Study of Italian Research on Workflows},
author = {Marco Aldinucci and Elena Maria Baralis and Valeria Cardellini and Iacopo Colonnelli and Marco Danelutto and Sergio Decherchi and Giuseppe Di Modica and Luca Ferrucci and Marco Gribaudo and Francesco Iannone and Marco Lapegna and Doriana Medic and Giuseppa Muscianisi and Francesca Righetti and Eva Sciacca and Nicola Tonellotto and Mauro Tortonesi and Paolo Trunfio and Tullio Vardanega},
url = {https://doi.org/10.1145/3624062.3624285},
doi = {10.1145/3624062.3624285},
year = {2023},
date = {2023-11-01},
booktitle = {Proceedings of the SC '23 Workshops of The International Conference on High Performance Computing, Network, Storage, and Analysis, SC-W 2023},
pages = {2065–2076},
publisher = {ACM},
address = {Denver, CO, USA},
abstract = {An entire ecosystem of methodologies and tools revolves around scientific workflow management. They cover crucial non-functional requirements that standard workflow models fail to target, such as interactive execution, energy efficiency, performance portability, Big Data management, and intelligent orchestration in the Computing Continuum. Characterizing and monitoring this ecosystem is crucial to develop an informed view of current and future research directions. This work conducts a systematic mapping study of the Italian workflow research community, collecting and analyzing 25 tools and 10 applications from several scientific domains in the context of the ``National Research Centre for HPC, Big Data, and Quantum Computing'' (ICSC). The study aims to outline the main current research directions and determine how they address the critical needs of modern scientific applications. The findings highlight a variegated research ecosystem of tools, with a prominent interest in advanced workflow orchestration and still immature but promising efforts toward energy efficiency.},
keywords = {icsc, jupyter-workflow, streamflow},
pubstate = {published},
tppubtype = {inproceedings}
}
Gianluca Mittone, Walter Riviera, Iacopo Colonnelli, Robert Birke, Marco Aldinucci
Model-Agnostic Federated Learning Proceedings Article
In: Euro-Par 2023: Parallel Processing, pp. 383–396, Springer, Limassol, Cyprus, 2023.
Abstract | Links | BibTeX | Tags: confidential, eupilot, icsc, riscv
@inproceedings{23:mittone:mafl,
title = {Model-Agnostic Federated Learning},
author = {Gianluca Mittone and Walter Riviera and Iacopo Colonnelli and Robert Birke and Marco Aldinucci},
url = {https://doi.org/10.1007/978-3-031-39698-4_26},
doi = {10.1007/978-3-031-39698-4_26},
year = {2023},
date = {2023-08-01},
booktitle = {Euro-Par 2023: Parallel Processing},
volume = {14100},
pages = {383–396},
publisher = {Springer},
address = {Limassol, Cyprus},
institution = {Computer Science Department, University of Torino},
abstract = {Since its debut in 2016, Federated Learning (FL) has been tied to the inner workings of Deep Neural Networks (DNNs). On the one hand, this allowed its development and widespread use as DNNs proliferated. On the other hand, it neglected all those scenarios in which using DNNs is not possible or advantageous. The fact that most current FL frameworks only allow training DNNs reinforces this problem. To address the lack of FL solutions for non-DNN-based use cases, we propose MAFL (Model-Agnostic Federated Learning). MAFL marries a model-agnostic FL algorithm, AdaBoost.F, with an open industry-grade FL framework: Intel OpenFL. MAFL is the first FL system not tied to any specific type of machine learning model, allowing exploration of FL scenarios beyond DNNs and trees. We test MAFL from multiple points of view, assessing its correctness, flexibility and scaling properties up to 64 nodes. We optimised the base software achieving a 5.5x speedup on a standard FL scenario. MAFL is compatible with x86-64, ARM-v8, Power and RISC-V.},
keywords = {confidential, eupilot, icsc, riscv},
pubstate = {published},
tppubtype = {inproceedings}
}
Iacopo Colonnelli, Robert Birke, Marco Aldinucci
Experimenting with PyTorch on RISC-V Proceedings Article
In: RISC-V Summit Europe 2023, Barcelona, Spain, 2023, (Poster).
Abstract | Links | BibTeX | Tags: eupilot, icsc, riscv
@inproceedings{23:risc-v-summit,
title = {Experimenting with PyTorch on RISC-V},
author = {Iacopo Colonnelli and Robert Birke and Marco Aldinucci},
url = {https://iris.unito.it/retrieve/429bf344-9090-42c3-809c-1b8ac320a930/2023-06-08-Iacopo-COLONNELLI-abstract.pdf},
year = {2023},
date = {2023-06-01},
booktitle = {RISC-V Summit Europe 2023},
address = {Barcelona, Spain},
abstract = {RISC-V is an emerging instruction set architecture. Its modular and extensible open-source royalty-free design is increasingly attracting interest from both research and industry. Nowadays, different RISC-V-based boards can be bought off the shelf. However, software availability is equivalently vital in guaranteeing the RISC-V ecosystem's success. Here we contribute with the first publicly available port of PyTorch. PyTorch is one of the most popular Deep Learning libraries available today. As such, it is a crucial enabler in running state-of-the-art AI applications on RISC-V-based systems and a first step towards a fully democratic end-to-end codesign process.},
note = {Poster},
keywords = {eupilot, icsc, riscv},
pubstate = {published},
tppubtype = {inproceedings}
}
Gianluca Mittone, Nicolò Tonci, Robert Birke, Iacopo Colonnelli, Doriana Medić, Andrea Bartolini, Roberto Esposito, Emanuele Parisi, Francesco Beneventi, Mirko Polato, Massimo Torquati, Luca Benini, Marco Aldinucci
Experimenting with Emerging RISC-V Systems for Decentralised Machine Learning Proceedings Article
In: 20th ACM International Conference on Computing Frontiers (CF '23), ACM, Bologna, Italy, 2023, ISBN: 979-8-4007-0140-5/23/05, (https://arxiv.org/abs/2302.07946).
Abstract | Links | BibTeX | Tags: confidential, eupilot, HPC, icsc, riscv
@inproceedings{23:mittone:fl-riscv,
title = {Experimenting with Emerging RISC-V Systems for Decentralised Machine Learning},
author = {Gianluca Mittone and Nicolò Tonci and Robert Birke and Iacopo Colonnelli and Doriana Medić and Andrea Bartolini and Roberto Esposito and Emanuele Parisi and Francesco Beneventi and Mirko Polato and Massimo Torquati and Luca Benini and Marco Aldinucci},
url = {https://dl.acm.org/doi/pdf/10.1145/3587135.3592211},
doi = {10.1145/3587135.3592211},
isbn = {979-8-4007-0140-5/23/05},
year = {2023},
date = {2023-05-01},
booktitle = {20th ACM International Conference on Computing Frontiers (CF '23)},
publisher = {ACM},
address = {Bologna, Italy},
institution = {Computer Science Department, University of Torino},
abstract = {Decentralised Machine Learning (DML) enables collaborative machine learning without centralised input data. Federated Learning (FL) and Edge Inference are examples of DML. While tools for DML (especially FL) are starting to flourish, many are not flexible and portable enough to experiment with novel systems (e.g., RISC-V), non-fully connected topologies, and asynchronous collaboration schemes. We overcome these limitations via a domain-specific language allowing to map DML schemes to an underlying middleware, i.e. the FastFlow parallel programming library. We experiment with it by generating different working DML schemes on two emerging architectures (ARM-v8, RISC-V) and the x86-64 platform. We characterise the performance and energy efficiency of the presented schemes and systems. As a byproduct, we introduce a RISC-V porting of the PyTorch framework, the first publicly available to our knowledge.},
note = {https://arxiv.org/abs/2302.07946},
keywords = {confidential, eupilot, HPC, icsc, riscv},
pubstate = {published},
tppubtype = {inproceedings}
}
Iacopo Colonnelli
Workflow Models for Heterogeneous Distributed Systems Proceedings Article
In: Bena, Nicola, Martino, Beniamino Di, Maratea, Antonio, Sperduti, Alessandro, Nardo, Emanuel Di, Ciaramella, Angelo, Montella, Raffaele, Ardagna, Claudio A. (Ed.): Proceedings of the 2nd Italian Conference on Big Data and Data Science (ITADATA 2023), Naples, Italy, September 11-13, 2023, CEUR-WS.org, 2023.
Abstract | Links | BibTeX | Tags: across, eupex, icsc, jupyter-workflow, streamflow
@inproceedings{23:colonnelli:itadata,
title = {Workflow Models for Heterogeneous Distributed Systems},
author = {Iacopo Colonnelli},
editor = {Nicola Bena and Beniamino Di Martino and Antonio Maratea and Alessandro Sperduti and Emanuel Di Nardo and Angelo Ciaramella and Raffaele Montella and Claudio A. Ardagna},
url = {https://ceur-ws.org/Vol-3606/invited77.pdf},
year = {2023},
date = {2023-01-01},
booktitle = {Proceedings of the 2nd Italian Conference on Big Data and Data Science (ITADATA 2023), Naples, Italy, September 11-13, 2023},
volume = {3606},
publisher = {CEUR-WS.org},
series = {CEUR Workshop Proceedings},
abstract = {This article introduces a novel hybrid workflow abstraction that injects topology awareness directly into the definition of a distributed workflow model. In particular, the article briefly discusses the advantages brought by this approach to the design and orchestration of large-scale data-oriented workflows, the current level of support from state-of-the-art workflow systems, and some future research directions.},
keywords = {across, eupex, icsc, jupyter-workflow, streamflow},
pubstate = {published},
tppubtype = {inproceedings}
}
William Fornaciari, Federico Reghenzani, Federico Terraneo, Davide Baroffio, Cecilia Metra, Martin Omana, Josie E. Rodriguez Condia, Matteo Sonza Reorda, Robert Birke, Iacopo Colonnelli, Gianluca Mittone, Marco Aldinucci, Gabriele Mencagli, Francesco Iannone, Filippo Palombi, Giuseppe Zummo, Daniele Cesarini, Federico Tesser
RISC-V-based Platforms for HPC: Analyzing Non-functional Properties for Future HPC and Big-Data Clusters Proceedings Article
In: Embedded Computer Systems: Architectures, Modeling, and Simulation - 23rd International Conference, SAMOS 2023, Samos, Greece, 2023, (icsc).
Abstract | Links | BibTeX | Tags: icsc, riscv
@inproceedings{23:SAMOS,
title = {RISC-V-based Platforms for HPC: Analyzing Non-functional Properties for Future HPC and Big-Data Clusters},
author = {William Fornaciari and Federico Reghenzani and Federico Terraneo and Davide Baroffio and Cecilia Metra and Martin Omana and Josie E. Rodriguez Condia and Matteo Sonza Reorda and Robert Birke and Iacopo Colonnelli and Gianluca Mittone and Marco Aldinucci and Gabriele Mencagli and Francesco Iannone and Filippo Palombi and Giuseppe Zummo and Daniele Cesarini and Federico Tesser},
url = {https://iris.unito.it/retrieve/b627eab0-3aa1-4fd7-8685-f47c62c792b3/SAMOS_2023_CN_HPC_FL1.pdf},
doi = {10.1007/978-3-031-46077-7_26},
year = {2023},
date = {2023-01-01},
booktitle = {Embedded Computer Systems: Architectures, Modeling, and Simulation - 23rd International Conference, SAMOS 2023},
address = {Samos, Greece},
abstract = {High-PerformanceComputing(HPC)haveevolvedtobeused to perform simulations of systems where physical experimentation is pro- hibitively impractical, expensive, or dangerous. This paper provides a general overview and showcases the analysis of non-functional properties in RISC-V-based platforms for HPCs. In particular, our analyses target the evaluation of power and energy control, thermal management, and reliability assessment of promising systems, structures, and technologies devised for current and future generation of HPC machines. The main set of design methodologies and technologies developed within the activ- ities of the Future and HPC & Big Data spoke of the National Centre of HPC, Big Data and Quantum Computing project are described along with the description of the testbed for experimenting two-phase cooling approaches.},
note = {icsc},
keywords = {icsc, riscv},
pubstate = {published},
tppubtype = {inproceedings}
}
Yasir Arfat, Gianluca Mittone, Iacopo Colonnelli, Fabrizio D'Ascenzo, Roberto Esposito, Marco Aldinucci
Pooling critical datasets with Federated Learning Proceedings Article
In: 31st Euromicro International Conference on Parallel, Distributed and Network-Based Processing, PDP 2023, pp. 329–337, IEEE, Napoli, Italy, 2023.
Abstract | Links | BibTeX | Tags: admire, confidential, hpc4ai
@inproceedings{23:praise-fl:pdp,
title = {Pooling critical datasets with Federated Learning},
author = {Yasir Arfat and Gianluca Mittone and Iacopo Colonnelli and Fabrizio D'Ascenzo and Roberto Esposito and Marco Aldinucci},
url = {https://iris.unito.it/retrieve/491e22ec-3db5-4989-a063-085a199edd20/23_pdp_fl.pdf},
doi = {10.1109/PDP59025.2023.00057},
year = {2023},
date = {2023-01-01},
booktitle = {31st Euromicro International Conference on Parallel, Distributed and Network-Based Processing, PDP 2023},
pages = {329–337},
publisher = {IEEE},
address = {Napoli, Italy},
abstract = {Federated Learning (FL) is becoming popular in different industrial sectors where data access is critical for security, privacy and the economic value of data itself. Unlike traditional machine learning, where all the data must be globally gathered for analysis, FL makes it possible to extract knowledge from data distributed across different organizations that can be coupled with different Machine Learning paradigms. In this work, we replicate, using Federated Learning, the analysis of a pooled dataset (with AdaBoost) that has been used to define the PRAISE score, which is today among the most accurate scores to evaluate the risk of a second acute myocardial infarction. We show that thanks to the extended-OpenFL framework, which implements AdaBoost.F, we can train a federated PRAISE model that exhibits comparable accuracy and recall as the centralised model. We achieved F1 and F2 scores which are consistently comparable to the PRAISE score study of a 16- parties federation but within an order of magnitude less time.},
keywords = {admire, confidential, hpc4ai},
pubstate = {published},
tppubtype = {inproceedings}
}
Sandro Gepiro Contaldo, Luca Alessandri, Iacopo Colonnelli, Marco Beccuti, Marco Aldinucci
Bringing Cell Subpopulation Discovery on a Cloud-HPC Using rCASC and StreamFlow Book Chapter
In: Calogero, Raffaele Adolfo, Benes, Vladimir (Ed.): Single Cell Transcriptomics: Methods and Protocols, pp. 337–345, Springer US, New York, NY, 2023, ISBN: 978-1-0716-2756-3.
Abstract | Links | BibTeX | Tags: streamflow
@inbook{Contaldo2023,
title = {Bringing Cell Subpopulation Discovery on a Cloud-HPC Using rCASC and StreamFlow},
author = {Sandro Gepiro Contaldo and Luca Alessandri and Iacopo Colonnelli and Marco Beccuti and Marco Aldinucci},
editor = {Raffaele Adolfo Calogero and Vladimir Benes},
url = {https://datacloud.di.unito.it/index.php/s/KMfKo4m7GTGdZmF},
doi = {10.1007/978-1-0716-2756-3_17},
isbn = {978-1-0716-2756-3},
year = {2023},
date = {2023-01-01},
booktitle = {Single Cell Transcriptomics: Methods and Protocols},
pages = {337–345},
publisher = {Springer US},
address = {New York, NY},
abstract = {The idea behind novel single-cell RNA sequencing (scRNA-seq) pipelines is to isolate single cells through microfluidic approaches and generate sequencing libraries in which the transcripts are tagged to track their cell of origin. Modern scRNA-seq platforms are capable of analyzing up to many thousands of cells in each run. Then, combined with massive high-throughput sequencing producing billions of reads, scRNA-seq allows the assessment of fundamental biological properties of cell populations and biological systems at unprecedented resolution.},
keywords = {streamflow},
pubstate = {published},
tppubtype = {inbook}
}
2022
Iacopo Colonnelli, Marco Aldinucci
Hybrid Workflows For Large - Scale Scientific Applications Proceedings Article
In: Sixth EAGE High Performance Computing Workshop, pp. 1–5, European Association of Geoscientists & Engineers , Milano, Italy, 2022, ISSN: 2214-4609.
Abstract | Links | BibTeX | Tags: across, eupex
@inproceedings{22:eage-hpc-workshop,
title = {Hybrid Workflows For Large - Scale Scientific Applications},
author = {Iacopo Colonnelli and Marco Aldinucci},
url = {https://iris.unito.it/retrieve/d79ddabb-f9d7-4a55-9f84-1528b1533ba3/Extended_Abstract.pdf},
doi = {10.3997/2214-4609.2022615029},
issn = {2214-4609},
year = {2022},
date = {2022-09-01},
booktitle = {Sixth EAGE High Performance Computing Workshop},
pages = {1–5},
publisher = {European Association of Geoscientists & Engineers },
address = {Milano, Italy},
abstract = {Large-scale scientific applications are facing an irrevrsible transition from monolithic, high-performance oriented codes to modular and polyglot deployments of specialised (micro-)services. The reasons behind this transition are many: coupling of standard solvers with Deep Learning techniques, offloading of data analysis and visualisation to Cloud, and the advent of specialised hardware accelerators. Topology-aware Workflow Management Systems (WMSs) play a crucial role. In particular, topology-awareness allows an explicit mapping of workflow steps onto heterogeneous locations, allowing automated executions on top of hybrid architectures (e.g., cloud+HPC or classical+quantum). Plus, topology-aware WMSs can offer nonfunctional requirements OOTB, e.g. components' life-cycle orchestration, secure and efficient data transfers, fault tolerance, and cross-cluster execution of urgent workloads. Augmenting interactive Jupyter Notebooks with distributed workflow capabilities allows domain experts to prototype and scale applications using the same technological stack, while relying on a feature-rich and user-friendly web interface. This abstract will showcase how these general methodologies can be applied to a typical geoscience simulation pipeline based on the Full Wavefront Inversion (FWI) technique. In particular, a prototypical Jupyter Notebook will be executed interactively on Cloud. Preliminary data analyses and post-processing will be executed locally, while the computationally demanding optimisation loop will be scheduled on a remote HPC cluster.},
keywords = {across, eupex},
pubstate = {published},
tppubtype = {inproceedings}
}
Giovanni Agosta, Marco Aldinucci, Carlos Alvarez, Roberto Ammendola, Yasir Arfat, Olivier Beaumont, Massimo Bernaschi, Andrea Biagioni, Tommaso Boccali, Berenger Bramas, Carlo Brandolese, Barbara Cantalupo, Mauro Carrozzo, Daniele Cattaneo, Alessandro Celestini, Massimo Celino, Iacopo Colonnelli, Paolo Cretaro, Pasqua D'Ambra, Marco Danelutto, Roberto Esposito, Lionel Eyraud-Dubois, Antonio Filgueras, William Fornaciari, Ottorino Frezza, Andrea Galimberti, Francesco Giacomini, Brice Goglin, Daniele Gregori, Abdou Guermouche, Francesco Iannone, Michal Kulczewski, Francesca Lo Cicero, Alessandro Lonardo, Alberto R. Martinelli, Michele Martinelli, Xavier Martorell, Giuseppe Massari, Simone Montangero, Gianluca Mittone, Raymond Namyst, Ariel Oleksiak, Paolo Palazzari, Pier Stanislao Paolucci, Federico Reghenzani, Cristian Rossi, Sergio Saponara, Francesco Simula, Federico Terraneo, Samuel Thibault, Massimo Torquati, Matteo Turisini, Piero Vicini, Miquel Vidal, Davide Zoni, Giuseppe Zummo
Towards EXtreme scale technologies and accelerators for euROhpc hw/Sw supercomputing applications for exascale: The TEXTAROSSA approach Journal Article
In: Microprocessors and Microsystems, vol. 95, pp. 104679, 2022, ISSN: 0141-9331.
Abstract | Links | BibTeX | Tags: textarossa
@article{textarossa2022micpro:,
title = {Towards EXtreme scale technologies and accelerators for euROhpc hw/Sw supercomputing applications for exascale: The TEXTAROSSA approach},
author = {Giovanni Agosta and Marco Aldinucci and Carlos Alvarez and Roberto Ammendola and Yasir Arfat and Olivier Beaumont and Massimo Bernaschi and Andrea Biagioni and Tommaso Boccali and Berenger Bramas and Carlo Brandolese and Barbara Cantalupo and Mauro Carrozzo and Daniele Cattaneo and Alessandro Celestini and Massimo Celino and Iacopo Colonnelli and Paolo Cretaro and Pasqua D'Ambra and Marco Danelutto and Roberto Esposito and Lionel Eyraud-Dubois and Antonio Filgueras and William Fornaciari and Ottorino Frezza and Andrea Galimberti and Francesco Giacomini and Brice Goglin and Daniele Gregori and Abdou Guermouche and Francesco Iannone and Michal Kulczewski and Francesca Lo Cicero and Alessandro Lonardo and Alberto R. Martinelli and Michele Martinelli and Xavier Martorell and Giuseppe Massari and Simone Montangero and Gianluca Mittone and Raymond Namyst and Ariel Oleksiak and Paolo Palazzari and Pier Stanislao Paolucci and Federico Reghenzani and Cristian Rossi and Sergio Saponara and Francesco Simula and Federico Terraneo and Samuel Thibault and Massimo Torquati and Matteo Turisini and Piero Vicini and Miquel Vidal and Davide Zoni and Giuseppe Zummo},
doi = {10.1016/j.micpro.2022.104679},
issn = {0141-9331},
year = {2022},
date = {2022-01-01},
journal = {Microprocessors and Microsystems},
volume = {95},
pages = {104679},
abstract = {In the near future, Exascale systems will need to bridge three technology gaps to achieve high performance while remaining under tight power constraints: energy efficiency and thermal control; extreme computation efficiency via HW acceleration and new arithmetic; methods and tools for seamless integration of reconfigurable accelerators in heterogeneous HPC multi-node platforms. TEXTAROSSA addresses these gaps through a co-design approach to heterogeneous HPC solutions, supported by the integration and extension of HW and SW IPs, programming models, and tools derived from European research.},
keywords = {textarossa},
pubstate = {published},
tppubtype = {article}
}
Marco Aldinucci, David Atienza, Federico Bolelli, Mónica Caballero, Iacopo Colonnelli, José Flich, Jon Ander Gómez, David González, Costantino Grana, Marco Grangetto, Simone Leo, Pedro López, Dana Oniga, Roberto Paredes, Luca Pireddu, Eduardo Quiñones, Tatiana Silva, Enzo Tartaglione, Marina Zapater
In: Curry, Edward, Auer, Sören, Berre, Arne J., Metzger, Andreas, Perez, Maria S., Zillner, Sonja (Ed.): Technologies and Applications for Big Data Value, pp. 183–202, Springer International Publishing, Cham, 2022, ISBN: 978-3-030-78307-5.
Abstract | Links | BibTeX | Tags: deephealth, streamflow
@incollection{22:TABDV,
title = {The DeepHealth Toolkit: A Key European Free and Open-Source Software for Deep Learning and Computer Vision Ready to Exploit Heterogeneous HPC and Cloud Architectures},
author = {Marco Aldinucci and David Atienza and Federico Bolelli and Mónica Caballero and Iacopo Colonnelli and José Flich and Jon Ander Gómez and David González and Costantino Grana and Marco Grangetto and Simone Leo and Pedro López and Dana Oniga and Roberto Paredes and Luca Pireddu and Eduardo Quiñones and Tatiana Silva and Enzo Tartaglione and Marina Zapater},
editor = {Edward Curry and Sören Auer and Arne J. Berre and Andreas Metzger and Maria S. Perez and Sonja Zillner},
url = {https://link.springer.com/content/pdf/10.1007/978-3-030-78307-5_9.pdf},
doi = {10.1007/978-3-030-78307-5_9},
isbn = {978-3-030-78307-5},
year = {2022},
date = {2022-01-01},
booktitle = {Technologies and Applications for Big Data Value},
pages = {183–202},
publisher = {Springer International Publishing},
address = {Cham},
chapter = {9},
abstract = {At the present time, we are immersed in the convergence between Big Data, High-Performance Computing and Artificial Intelligence. Technological progress in these three areas has accelerated in recent years, forcing different players like software companies and stakeholders to move quickly. The European Union is dedicating a lot of resources to maintain its relevant position in this scenario, funding projects to implement large-scale pilot testbeds that combine the latest advances in Artificial Intelligence, High-Performance Computing, Cloud and Big Data technologies. The DeepHealth project is an example focused on the health sector whose main outcome is the DeepHealth toolkit, a European unified framework that offers deep learning and computer vision capabilities, completely adapted to exploit underlying heterogeneous High-Performance Computing, Big Data and cloud architectures, and ready to be integrated into any software platform to facilitate the development and deployment of new applications for specific problems in any sector. This toolkit is intended to be one of the European contributions to the field of AI. This chapter introduces the toolkit with its main components and complementary tools, providing a clear view to facilitate and encourage its adoption and wide use by the European community of developers of AI-based solutions and data scientists working in the healthcare sector and others.},
keywords = {deephealth, streamflow},
pubstate = {published},
tppubtype = {incollection}
}
Eduardo Quiñones, Jesus Perales, Jorge Ejarque, Asaf Badouh, Santiago Marco, Fabrice Auzanneau, François Galea, David González, José Ramón Hervás, Tatiana Silva, Iacopo Colonnelli, Barbara Cantalupo, Marco Aldinucci, Enzo Tartaglione, Rafael Tornero, José Flich, Jose Maria Martinez, David Rodriguez, Izan Catalán, Jorge Garcia, Carles Hernández
In: Terzo, Olivier, Martinovič, Jan (Ed.): HPC, Big Data, and AI Convergence Towards Exascale: Challenge and Vision, pp. 191–216, CRC Press, Boca Raton, Florida, 2022, ISBN: 978-1-0320-0984-1.
Abstract | Links | BibTeX | Tags: deephealth, streamflow
@incollection{22:deephealth:HPCbook,
title = {The DeepHealth HPC Infrastructure: Leveraging Heterogenous HPC and Cloud Computing Infrastructures for IA-based Medical Solutions},
author = {Eduardo Quiñones and Jesus Perales and Jorge Ejarque and Asaf Badouh and Santiago Marco and Fabrice Auzanneau and François Galea and David González and José Ramón Hervás and Tatiana Silva and Iacopo Colonnelli and Barbara Cantalupo and Marco Aldinucci and Enzo Tartaglione and Rafael Tornero and José Flich and Jose Maria Martinez and David Rodriguez and Izan Catalán and Jorge Garcia and Carles Hernández},
editor = {Olivier Terzo and Jan Martinovič},
url = {https://iris.unito.it/retrieve/handle/2318/1832050/912413/Preprint.pdf},
doi = {10.1201/9781003176664},
isbn = {978-1-0320-0984-1},
year = {2022},
date = {2022-01-01},
booktitle = {HPC, Big Data, and AI Convergence Towards Exascale: Challenge and Vision},
pages = {191–216},
publisher = {CRC Press},
address = {Boca Raton, Florida},
chapter = {10},
abstract = {This chapter presents the DeepHealth HPC toolkit for an efficient execution of deep learning (DL) medical application into HPC and cloud-computing infrastructures, featuring many-core, GPU, and FPGA acceleration devices. The toolkit offers to the European Computer Vision Library and the European Distributed Deep Learning Library (EDDL), developed in the DeepHealth project as well, the mechanisms to distribute and parallelize DL operations on HPC and cloud infrastructures in a fully transparent way. The toolkit implements workflow managers used to orchestrate HPC workloads for an efficient parallelization of EDDL training operations on HPC and cloud infrastructures, and includes the parallel programming models for an efficient execution EDDL inference and training operations on many-core, GPUs and FPGAs acceleration devices.},
keywords = {deephealth, streamflow},
pubstate = {published},
tppubtype = {incollection}
}
Iacopo Colonnelli, Marco Aldinucci, Barbara Cantalupo, Luca Padovani, Sergio Rabellino, Concetto Spampinato, Roberto Morelli, Rosario Di Carlo, Nicolò Magini, Carlo Cavazzoni
Distributed workflows with Jupyter Journal Article
In: Future Generation Computer Systems, vol. 128, pp. 282–298, 2022, ISSN: 0167-739X.
Abstract | Links | BibTeX | Tags: across, deephealth, jupyter-workflow, streamflow
@article{21:FGCS:jupyflow,
title = {Distributed workflows with Jupyter},
author = {Iacopo Colonnelli and Marco Aldinucci and Barbara Cantalupo and Luca Padovani and Sergio Rabellino and Concetto Spampinato and Roberto Morelli and Rosario Di Carlo and Nicolò Magini and Carlo Cavazzoni},
url = {https://www.sciencedirect.com/science/article/pii/S0167739X21003976},
doi = {10.1016/j.future.2021.10.007},
issn = {0167-739X},
year = {2022},
date = {2022-01-01},
journal = {Future Generation Computer Systems},
volume = {128},
pages = {282–298},
abstract = {The designers of a new coordination interface enacting complex workflows have to tackle a dichotomy: choosing a language-independent or language-dependent approach. Language-independent approaches decouple workflow models from the host code's business logic and advocate portability. Language-dependent approaches foster flexibility and performance by adopting the same host language for business and coordination code. Jupyter Notebooks, with their capability to describe both imperative and declarative code in a unique format, allow taking the best of the two approaches, maintaining a clear separation between application and coordination layers but still providing a unified interface to both aspects. We advocate the Jupyter Notebooks' potential to express complex distributed workflows, identifying the general requirements for a Jupyter-based Workflow Management System (WMS) and introducing a proof-of-concept portable implementation working on hybrid Cloud-HPC infrastructures. As a byproduct, we extended the vanilla IPython kernel with workflow-based parallel and distributed execution capabilities. The proposed Jupyter-workflow (Jw) system is evaluated on common scenarios for High Performance Computing (HPC) and Cloud, showing its potential in lowering the barriers between prototypical Notebooks and production-ready implementations.},
keywords = {across, deephealth, jupyter-workflow, streamflow},
pubstate = {published},
tppubtype = {article}
}
2021
Giovanni Agosta, William Fornaciari, Andrea Galimberti, Giuseppe Massari, Federico Reghenzani, Federico Terraneo, Davide Zoni, Carlo Brandolese, Massimo Celino, Francesco Iannone, Paolo Palazzari, Giuseppe Zummo, Massimo Bernaschi, Pasqua D'Ambra, Sergio Saponara, Marco Danelutto, Massimo Torquati, Marco Aldinucci, Yasir Arfat, Barbara Cantalupo, Iacopo Colonnelli, Roberto Esposito, Alberto Riccardo Martinelli, Gianluca Mittone, Olivier Beaumont, Berenger Bramas, Lionel Eyraud-Dubois, Brice Goglin, Abdou Guermouche, Raymond Namyst, Samuel Thibault, Antonio Filgueras, Miquel Vidal, Carlos Alvarez, Xavier Martorell, Ariel Oleksiak, Michal Kulczewski, Alessandro Lonardo, Piero Vicini, Francesco Lo Cicero, Francesco Simula, Andrea Biagioni, Paolo Cretaro, Ottorino Frezza, Pier Stanislao Paolucci, Matteo Turisini, Francesco Giacomini, Tommaso Boccali, Simone Montangero, Roberto Ammendola
TEXTAROSSA: Towards EXtreme scale Technologies and Accelerators for euROhpc hw/Sw Supercomputing Applications for exascale Proceedings Article
In: Proc. of the 24th Euromicro Conference on Digital System Design (DSD), IEEE, Palermo, Italy, 2021.
Abstract | Links | BibTeX | Tags: streamflow, textarossa
@inproceedings{21:DSD:textarossa,
title = {TEXTAROSSA: Towards EXtreme scale Technologies and Accelerators for euROhpc hw/Sw Supercomputing Applications for exascale},
author = {Giovanni Agosta and William Fornaciari and Andrea Galimberti and Giuseppe Massari and Federico Reghenzani and Federico Terraneo and Davide Zoni and Carlo Brandolese and Massimo Celino and Francesco Iannone and Paolo Palazzari and Giuseppe Zummo and Massimo Bernaschi and Pasqua D'Ambra and Sergio Saponara and Marco Danelutto and Massimo Torquati and Marco Aldinucci and Yasir Arfat and Barbara Cantalupo and Iacopo Colonnelli and Roberto Esposito and Alberto Riccardo Martinelli and Gianluca Mittone and Olivier Beaumont and Berenger Bramas and Lionel Eyraud-Dubois and Brice Goglin and Abdou Guermouche and Raymond Namyst and Samuel Thibault and Antonio Filgueras and Miquel Vidal and Carlos Alvarez and Xavier Martorell and Ariel Oleksiak and Michal Kulczewski and Alessandro Lonardo and Piero Vicini and Francesco Lo Cicero and Francesco Simula and Andrea Biagioni and Paolo Cretaro and Ottorino Frezza and Pier Stanislao Paolucci and Matteo Turisini and Francesco Giacomini and Tommaso Boccali and Simone Montangero and Roberto Ammendola},
doi = {10.1109/DSD53832.2021.00051},
year = {2021},
date = {2021-08-01},
booktitle = {Proc. of the 24th Euromicro Conference on Digital System Design (DSD)},
publisher = {IEEE},
address = {Palermo, Italy},
abstract = {To achieve high performance and high energy effi- ciency on near-future exascale computing systems, three key technology gaps needs to be bridged. These gaps include: en- ergy efficiency and thermal control; extreme computation effi- ciency via HW acceleration and new arithmetics; methods and tools for seamless integration of reconfigurable accelerators in heterogeneous HPC multi-node platforms. TEXTAROSSA aims at tackling this gap through a co-design approach to heterogeneous HPC solutions, supported by the integration and extension of HW and SW IPs, programming models and tools derived from European research.},
keywords = {streamflow, textarossa},
pubstate = {published},
tppubtype = {inproceedings}
}
Marco Aldinucci, Valentina Cesare, Iacopo Colonnelli, Alberto Riccardo Martinelli, Gianluca Mittone, Barbara Cantalupo
Practical Parallelizazion of a Laplace Solver with MPI Proceedings Article
In: Iannone, Francesco (Ed.): ENEA CRESCO in the fight against COVID-19, pp. 21–24, ENEA, 2021.
Abstract | BibTeX | Tags: hpc4ai
@inproceedings{21:laplace:enea,
title = {Practical Parallelizazion of a Laplace Solver with MPI},
author = {Marco Aldinucci and Valentina Cesare and Iacopo Colonnelli and Alberto Riccardo Martinelli and Gianluca Mittone and Barbara Cantalupo},
editor = {Francesco Iannone},
year = {2021},
date = {2021-01-01},
booktitle = {ENEA CRESCO in the fight against COVID-19},
pages = {21–24},
publisher = {ENEA},
abstract = {This work exposes a practical methodology for the semi-automatic parallelization of existing code. We show how a scientific sequential code can be parallelized through our approach. The obtained parallel code is only slightly different from the starting sequential one, providing an example of how little re-designing our methodology involves. The performance of the parallelized code, executed on the CRESCO6 cluster, is then exposed and discussed. We also believe in the educational value of this approach and suggest its use as a teaching device for students.},
keywords = {hpc4ai},
pubstate = {published},
tppubtype = {inproceedings}
}
Iacopo Colonnelli, Barbara Cantalupo, Concetto Spampinato, Matteo Pennisi, Marco Aldinucci
Bringing AI pipelines onto cloud-HPC: setting a baseline for accuracy of COVID-19 diagnosis Proceedings Article
In: Iannone, Francesco (Ed.): ENEA CRESCO in the fight against COVID-19, ENEA, 2021.
Abstract | Links | BibTeX | Tags: streamflow
@inproceedings{21:covi:enea,
title = {Bringing AI pipelines onto cloud-HPC: setting a baseline for accuracy of COVID-19 diagnosis},
author = {Iacopo Colonnelli and Barbara Cantalupo and Concetto Spampinato and Matteo Pennisi and Marco Aldinucci},
editor = {Francesco Iannone},
url = {https://iris.unito.it/retrieve/handle/2318/1796029/779853/21_AI-pipelines_ENEA-COVID19.pdf},
doi = {10.5281/zenodo.5151511},
year = {2021},
date = {2021-01-01},
booktitle = {ENEA CRESCO in the fight against COVID-19},
publisher = {ENEA},
abstract = {HPC is an enabling platform for AI. The introduction of AI workloads in the HPC applications basket has non-trivial consequences both on the way of designing AI applications and on the way of providing HPC computing. This is the leitmotif of the convergence between HPC and AI. The formalized definition of AI pipelines is one of the milestones of HPC-AI convergence. If well conducted, it allows, on the one hand, to obtain portable and scalable applications. On the other hand, it is crucial for the reproducibility of scientific pipelines. In this work, we advocate the StreamFlow Workflow Management System as a crucial ingredient to define a parametric pipeline, called ``CLAIRE COVID-19 Universal Pipeline'', which is able to explore the optimization space of methods to classify COVID-19 lung lesions from CT scans, compare them for accuracy, and therefore set a performance baseline. The universal pipeline automatizes the training of many different Deep Neural Networks (DNNs) and many different hyperparameters. It, therefore, requires a massive computing power, which is found in traditional HPC infrastructure thanks to the portability-by-design of pipelines designed with StreamFlow. Using the universal pipeline, we identified a DNN reaching over 90% accuracy in detecting COVID-19 lesions in CT scans.},
keywords = {streamflow},
pubstate = {published},
tppubtype = {inproceedings}
}
Ovidio De Filippo, Jeehoon Kang, Francesco Bruno, Jung-Kyu Han, Andrea Saglietto, Han-Mo Yang, Giuseppe Patti, Kyung-Woo Park, Radoslaw Parma, Hyo-Soo Kim, Leonardo De Luca, Hyeon-Cheol Gwon, Mario Iannaccone, Woo Jung Chun, Grzegorz Smolka, Seung-Ho Hur, Enrico Cerrato, Seung Hwan Han, Carlo Mario, Young Bin Song, Javier Escaned, Ki Hong Choi, Gerard Helft, Joon-Hyung Doh, Alessandra Truffa Giachet, Soon-Jun Hong, Saverio Muscoli, Chang-Wook Nam, Guglielmo Gallone, Davide Capodanno, Daniela Trabattoni, Yoichi Imori, Veronica Dusi, Bernardo Cortese, Antonio Montefusco, Federico Conrotto, Iacopo Colonnelli, Imad Sheiban, Gaetano Maria Ferrari, Bon-Kwon Koo, Fabrizio D'Ascenzo
In: The American Journal of Cardiology, 2021, ISSN: 0002-9149.
Abstract | Links | BibTeX | Tags: ai
@article{21:ajc:bifurcat,
title = {Benefit of Extended Dual Antiplatelet Therapy Duration in Acute Coronary Syndrome Patients Treated with Drug Eluting Stents for Coronary Bifurcation Lesions (from the BIFURCAT Registry)},
author = {Ovidio De Filippo and Jeehoon Kang and Francesco Bruno and Jung-Kyu Han and Andrea Saglietto and Han-Mo Yang and Giuseppe Patti and Kyung-Woo Park and Radoslaw Parma and Hyo-Soo Kim and Leonardo De Luca and Hyeon-Cheol Gwon and Mario Iannaccone and Woo Jung Chun and Grzegorz Smolka and Seung-Ho Hur and Enrico Cerrato and Seung Hwan Han and Carlo Mario and Young Bin Song and Javier Escaned and Ki Hong Choi and Gerard Helft and Joon-Hyung Doh and Alessandra Truffa Giachet and Soon-Jun Hong and Saverio Muscoli and Chang-Wook Nam and Guglielmo Gallone and Davide Capodanno and Daniela Trabattoni and Yoichi Imori and Veronica Dusi and Bernardo Cortese and Antonio Montefusco and Federico Conrotto and Iacopo Colonnelli and Imad Sheiban and Gaetano Maria Ferrari and Bon-Kwon Koo and Fabrizio D'Ascenzo},
url = {https://www.sciencedirect.com/science/article/pii/S0002914921006354},
doi = {10.1016/j.amjcard.2021.07.005},
issn = {0002-9149},
year = {2021},
date = {2021-01-01},
journal = {The American Journal of Cardiology},
abstract = {Optimal dual antiplatelet therapy (DAPT) duration for patients undergoing percutaneous coronary intervention (PCI) for coronary bifurcations is an unmet issue. The BIFURCAT registry was obtained by merging two registries on coronary bifurcations. Three groups were compared in a two-by-two fashion: short-term DAPT (≤ 6 months), intermediate-term DAPT (6-12 months) and extended DAPT (>12 months). Major adverse cardiac events (MACE) (a composite of all-cause death, myocardial infarction (MI), target-lesion revascularization and stent thrombosis) were the primary endpoint. Single components of MACE were the secondary endpoints. Events were appraised according to the clinical presentation: chronic coronary syndrome (CCS) versus acute coronary syndrome (ACS). 5537 patients (3231 ACS, 2306 CCS) were included. After a median follow-up of 2.1 years (IQR 0.9-2.2), extended DAPT was associated with a lower incidence of MACE compared with intermediate-term DAPT (2.8% versus 3.4%, adjusted HR 0.23 [0.1-0.54], p <0.001), driven by a reduction of all-cause death in the ACS cohort. In the CCS cohort, an extended DAPT strategy was not associated with a reduced risk of MACE. In conclusion, among real-world patients receiving PCI for coronary bifurcation, an extended DAPT strategy was associated with a reduction of MACE in ACS but not in CCS patients.},
keywords = {ai},
pubstate = {published},
tppubtype = {article}
}
Marco Aldinucci, Valentina Cesare, Iacopo Colonnelli, Alberto Riccardo Martinelli, Gianluca Mittone, Barbara Cantalupo, Carlo Cavazzoni, Maurizio Drocco
Practical Parallelization of Scientific Applications with OpenMP, OpenACC and MPI Journal Article
In: Journal of Parallel and Distributed Computing, vol. 157, pp. 13–29, 2021.
Abstract | Links | BibTeX | Tags: HPC
@article{21:jpdc:loop,
title = {Practical Parallelization of Scientific Applications with OpenMP, OpenACC and MPI},
author = {Marco Aldinucci and Valentina Cesare and Iacopo Colonnelli and Alberto Riccardo Martinelli and Gianluca Mittone and Barbara Cantalupo and Carlo Cavazzoni and Maurizio Drocco},
url = {https://iris.unito.it/retrieve/handle/2318/1792557/770851/Practical_Parallelization_JPDC_preprint.pdf},
doi = {10.1016/j.jpdc.2021.05.017},
year = {2021},
date = {2021-01-01},
journal = {Journal of Parallel and Distributed Computing},
volume = {157},
pages = {13–29},
abstract = {This work aims at distilling a systematic methodology to modernize existing sequential scientific codes with a little re-designing effort, turning an old codebase into emphmodern code, i.e., parallel and robust code. We propose a semi-automatic methodology to parallelize scientific applications designed with a purely sequential programming mindset, possibly using global variables, aliasing, random number generators, and stateful functions. We demonstrate that the same methodology works for the parallelization in the shared memory model (via OpenMP), message passing model (via MPI), and General Purpose Computing on GPU model (via OpenACC). The method is demonstrated parallelizing four real-world sequential codes in the domain of physics and material science. The methodology itself has been distilled in collaboration with MSc students of the Parallel Computing course at the University of Torino, that applied it for the first time to the project works that they presented for the final exam of the course. Every year the course hosts some special lectures from industry representatives, who present how they use parallel computing and offer codes to be parallelized.},
keywords = {HPC},
pubstate = {published},
tppubtype = {article}
}
Iacopo Colonnelli, Barbara Cantalupo, Roberto Esposito, Matteo Pennisi, Concetto Spampinato, Marco Aldinucci
HPC Application Cloudification: The StreamFlow Toolkit Proceedings Article
In: Bispo, João, Cherubin, Stefano, Flich, José (Ed.): 12th Workshop on Parallel Programming and Run-Time Management Techniques for Many-core Architectures and 10th Workshop on Design Tools and Architectures for Multicore Embedded Computing Platforms (PARMA-DITAM 2021), pp. 5:1–5:13, Schloss Dagstuhl – Leibniz-Zentrum für Informatik, Dagstuhl, Germany, 2021, ISSN: 2190-6807.
Abstract | Links | BibTeX | Tags: deephealth, hpc4ai, streamflow
@inproceedings{colonnelli_et_al:OASIcs.PARMA-DITAM.2021.5,
title = {HPC Application Cloudification: The StreamFlow Toolkit},
author = {Iacopo Colonnelli and Barbara Cantalupo and Roberto Esposito and Matteo Pennisi and Concetto Spampinato and Marco Aldinucci},
editor = {João Bispo and Stefano Cherubin and José Flich},
url = {https://drops.dagstuhl.de/opus/volltexte/2021/13641/pdf/OASIcs-PARMA-DITAM-2021-5.pdf},
doi = {10.4230/OASIcs.PARMA-DITAM.2021.5},
issn = {2190-6807},
year = {2021},
date = {2021-01-01},
booktitle = {12th Workshop on Parallel Programming and Run-Time Management Techniques for Many-core Architectures and 10th Workshop on Design Tools and Architectures for Multicore Embedded Computing Platforms (PARMA-DITAM 2021)},
volume = {88},
pages = {5:1–5:13},
publisher = {Schloss Dagstuhl – Leibniz-Zentrum für Informatik},
address = {Dagstuhl, Germany},
series = {Open Access Series in Informatics (OASIcs)},
abstract = {Finding an effective way to improve accessibility to High-Performance Computing facilities, still anchored to SSH-based remote shells and queue-based job submission mechanisms, is an open problem in computer science. This work advocates a cloudification of HPC applications through a cluster-as-accelerator pattern, where computationally demanding portions of the main execution flow hosted on a Cloud Finding an effective way to improve accessibility to High-Performance Computing facilities, still anchored to SSH-based remote shells and queue-based job submission mechanisms, is an open problem in computer science. This work advocates a cloudification of HPC applications through a cluster-as-accelerator pattern, where computationally demanding portions of the main execution flow hosted on a Cloud infrastructure can be offloaded to HPC environments to speed them up. We introduce StreamFlow, a novel Workflow Management System that supports such a design pattern and makes it possible to run the steps of a standard workflow model on independent processing elements with no shared storage. We validated the proposed approach's effectiveness on the CLAIRE COVID-19 universal pipeline, i.e. a reproducible workflow capable of automating the comparison of (possibly all) state-of-the-art pipelines for the diagnosis of COVID-19 interstitial pneumonia from CT scans images based on Deep Neural Networks (DNNs).},
keywords = {deephealth, hpc4ai, streamflow},
pubstate = {published},
tppubtype = {inproceedings}
}
Fabrizio D'Ascenzo, Ovidio De Filippo, Guglielmo Gallone, Gianluca Mittone, Marco Agostino Deriu, Mario Iannaccone, Albert Ariza-Solé, Christoph Liebetrau, Sergio Manzano-Fernández, Giorgio Quadri, Tim Kinnaird, Gianluca Campo, Jose Paulo Simao Henriques, James M Hughes, Alberto Dominguez-Rodriguez, Marco Aldinucci, Umberto Morbiducci, Giuseppe Patti, Sergio Raposeiras-Roubin, Emad Abu-Assi, Gaetano Maria De Ferrari, Francesco Piroli, Andrea Saglietto, Federico Conrotto, Pierluigi Omedé, Antonio Montefusco, Mauro Pennone, Francesco Bruno, Pier Paolo Bocchino, Giacomo Boccuzzi, Enrico Cerrato, Ferdinando Varbella, Michela Sperti, Stephen B. Wilton, Lazar Velicki, Ioanna Xanthopoulou, Angel Cequier, Andres Iniguez-Romo, Isabel Munoz Pousa, Maria Cespon Fernandez, Berenice Caneiro Queija, Rafael Cobas-Paz, Angel Lopez-Cuenca, Alberto Garay, Pedro Flores Blanco, Andrea Rognoni, Giuseppe Biondi Zoccai, Simone Biscaglia, Ivan Nunez-Gil, Toshiharu Fujii, Alessandro Durante, Xiantao Song, Tetsuma Kawaji, Dimitrios Alexopoulos, Zenon Huczek, Jose Ramon Gonzalez Juanatey, Shao-Ping Nie, Masa-aki Kawashiri, Iacopo Colonnelli, Barbara Cantalupo, Roberto Esposito, Sergio Leonardi, Walter Grosso Marra, Alaide Chieffo, Umberto Michelucci, Dario Piga, Marta Malavolta, Sebastiano Gili, Marco Mennuni, Claudio Montalto, Luigi Oltrona Visconti, Yasir Arfat
Machine learning-based prediction of adverse events following an acute coronary syndrome (PRAISE): a modelling study of pooled datasets Journal Article
In: The Lancet, vol. 397, no. 10270, pp. 199–207, 2021, ISSN: 0140-6736.
Abstract | Links | BibTeX | Tags: deephealth, hpc4ai
@article{21:lancet,
title = {Machine learning-based prediction of adverse events following an acute coronary syndrome (PRAISE): a modelling study of pooled datasets},
author = {Fabrizio D'Ascenzo and Ovidio De Filippo and Guglielmo Gallone and Gianluca Mittone and Marco Agostino Deriu and Mario Iannaccone and Albert Ariza-Solé and Christoph Liebetrau and Sergio Manzano-Fernández and Giorgio Quadri and Tim Kinnaird and Gianluca Campo and Jose Paulo Simao Henriques and James M Hughes and Alberto Dominguez-Rodriguez and Marco Aldinucci and Umberto Morbiducci and Giuseppe Patti and Sergio Raposeiras-Roubin and Emad Abu-Assi and Gaetano Maria De Ferrari and Francesco Piroli and Andrea Saglietto and Federico Conrotto and Pierluigi Omedé and Antonio Montefusco and Mauro Pennone and Francesco Bruno and Pier Paolo Bocchino and Giacomo Boccuzzi and Enrico Cerrato and Ferdinando Varbella and Michela Sperti and Stephen B. Wilton and Lazar Velicki and Ioanna Xanthopoulou and Angel Cequier and Andres Iniguez-Romo and Isabel Munoz Pousa and Maria Cespon Fernandez and Berenice Caneiro Queija and Rafael Cobas-Paz and Angel Lopez-Cuenca and Alberto Garay and Pedro Flores Blanco and Andrea Rognoni and Giuseppe Biondi Zoccai and Simone Biscaglia and Ivan Nunez-Gil and Toshiharu Fujii and Alessandro Durante and Xiantao Song and Tetsuma Kawaji and Dimitrios Alexopoulos and Zenon Huczek and Jose Ramon Gonzalez Juanatey and Shao-Ping Nie and Masa-aki Kawashiri and Iacopo Colonnelli and Barbara Cantalupo and Roberto Esposito and Sergio Leonardi and Walter Grosso Marra and Alaide Chieffo and Umberto Michelucci and Dario Piga and Marta Malavolta and Sebastiano Gili and Marco Mennuni and Claudio Montalto and Luigi Oltrona Visconti and Yasir Arfat},
url = {https://www.researchgate.net/profile/James_Hughes3/publication/348501148_Machine_learning-based_prediction_of_adverse_events_following_an_acute_coronary_syndrome_PRAISE_a_modelling_study_of_pooled_datasets/links/6002a81ba6fdccdcb858b6c2/Machine-learning-based-prediction-of-adverse-events-following-an-acute-coronary-syndrome-PRAISE-a-modelling-study-of-pooled-datasets.pdf},
doi = {10.1016/S0140-6736(20)32519-8},
issn = {0140-6736},
year = {2021},
date = {2021-01-01},
journal = {The Lancet},
volume = {397},
number = {10270},
pages = {199–207},
abstract = {Background The accuracy of current prediction tools for ischaemic and bleeding events after an acute coronary syndrome (ACS) remains insufficient for individualised patient management strategies. We developed a machine learning-based risk stratification model to predict all-cause death, recurrent acute myocardial infarction, and major bleeding after ACS. Methods Different machine learning models for the prediction of 1-year post-discharge all-cause death, myocardial infarction, and major bleeding (defined as Bleeding Academic Research Consortium type 3 or 5) were trained on a cohort of 19826 adult patients with ACS (split into a training cohort [80%] and internal validation cohort [20%]) from the BleeMACS and RENAMI registries, which included patients across several continents. 25 clinical features routinely assessed at discharge were used to inform the models. The best-performing model for each study outcome (the PRAISE score) was tested in an external validation cohort of 3444 patients with ACS pooled from a randomised controlled trial and three prospective registries. Model performance was assessed according to a range of learning metrics including area under the receiver operating characteristic curve (AUC). Findings The PRAISE score showed an AUC of 0.82 (95% CI 0.78-0.85) in the internal validation cohort and 0.92 (0.90-0.93) in the external validation cohort for 1-year all-cause death; an AUC of 0.74 (0.70-0.78) in the internal validation cohort and 0.81 (0.76-0.85) in the external validation cohort for 1-year myocardial infarction; and an AUC of 0.70 (0.66-0.75) in the internal validation cohort and 0.86 (0.82-0.89) in the external validation cohort for 1-year major bleeding. Interpretation A machine learning-based approach for the identification of predictors of events after an ACS is feasible and effective. The PRAISE score showed accurate discriminative capabilities for the prediction of all-cause death, myocardial infarction, and major bleeding, and might be useful to guide clinical decision making.},
keywords = {deephealth, hpc4ai},
pubstate = {published},
tppubtype = {article}
}
Iacopo Colonnelli, Barbara Cantalupo, Ivan Merelli, Marco Aldinucci
StreamFlow: cross-breeding cloud with HPC Journal Article
In: IEEE Transactions on Emerging Topics in Computing, vol. 9, no. 4, pp. 1723–1737, 2021.
Abstract | Links | BibTeX | Tags: deephealth, hpc4ai, streamflow
@article{20Lstreamflow:tetc,
title = {StreamFlow: cross-breeding cloud with HPC},
author = {Iacopo Colonnelli and Barbara Cantalupo and Ivan Merelli and Marco Aldinucci},
url = {https://arxiv.org/pdf/2002.01558},
doi = {10.1109/TETC.2020.3019202},
year = {2021},
date = {2021-01-01},
journal = {IEEE Transactions on Emerging Topics in Computing},
volume = {9},
number = {4},
pages = {1723–1737},
abstract = {Workflows are among the most commonly used tools in a variety of execution environments. Many of them target a specific environment; few of them make it possible to execute an entire workflow in different environments, e.g. Kubernetes and batch clusters. We present a novel approach to workflow execution, called StreamFlow, that complements the workflow graph with the declarative description of potentially complex execution environments, and that makes it possible the execution onto multiple sites not sharing a common data space. StreamFlow is then exemplified on a novel bioinformatics pipeline for single cell transcriptomic data analysis workflow.},
keywords = {deephealth, hpc4ai, streamflow},
pubstate = {published},
tppubtype = {article}
}
2020
Valentina Cesare, Iacopo Colonnelli, Marco Aldinucci
Practical Parallelization of Scientific Applications Proceedings Article
In: Proc. of 28th Euromicro Intl. Conference on Parallel Distributed and network-based Processing (PDP), pp. 376–384, IEEE, Västerås, Sweden, 2020.
Abstract | Links | BibTeX | Tags: c3s, hpc4ai
@inproceedings{20:looppar:pdp,
title = {Practical Parallelization of Scientific Applications},
author = {Valentina Cesare and Iacopo Colonnelli and Marco Aldinucci},
url = {https://iris.unito.it/retrieve/handle/2318/1735377/601141/2020_looppar_PDP.pdf},
doi = {10.1109/PDP50117.2020.00064},
year = {2020},
date = {2020-01-01},
booktitle = {Proc. of 28th Euromicro Intl. Conference on Parallel Distributed and network-based Processing (PDP)},
pages = {376–384},
publisher = {IEEE},
address = {Västerås, Sweden},
abstract = {This work aims at distilling a systematic methodology to modernize existing sequential scientific codes with a limited re-designing effort, turning an old codebase into modern code, i.e., parallel and robust code. We propose an automatable methodology to parallelize scientific applications designed with a purely sequential programming mindset, thus possibly using global variables, aliasing, random number generators, and stateful functions. We demonstrate the methodology by way of an astrophysical application, where we model at the same time the kinematic profiles of 30 disk galaxies with a Monte Carlo Markov Chain (MCMC), which is sequential by definition. The parallel code exhibits a 12 times speedup on a 48-core platform.},
keywords = {c3s, hpc4ai},
pubstate = {published},
tppubtype = {inproceedings}
}
2019
Paolo Viviani, Maurizio Drocco, Daniele Baccega, Iacopo Colonnelli, Marco Aldinucci
Deep Learning at Scale Proceedings Article
In: Proc. of 27th Euromicro Intl. Conference on Parallel Distributed and network-based Processing (PDP), pp. 124–131, IEEE, Pavia, Italy, 2019.
Abstract | Links | BibTeX | Tags: ai
@inproceedings{19:deeplearn:pdp,
title = {Deep Learning at Scale},
author = {Paolo Viviani and Maurizio Drocco and Daniele Baccega and Iacopo Colonnelli and Marco Aldinucci},
url = {https://iris.unito.it/retrieve/handle/2318/1695211/487778/19_deeplearning_PDP.pdf},
doi = {10.1109/EMPDP.2019.8671552},
year = {2019},
date = {2019-01-01},
booktitle = {Proc. of 27th Euromicro Intl. Conference on Parallel Distributed and network-based Processing (PDP)},
pages = {124–131},
publisher = {IEEE},
address = {Pavia, Italy},
abstract = {This work presents a novel approach to distributed training of deep neural networks (DNNs) that aims to overcome the issues related to mainstream approaches to data parallel training. Established techniques for data parallel training are discussed from both a parallel computing and deep learning perspective, then a different approach is presented that is meant to allow DNN training to scale while retaining good convergence properties. Moreover, an experimental implementation is presented as well as some preliminary results.},
keywords = {ai},
pubstate = {published},
tppubtype = {inproceedings}
}
Maurizio Drocco, Paolo Viviani, Iacopo Colonnelli, Marco Aldinucci, Marco Grangetto
Accelerating spectral graph analysis through wavefronts of linear algebra operations Proceedings Article
In: Proc. of 27th Euromicro Intl. Conference on Parallel Distributed and network-based Processing (PDP), pp. 9–16, IEEE, Pavia, Italy, 2019.
Abstract | Links | BibTeX | Tags:
@inproceedings{19:gsp:pdp,
title = {Accelerating spectral graph analysis through wavefronts of linear algebra operations},
author = {Maurizio Drocco and Paolo Viviani and Iacopo Colonnelli and Marco Aldinucci and Marco Grangetto},
url = {https://iris.unito.it/retrieve/handle/2318/1695315/488105/19_wavefront_PDP.pdf},
doi = {10.1109/EMPDP.2019.8671640},
year = {2019},
date = {2019-01-01},
booktitle = {Proc. of 27th Euromicro Intl. Conference on Parallel Distributed and network-based Processing (PDP)},
pages = {9–16},
publisher = {IEEE},
address = {Pavia, Italy},
abstract = {The wavefront pattern captures the unfolding of a parallel computation in which data elements are laid out as a logical multidimensional grid and the dependency graph favours a diagonal sweep across the grid. In the emerging area of spectral graph analysis, the computing often consists in a wavefront running over a tiled matrix, involving expensive linear algebra kernels. While these applications might benefit from parallel heterogeneous platforms (multi-core with GPUs),programming wavefront applications directly with high-performance linear algebra libraries yields code that is complex to write and optimize for the specific application. We advocate a methodology based on two abstractions (linear algebra and parallel pattern-based run-time), that allows to develop portable, self-configuring, and easy-to-profile code on hybrid platforms.},
keywords = {},
pubstate = {published},
tppubtype = {inproceedings}
}
Talks
2024
Iacopo Colonnelli
CWL in the HPC Ecosystem Miscellaneous
Workshop on workflow languages for HEP analysis, 2024.
Links | BibTeX | Tags: across, eupex, icsc, space, streamflow
@misc{24:icolonne:cwl4hpccern,
title = {CWL in the HPC Ecosystem},
author = {Iacopo Colonnelli},
url = {https://datacloud.di.unito.it/index.php/s/PRmqdwWHt6P2PH7},
year = {2024},
date = {2024-04-01},
address = {CERN, Meyrin, Switzerland},
howpublished = {Workshop on workflow languages for HEP analysis},
keywords = {across, eupex, icsc, space, streamflow},
pubstate = {published},
tppubtype = {misc}
}
2023
Iacopo Colonnelli, Robert Birke, Giulio Malenza, Gianluca Mittone, Alberto Mulone, Marco Aldinucci, Valerio Basile, Marco Antonio Stranisci, Viviana Patti, Jeroen Galjaard, Lydia Y. Chen, Sanzio Bassini, Massimiliano Guarrasi, Gabriella Scipione, Jan Martinovič, Vit Vondrák
Cross-Facility Federated Learning Miscellaneous
1st EuroHPC User Day, 2023.
Links | BibTeX | Tags: across, eupex, eupilot
@misc{23:eurohpc,
title = {Cross-Facility Federated Learning},
author = {Iacopo Colonnelli and Robert Birke and Giulio Malenza and Gianluca Mittone and Alberto Mulone and Marco Aldinucci and Valerio Basile and Marco Antonio Stranisci and Viviana Patti and Jeroen Galjaard and Lydia Y. Chen and Sanzio Bassini and Massimiliano Guarrasi and Gabriella Scipione and Jan Martinovič and Vit Vondrák},
url = {https://datacloud.di.unito.it/index.php/s/DDAz4QkJP3WZ68M},
year = {2023},
date = {2023-12-01},
address = {Bruxelles, Belgium},
howpublished = {1st EuroHPC User Day},
keywords = {across, eupex, eupilot},
pubstate = {published},
tppubtype = {misc}
}
Alberto Scionti, Iacopo Colonnelli
Orchestrating Multi-Domain Workflows: The ACROSS Approach Miscellaneous
Workflows Community: Modern Workflows for Continuum and Cross-Facility Computing, 2023.
Links | BibTeX | Tags: across, streamflow
@misc{23:sc:WCIBoF,
title = {Orchestrating Multi-Domain Workflows: The ACROSS Approach},
author = {Alberto Scionti and Iacopo Colonnelli},
url = {https://datacloud.di.unito.it/index.php/s/rJXcDBK4mLmS8yz},
year = {2023},
date = {2023-11-01},
address = {Denver, CO, Usa},
howpublished = {Workflows Community: Modern Workflows for Continuum and Cross-Facility Computing},
keywords = {across, streamflow},
pubstate = {published},
tppubtype = {misc}
}
Marco Aldinucci, Elena Baralis, Valeria Cardellini, Iacopo Colonnelli, Marco Danelutto, Sergio Decherchi, Giuseppe Di Modica, Luca Ferrucci, Marco Gribaudo, Francesco Iannone, Marco Lapegna, Doriana Medić, Giuseppa Muscianisi, Francesca Righetti, Eva Sciacca, Nicola Tonellotto, Mauro Tortonesi, Paolo Trunfio, Tullio Vardanega
A Systematic Mapping Study of Italian Research on Workflows Miscellaneous
18th Workshop on Workflows in Support of Large-Scale Science (WORKS 2023), 2023.
Abstract | Links | BibTeX | Tags: icsc
@misc{23:sc:works,
title = {A Systematic Mapping Study of Italian Research on Workflows},
author = {Marco Aldinucci and Elena Baralis and Valeria Cardellini and Iacopo Colonnelli and Marco Danelutto and Sergio Decherchi and Giuseppe Di Modica and Luca Ferrucci and Marco Gribaudo and Francesco Iannone and Marco Lapegna and Doriana Medić and Giuseppa Muscianisi and Francesca Righetti and Eva Sciacca and Nicola Tonellotto and Mauro Tortonesi and Paolo Trunfio and Tullio Vardanega},
url = {https://datacloud.di.unito.it/index.php/s/2kgooG43pGCykji},
year = {2023},
date = {2023-11-01},
address = {Denver, CO, Usa},
abstract = {An entire ecosystem of methodologies and tools revolves around scientific workflow management. They cover crucial non-functional requirements that standard workflow models fail to target, such as interactive execution, energy efficiency, performance portability, Big Data management, and intelligent orchestration in the Computing Continuum. Characterizing and monitoring this ecosystem is crucial to developing an informed view of current and future research directions. This work conducts a systematic mapping study of the Italian workflow research community, analyzing 25 tools and 10 applications from several scientific domains in the context of the ``National Research Centre for HPC, Big Data, and Quantum Computing'' (ICSC). The study aims to outline the main current research directions and determine how they address the critical needs of modern scientific applications. The findings highlight a variegated research ecosystem of tools, with a prominent interest in advanced workflow orchestration and still immature but promising efforts toward energy efficiency.},
howpublished = {18th Workshop on Workflows in Support of Large-Scale Science (WORKS 2023)},
keywords = {icsc},
pubstate = {published},
tppubtype = {misc}
}
Iacopo Colonnelli
ACROSS: HPC Big Data Artificial Intelligence Cross Stack Platform Towards Exascale Miscellaneous
LN HPC-KTT Assemblea Nazionale 2023, 2023.
Links | BibTeX | Tags: across, streamflow
@misc{23:AssembleaHPC-KTT,
title = {ACROSS: HPC Big Data Artificial Intelligence Cross Stack Platform Towards Exascale},
author = {Iacopo Colonnelli},
url = {https://datacloud.di.unito.it/index.php/s/aK7es8BgFeWorjD},
year = {2023},
date = {2023-10-01},
address = {Pisa, Italy},
howpublished = {LN HPC-KTT Assemblea Nazionale 2023},
keywords = {across, streamflow},
pubstate = {published},
tppubtype = {misc}
}
Iacopo Colonnelli, Doriana Medić, Barbara Cantalupo, Marco Aldinucci
Università degli Studi di Torino: Alpha parallel research group Miscellaneous
HaMMon Kick-Off meeting, 2023.
Links | BibTeX | Tags: icsc, streamflow
@misc{23:HaMMonProject,
title = {Università degli Studi di Torino: Alpha parallel research group},
author = {Iacopo Colonnelli and Doriana Medić and Barbara Cantalupo and Marco Aldinucci},
url = {https://datacloud.di.unito.it/index.php/s/cmgy9BZ3nwCR2QJ},
year = {2023},
date = {2023-10-01},
address = {Bologna, Italy},
howpublished = {HaMMon Kick-Off meeting},
keywords = {icsc, streamflow},
pubstate = {published},
tppubtype = {misc}
}
Iacopo Colonnelli
Workflow models for heterogeneous distributed systems Miscellaneous
2nd Italian Conference on Big Data and Data Science (ITADATA 2023), 2023, (Best PhD Thesis Award).
Links | BibTeX | Tags: jupyter-workflow, streamflow
@misc{23:ITADATABestPhDThesis,
title = {Workflow models for heterogeneous distributed systems},
author = {Iacopo Colonnelli},
url = {https://datacloud.di.unito.it/index.php/s/6RqcaJ4djqFNDC8},
year = {2023},
date = {2023-09-01},
address = {Napoli, Italy},
howpublished = {2nd Italian Conference on Big Data and Data Science (ITADATA 2023)},
note = {Best PhD Thesis Award},
keywords = {jupyter-workflow, streamflow},
pubstate = {published},
tppubtype = {misc}
}
Gianluca Mittone, Walter Riviera, Iacopo Colonnelli, Robert Birke, Marco Aldinucci
Model-Agnostic Federated Learning Miscellaneous
29th International European Conference on Parallel and Distributed Computing (Euro-Par '23), 2023.
Abstract | Links | BibTeX | Tags: eupilot, icsc
@misc{23:europar:mafl,
title = {Model-Agnostic Federated Learning},
author = {Gianluca Mittone and Walter Riviera and Iacopo Colonnelli and Robert Birke and Marco Aldinucci},
url = {https://datacloud.di.unito.it/index.php/s/9T6G2tRreRomBAE},
year = {2023},
date = {2023-09-01},
address = {Limassol, Cyprus},
abstract = {Since its debut in 2016, Federated Learning (FL) has been tied to the inner workings of Deep Neural Networks (DNNs); this allowed its development as DNNs proliferated but neglected those scenarios in which using DNNs is not possible or advantageous. The fact that most current FL frameworks only support DNNs reinforces this problem. To address the lack of non-DNN-based FL solutions, we propose MAFL (Model-Agnostic Federated Learning). MAFL merges a model-agnostic FL algorithm, AdaBoost.F, with an open industry-grade FL framework: Intel® OpenFL. MAFL is the first FL system not tied to any machine learning model, allowing exploration of FL beyond DNNs. We test MAFL from multiple points of view, assessing its correctness, flexibility, and scaling properties up to 64 nodes of an HPC cluster. We also show how we optimised OpenFL achieving a 5.5x speedup over a standard FL scenario. MAFL is compatible with x86-64, ARM-v8, Power and RISC-V.},
howpublished = {29th International European Conference on Parallel and Distributed Computing (Euro-Par '23)},
keywords = {eupilot, icsc},
pubstate = {published},
tppubtype = {misc}
}
Iacopo Colonnelli
Workflows and the Common Workflow Language (CWL) Miscellaneous
OSA2Micro: An Open Science Approach to Microbiology data integration, 2023, (Invited talk).
Links | BibTeX | Tags: streamflow
@misc{23:SA2Micro,
title = {Workflows and the Common Workflow Language (CWL)},
author = {Iacopo Colonnelli},
url = {https://datacloud.di.unito.it/index.php/s/NHWWzMMaQgAsA52},
year = {2023},
date = {2023-05-01},
address = {Torino, Italy},
howpublished = {OSA2Micro: An Open Science Approach to Microbiology data integration},
note = {Invited talk},
keywords = {streamflow},
pubstate = {published},
tppubtype = {misc}
}
Iacopo Colonnelli
UNITO tools presentation Miscellaneous
CN HPC Flagship 3 Working Day, 2023.
Links | BibTeX | Tags: jupyter-workflow, streamflow
@misc{23:FL3WorkingDay,
title = {UNITO tools presentation},
author = {Iacopo Colonnelli},
url = {https://datacloud.di.unito.it/index.php/s/fgHbnLDQSFtcwLd},
year = {2023},
date = {2023-05-01},
address = {Bologna, Italy},
howpublished = {CN HPC Flagship 3 Working Day},
keywords = {jupyter-workflow, streamflow},
pubstate = {published},
tppubtype = {misc}
}
Gianluca Mittone, Nicolò Tonci, Robert Birke, Iacopo Colonnelli, Doriana Medić, Andrea Bartolini, Roberto Esposito, Emanuele Parisi, Francesco Beneventi, Mirko Polato, Massimo Torquati, Luca Benini, Marco Aldinucci
Experimenting with Emerging RISC-V Systems for Decentralised Machine Learning Miscellaneous
20th ACM international conference on computing frontiers (CF '23), 2023, (Invited talk).
Abstract | Links | BibTeX | Tags: eupilot, icsc
@misc{23:ACMCF,
title = {Experimenting with Emerging RISC-V Systems for Decentralised Machine Learning},
author = {Gianluca Mittone and Nicolò Tonci and Robert Birke and Iacopo Colonnelli and Doriana Medić and Andrea Bartolini and Roberto Esposito and Emanuele Parisi and Francesco Beneventi and Mirko Polato and Massimo Torquati and Luca Benini and Marco Aldinucci},
url = {https://datacloud.di.unito.it/index.php/s/BYyqZbHzzN4DL8Z},
year = {2023},
date = {2023-05-01},
abstract = {Decentralised Machine Learning (DML) enables collaborative machine learning without centralised input data. Federated Learning (FL) and Edge Inference are examples of DML. While tools for DML (especially FL) are starting to flourish, many are not flexible and portable enough to experiment with novel processors (e.g., RISC-V), non-fully connected network topologies, and asynchronous collaboration schemes. We overcome these limitations via a domain-specific language allowing us to map DML schemes to an underlying middleware, i.e. the FastFlow parallel programming library. We experiment with it by generating different working DML schemes on x86-64 and ARM platforms and an emerging RISC-V one. We characterise the performance and energy efficiency of the presented schemes and systems. As a byproduct, we introduce a RISC-V porting of the PyTorch framework, the first publicly available to our knowledge.},
howpublished = {20th ACM international conference on computing frontiers (CF '23)},
note = {Invited talk},
keywords = {eupilot, icsc},
pubstate = {published},
tppubtype = {misc}
}
Sofia Karvounari, Eleni Mathioulaki, Michael R. Crusoe, Iacopo Colonnelli
Standardised Workflows at EBRAINS Miscellaneous
Human Brain Project Summit 2023, 2023, (Invited talk).
Abstract | Links | BibTeX | Tags: across, eupex, space, streamflow
@misc{23:HBPSummit,
title = {Standardised Workflows at EBRAINS},
author = {Sofia Karvounari and Eleni Mathioulaki and Michael R. Crusoe and Iacopo Colonnelli},
url = {https://datacloud.di.unito.it/index.php/s/K5YQKTsX9N7NLT8},
year = {2023},
date = {2023-03-01},
address = {Marseille, France},
abstract = {A hands-on training offer for Standardised Workflows in EBRAINS. A short presentation will be used as an introduction, while the main hands-on session will provide information about Writing and Executing Standardised Workflows. TC will give some guidelines, so attendees can experiment with writing CWL tools and workflows and then they will be given access to VM to execute these workflows. The Workflows Dashboard will be also presented during the same session, offering to the attendees the opportunity to understand the different functionalities, use it with TC support and provide useful comments.},
howpublished = {Human Brain Project Summit 2023},
note = {Invited talk},
keywords = {across, eupex, space, streamflow},
pubstate = {published},
tppubtype = {misc}
}
Iacopo Colonnelli
CWL for HPC: are we there yet? Miscellaneous
2023 CWL Conference, 2023, (Invited talk).
Abstract | Links | BibTeX | Tags: across, eupex, streamflow
@misc{23:CWLConference,
title = {CWL for HPC: are we there yet?},
author = {Iacopo Colonnelli},
url = {https://datacloud.di.unito.it/index.php/s/CMCd5LiZeXsxwEg},
year = {2023},
date = {2023-03-01},
address = {Heidelberg, Germany},
abstract = {Modern HPC applications are becoming so heterogeneous and complex that a modular approach to their design, deployment and orchestration is now necessary. This talk explores the benefits of using a vendor-agnostic workflow language (CWL) coupled with a hybrid workflow management system (StreamFlow) in the HPC ecosystem. Also, it will examine the requirements needed to model HPC applications effectively, the CWL’s readiness to meet such requirements, and the proposals made to improve the language where needed. Four real use cases will drive the discussion: the ACROSS Project (G.A. n. 955648), where CWL is the primary interface to model three HPC workflows, and the EUPEX Project (G.A. n. 101033975), where StreamFlow will be used for the rapid prototyping of a seismic engineering HPC application for a Modular Supercomputing Architecture (MSA) system.},
howpublished = {2023 CWL Conference},
note = {Invited talk},
keywords = {across, eupex, streamflow},
pubstate = {published},
tppubtype = {misc}
}
2022
Iacopo Colonnelli, Marco Aldinucci
Hybrid Workflows For Large-Scale Scientific Applications Miscellaneous
6th EAGE High Performance Computing Workshop, 2022.
Abstract | Links | BibTeX | Tags: across, eupex, jupyter-workflow, textarossa
@misc{22:eage,
title = {Hybrid Workflows For Large-Scale Scientific Applications},
author = {Iacopo Colonnelli and Marco Aldinucci},
url = {https://datacloud.di.unito.it/index.php/s/GScPS5LCPdt6Yoo},
year = {2022},
date = {2022-09-01},
address = {Milano, Italy},
abstract = {Large-scale scientific applications are facing an irreversible transition from monolithic, high-performance oriented codes to modular and polyglot deployments of specialised (micro-)services. The reasons behind this transition are many: coupling of standard solvers with Deep Learning techniques, offloading of data analysis and visualisation to Cloud, and the advent of specialised hardware accelerators. Topology-aware Workflow Management Systems (WMSs) play a crucial role. In particular, topology-awareness allows an explicit mapping of workflow steps onto heterogeneous locations, allowing automated executions on top of hybrid architectures (e.g., cloud+HPC or classical+quantum). Plus, topology-aware WMSs can offer non-functional requirements OOTB, e.g. components’ life-cycle orchestration, secure and efficient data transfers, fault tolerance, and cross-cluster execution of urgent workloads. Augmenting interactive Jupyter Notebooks with distributed workflow capabilities allows domain experts to prototype and scale applications using the same technological stack, while relying on a feature-rich and user-friendly web interface. This abstract will showcase how these general methodologies can be applied to a typical geoscience simulation pipeline based on the Full Wavefront Inversion (FWI) technique. In particular, a prototypical Jupyter Notebook will be executed interactively on Cloud. Preliminary data analyses and post-processing will be executed locally, while the computationally demanding optimisation loop will be scheduled on a remote HPC cluster.},
howpublished = {6th EAGE High Performance Computing Workshop},
keywords = {across, eupex, jupyter-workflow, textarossa},
pubstate = {published},
tppubtype = {misc}
}
Iacopo Colonnelli, Barbara Cantalupo, Doriana Medić, Marco Aldinucci
Hybrid workflows for heterogeneous distributed computing Miscellaneous
3rd Italian Workshop on HPC (ITWSHPC), 2022.
Links | BibTeX | Tags: across, admire, eumaster4hpc, eupex, eupilot, textarossa
@misc{22:itwshpc,
title = {Hybrid workflows for heterogeneous distributed computing},
author = {Iacopo Colonnelli and Barbara Cantalupo and Doriana Medić and Marco Aldinucci},
url = {https://datacloud.di.unito.it/index.php/s/ienbcA2DJ26aioE},
year = {2022},
date = {2022-09-01},
address = {Torino, Italy},
howpublished = {3rd Italian Workshop on HPC (ITWSHPC)},
keywords = {across, admire, eumaster4hpc, eupex, eupilot, textarossa},
pubstate = {published},
tppubtype = {misc}
}
Iacopo Colonnelli, Marco Aldinucci
CINI HPC-KTT: HPC Key Technologies and Tools National Lab Miscellaneous
NVIDIA HPC Roundtable, 2022, (Invited talk).
Links | BibTeX | Tags: across, admire, eumaster4hpc, eupex, eupilot, textarossa
@misc{22:nvidia_hpc_roundtable,
title = {CINI HPC-KTT: HPC Key Technologies and Tools National Lab},
author = {Iacopo Colonnelli and Marco Aldinucci},
url = {https://datacloud.di.unito.it/index.php/s/9EQniZ2dGzdJ26f},
year = {2022},
date = {2022-09-01},
address = {Casalecchio di Reno, Italy},
howpublished = {NVIDIA HPC Roundtable},
note = {Invited talk},
keywords = {across, admire, eumaster4hpc, eupex, eupilot, textarossa},
pubstate = {published},
tppubtype = {misc}
}
Iacopo Colonnelli, Dario Tranchitella
Dossier: multi-tenant distributed Jupyter Notebooks Miscellaneous
DoK Talks 141, 2022, (Invited talk).
Abstract | Links | BibTeX | Tags: across, deephealth, hpc4ai, jupyter-workflow
@misc{22:data-on-kubernetes,
title = {Dossier: multi-tenant distributed Jupyter Notebooks},
author = {Iacopo Colonnelli and Dario Tranchitella},
url = {https://datacloud.di.unito.it/index.php/s/RNqTGmTqWS66qHT},
year = {2022},
date = {2022-07-01},
address = {Virtual event},
abstract = {When providing data analysis as a service, one must tackle several problems. Data privacy and protection by design are crucial when working on sensitive data. Performance and scalability are fundamental for compute-intensive workloads, e.g. training Deep Neural Networks. User-friendly interfaces and fast prototyping tools are essential to allow domain experts to experiment with new techniques. Portability and reproducibility are necessary to assess the actual value of results. Kubernetes is the best platform to provide reliable, elastic, and maintainable services. However, Kubernetes alone is not enough to achieve large-scale multi-tenant reproducible data analysis. OOTB support for multi-tenancy is too rough, with only two levels of segregation (i.e. the single namespace or the entire cluster). Offloading computation to off-cluster resources is non-trivial and requires the user's manual configuration. Also, Jupyter Notebooks per se cannot provide much scalability (they execute locally and sequentially) and reproducibility (users can run cells in any order and any number of times). The Dossier platform allows system administrators to manage multi-tenant distributed Jupyter Notebooks at the cluster level in the Kubernetes way, i.e. through CRDs. Namespaces are aggregated in Tenants, and all security and accountability aspects are managed at that level. Each Notebook spawns into a user-dedicated namespace, subject to all Tenant-level constraints. Users can rely on provisioned resources, either in-cluster worker nodes or external resources like HPC facilities. Plus, they can plug their computing nodes in a BYOD fashion. Notebooks are interpreted as distributed workflows, where each cell is a task that one can offload to a different location in charge of its execution.},
howpublished = {DoK Talks 141},
note = {Invited talk},
keywords = {across, deephealth, hpc4ai, jupyter-workflow},
pubstate = {published},
tppubtype = {misc}
}
Iacopo Colonnelli
StreamFlow Miscellaneous
2nd HealthyCloud Workshop: Analysis of existing orchestration mechanisms for distributed computational analyses, 2022, (Invited talk).
Links | BibTeX | Tags: across, deephealth, eupex, streamflow, textarossa
@misc{22:healthycloud-workshop,
title = {StreamFlow},
author = {Iacopo Colonnelli},
url = {https://datacloud.di.unito.it/index.php/s/Taz8qtzmkmn9ffT},
year = {2022},
date = {2022-07-01},
address = {Virtual event},
howpublished = {2nd HealthyCloud Workshop: Analysis of existing orchestration mechanisms for distributed computational analyses},
note = {Invited talk},
keywords = {across, deephealth, eupex, streamflow, textarossa},
pubstate = {published},
tppubtype = {misc}
}
Iacopo Colonnelli, Marco Aldinucci
T4.1: Streaming models Miscellaneous
TEXTAROSSA General Meeting, 2022.
Links | BibTeX | Tags: textarossa
@misc{22:textarossa-ga-meeting,
title = {T4.1: Streaming models},
author = {Iacopo Colonnelli and Marco Aldinucci},
url = {https://datacloud.di.unito.it/index.php/s/cNBnwSnTc8GiCkN},
year = {2022},
date = {2022-06-01},
address = {Roma, Italy},
howpublished = {TEXTAROSSA General Meeting},
keywords = {textarossa},
pubstate = {published},
tppubtype = {misc}
}
Iacopo Colonnelli
StreamFlow: a topology-aware WMS Miscellaneous
ELIXIR Cloud, Data & AAI Bi-weekly Technical Calls, 2022, (Invited talk).
Links | BibTeX | Tags: across, deephealth, eupex, streamflow, textarossa
@misc{22:elixir-streamflow,
title = {StreamFlow: a topology-aware WMS},
author = {Iacopo Colonnelli},
url = {https://datacloud.di.unito.it/index.php/s/Z9GsKnRCxmBdMd3},
year = {2022},
date = {2022-06-01},
address = {Virtual event},
howpublished = {ELIXIR Cloud, Data & AAI Bi-weekly Technical Calls},
note = {Invited talk},
keywords = {across, deephealth, eupex, streamflow, textarossa},
pubstate = {published},
tppubtype = {misc}
}
Iacopo Colonnelli
StreamFlow: A framework for hybrid workflows Miscellaneous
EUPEX WP5 bi-weekly meeting, 2022.
Links | BibTeX | Tags: eupex, streamflow
@misc{22:eupex-streamflow,
title = {StreamFlow: A framework for hybrid workflows},
author = {Iacopo Colonnelli},
url = {https://datacloud.di.unito.it/index.php/s/NjKEySP7HfrCQHZ},
year = {2022},
date = {2022-04-01},
address = {Virtual event},
howpublished = {EUPEX WP5 bi-weekly meeting},
keywords = {eupex, streamflow},
pubstate = {published},
tppubtype = {misc}
}
Iacopo Colonnelli, Dario Tranchitella
OpenDeepHealth: Crafting a Deep Learning Platform as a Service with Kubernetes Miscellaneous
J on The Beach 2022, 2022.
Links | BibTeX | Tags: across, deephealth, hpc4ai, jupyter-workflow, streamflow
@misc{22:jotb22,
title = {OpenDeepHealth: Crafting a Deep Learning Platform as a Service with Kubernetes},
author = {Iacopo Colonnelli and Dario Tranchitella},
url = {https://datacloud.di.unito.it/index.php/s/n6J7STNnwdyqtET},
year = {2022},
date = {2022-04-01},
address = {Malaga, Spain},
howpublished = {J on The Beach 2022},
keywords = {across, deephealth, hpc4ai, jupyter-workflow, streamflow},
pubstate = {published},
tppubtype = {misc}
}
Iacopo Colonnelli
Distributed workflows with Jupyter Miscellaneous
J on The Beach 2022, 2022, (Workshop).
Links | BibTeX | Tags: across, deephealth, jupyter-workflow, streamflow
@misc{22:jotb22-workshop,
title = {Distributed workflows with Jupyter},
author = {Iacopo Colonnelli},
url = {https://datacloud.di.unito.it/index.php/s/om89q55S6ePf2Ji},
year = {2022},
date = {2022-04-01},
address = {Malaga, Spain},
howpublished = {J on The Beach 2022},
note = {Workshop},
keywords = {across, deephealth, jupyter-workflow, streamflow},
pubstate = {published},
tppubtype = {misc}
}
Iacopo Colonnelli
StreamFlow: A framework for hybrid workflows Miscellaneous
ACROSS WP4 meeting, 2022.
Links | BibTeX | Tags: across, streamflow
@misc{22:across-streamflow,
title = {StreamFlow: A framework for hybrid workflows},
author = {Iacopo Colonnelli},
url = {https://datacloud.di.unito.it/index.php/s/FXFTKtQSRf6anMX},
year = {2022},
date = {2022-02-01},
address = {Virtual event},
howpublished = {ACROSS WP4 meeting},
keywords = {across, streamflow},
pubstate = {published},
tppubtype = {misc}
}
Iacopo Colonnelli
The OpenDeepHealth toolkit Miscellaneous
DeepHealth Winter School, 2022.
Links | BibTeX | Tags: deephealth, hpc4ai
@misc{22:DHWinterSchool,
title = {The OpenDeepHealth toolkit},
author = {Iacopo Colonnelli},
url = {https://datacloud.di.unito.it/index.php/s/cJ8pRNsWRrfwPqr},
year = {2022},
date = {2022-01-01},
address = {Torino, Italy},
howpublished = {DeepHealth Winter School},
keywords = {deephealth, hpc4ai},
pubstate = {published},
tppubtype = {misc}
}
2021
Iacopo Colonnelli
StreamFlow: A framework for hybrid workflows Miscellaneous
ACROSS WP4 meeting, 2021.
Links | BibTeX | Tags: across, streamflow
@misc{21:across-streamflow,
title = {StreamFlow: A framework for hybrid workflows},
author = {Iacopo Colonnelli},
url = {https://datacloud.di.unito.it/index.php/s/yrGYJL6CyNywF8a},
year = {2021},
date = {2021-10-01},
address = {Virtual event},
howpublished = {ACROSS WP4 meeting},
keywords = {across, streamflow},
pubstate = {published},
tppubtype = {misc}
}
Iacopo Colonnelli
HPC Containers Miscellaneous
ACROSS WP4 meeting, 2021.
@misc{21:across-containers,
title = {HPC Containers},
author = {Iacopo Colonnelli},
url = {https://datacloud.di.unito.it/index.php/s/ddf3YBjpm8KBGAF},
year = {2021},
date = {2021-07-01},
address = {Virtual event},
howpublished = {ACROSS WP4 meeting},
keywords = {across},
pubstate = {published},
tppubtype = {misc}
}
Marco Aldinucci, Iacopo Colonnelli
The Universal Cloud-HPC Pipeline for the AI-Assisted Explainable Diagnosis of COVID-19 Pneumonia Miscellaneous
NVidia GTC'21, 2021, (Invited talk).
Abstract | Links | BibTeX | Tags: deephealth, hpc4ai, streamflow
@misc{21:gtc:clairecovid,
title = {The Universal Cloud-HPC Pipeline for the AI-Assisted Explainable Diagnosis of COVID-19 Pneumonia},
author = {Marco Aldinucci and Iacopo Colonnelli},
url = {https://datacloud.di.unito.it/index.php/s/AkQLbPpEEtDzbbm},
year = {2021},
date = {2021-04-01},
address = {Virtual event},
abstract = {We'll present a methodology to run DNN pipelines on hybrid cloud+HPC infrastructure. We'll also define a "universal pipeline" for medical images. The pipeline can reproduce all state-of-the-art DNNs to diagnose COVID-19 pneumonia, which appeared in the literature during the first Italian lockdown and following months. We can run all of them (across cloud+HPC platforms) and compare their performance in terms of sensitivity and specificity to set a baseline to evaluate future progress in the automated diagnosis of COVID-19. Also, the pipeline makes existing DNNs explainable by way of adversarial training. The pipeline is easily portable and can run across different infrastructures, adapting the performance-urgency trade-off. The methodology builds onto two novel software programs: the streamflow workflow system and the AI-sandbox concept (parallel container with user-space encrypted file system). We reach over 92% accuracy in diagnosing COVID pneumonia.},
howpublished = {NVidia GTC'21},
note = {Invited talk},
keywords = {deephealth, hpc4ai, streamflow},
pubstate = {published},
tppubtype = {misc}
}
Iacopo Colonnelli
StreamFlow: cross breeding cloud with HPC Miscellaneous
2021 CWL Mini Conference, 2021, (Invited talk).
Abstract | Links | BibTeX | Tags: deephealth, streamflow
@misc{21:CWLMiniConference,
title = {StreamFlow: cross breeding cloud with HPC},
author = {Iacopo Colonnelli},
url = {https://datacloud.di.unito.it/index.php/s/Le9gg4PfjRxBwXD},
year = {2021},
date = {2021-02-01},
address = {Virtual event},
abstract = {Workflows are among the most commonly used tools in a variety of execution environments. Many of them target a specific environment; few of them make it possible to execute an entire workflow in different environments, e.g. Kubernetes and batch clusters. We present a novel approach to workflow execution, called StreamFlow, that complements the workflow graph with the declarative description of potentially complex execution environments, and that makes it possible the execution onto multiple sites not sharing a common data space.},
howpublished = {2021 CWL Mini Conference},
note = {Invited talk},
keywords = {deephealth, streamflow},
pubstate = {published},
tppubtype = {misc}
}
2020
Iacopo Colonnelli, Sergio Rabellino
JupyterFlow: Jupyter Notebooks su larga scala Miscellaneous
Workshop GARR 2020, 2020.
Abstract | Links | BibTeX | Tags: deephealth, hpc4ai, jupyter-workflow
@misc{20:GarrWorkshop,
title = {JupyterFlow: Jupyter Notebooks su larga scala},
author = {Iacopo Colonnelli and Sergio Rabellino},
url = {https://datacloud.di.unito.it/index.php/s/ASPEmyXAj5QscgC},
year = {2020},
date = {2020-11-01},
address = {Virtual event},
abstract = {I Jupyter Notebook sono largamente utilizzati sia in ambito industriale che accademico come strumento di didattica, prototipazione e analisi esplorative. Purtroppo il sistema runtime standard di Jupyter non è abbastanza potente per sostenere un carichi di lavoro reali e spesso l'unica soluzione è quella di riscrivere il codice da zero in una tecnologia con supporto HPC. Intrgrando lo stack Jupyter con StreamFlow (https://streamflow.di.unito.it/) è possibile creare i Notebook tramite un'interfaccia web su cloud ed eseguirli in maniera trasparente in remoto su una VM con GPU o su nodi HPC.},
howpublished = {Workshop GARR 2020},
keywords = {deephealth, hpc4ai, jupyter-workflow},
pubstate = {published},
tppubtype = {misc}
}
Iacopo Colonnelli
StreamFlow: cross breeding cloud with HPC Miscellaneous
HPC-Europa3 2nd Transnational Access Meeting (TAM), 2020, (Invited talk).
Abstract | Links | BibTeX | Tags: streamflow
@misc{20:HPCEuropa3TAM,
title = {StreamFlow: cross breeding cloud with HPC},
author = {Iacopo Colonnelli},
url = {https://datacloud.di.unito.it/index.php/s/qPHHrSNxk8QXJDw},
year = {2020},
date = {2020-10-01},
address = {Virtual event},
abstract = {Workflows are among the most commonly used tools in a variety of execution environments. Many of them target a specific environment; few of them make it possible to execute an entire workflow in different environments, e.g. Kubernetes and batch clusters. We present a novel approach to workflow execution, called StreamFlow, that complements the workflow graph with the declarative description of potentially complex execution environments, and that makes it possible the execution onto multiple sites not sharing a common data space. StreamFlow is then exemplified on a novel bioinformatics pipeline for single-cell transcriptomic data analysis workflow.},
howpublished = {HPC-Europa3 2nd Transnational Access Meeting (TAM)},
note = {Invited talk},
keywords = {streamflow},
pubstate = {published},
tppubtype = {misc}
}
2019
Iacopo Colonnelli
StreamFlow: un approccio dichiarativo a workflow e pipeline di micro-servizi Miscellaneous
Workshop GARR 2019, 2019.
Abstract | Links | BibTeX | Tags: streamflow
@misc{19:GarrWorkshop,
title = {StreamFlow: un approccio dichiarativo a workflow e pipeline di micro-servizi},
author = {Iacopo Colonnelli},
url = {https://datacloud.di.unito.it/index.php/s/kZqyiQnBEQNdXJe},
year = {2019},
date = {2019-10-01},
address = {Roma, Italy},
abstract = {Negli ultimi anni, gli approcci orientati ai container si sono dimostrati particolarmente efficaci nel garantire portabilità e riproducibilità dei workflow scientifici. Tuttavia, con il continuo aumento del volume di dati a disposizione e la crescente complessità delle procedure di analisi in ogni campo della ricerca, anche i requisiti di performance e riusabilità si fanno via via sempre più essenziali. L'obiettivo principale di StreamFlow è quello di fornire un nuovo paradigma, totalmente dichiarativo, per la descrizione e l'accelerazione di workflow scientifici in ambienti distribuiti. La peculiarità di StreamFlow risiede nel fatto che l'ambiente di esecuzione è interamente descritto in termini di servizi (container), connessioni tra essi e fattori di replica. Inoltre, ogni task del workflow è esplicitamente mappato sulla tipologia di servizio richiesta. Questo permette un maggior controllo sull'utilizzo delle risorse e politiche di scheduling più precise, a vantaggio delle performance. I principali vantaggi di un approccio dichiarativo sono invece la più facile comprensione ed estensione dei modelli esistenti, a vantaggio della riusabilitià.},
howpublished = {Workshop GARR 2019},
keywords = {streamflow},
pubstate = {published},
tppubtype = {misc}
}
Iacopo Colonnelli
Deep Learning at Scale Miscellaneous
27th Euromicro International Conference on Parallel, Distributed and Network-based Processing (PDP 2019), 2019.
Abstract | Links | BibTeX | Tags:
@misc{19:PDPNNT,
title = {Deep Learning at Scale},
author = {Iacopo Colonnelli},
url = {https://datacloud.di.unito.it/index.php/s/nRW9M69C3AtpDoM},
year = {2019},
date = {2019-02-01},
publisher = {IEEE},
address = {Pavia, Italy},
abstract = {This work presents a novel approach to distributed training of deep neural networks (DNNs) that aims to overcome the issues related to mainstream approaches to data parallel training. Established techniques for data parallel training are discussed from both a parallel computing and deep learning perspective, then a different approach is presented that is meant to allow DNN training to scale while retaining good convergence properties. Moreover, an experimental implementation is presented as well as some preliminary results.},
howpublished = {27th Euromicro International Conference on Parallel, Distributed and Network-based Processing (PDP 2019)},
keywords = {},
pubstate = {published},
tppubtype = {misc}
}
Iacopo Colonnelli
Accelerating spectral graph analysis through wavefronts of linear algebra operations Miscellaneous
27th Euromicro International Conference on Parallel, Distributed and Network-based Processing (PDP 2019), 2019.
Abstract | Links | BibTeX | Tags:
@misc{19:PDPArmadillo,
title = {Accelerating spectral graph analysis through wavefronts of linear algebra operations},
author = {Iacopo Colonnelli},
url = {https://datacloud.di.unito.it/index.php/s/zK4eSzdsdB8CfQX},
year = {2019},
date = {2019-02-01},
publisher = {IEEE},
address = {Pavia, Italy},
abstract = {The wavefront pattern captures the unfolding of a parallel computation in which data elements are laid out as a logical multidimensional grid and the dependency graph favours a diagonal sweep across the grid. In the emerging area of spectral graph analysis, the computing often consists in a wavefront running over a tiled matrix, involving expensive linear algebra kernels. While these applications might benefit from parallel heterogeneous platforms (multi-core with GPUs),programming wavefront applications directly with high-performance linear algebra libraries yields code that is complex to write and optimize for the specific application. We advocate a methodology based on two abstractions (linear algebra and parallel pattern-based run-time), that allows to develop portable, self-configuring, and easy-to-profile code on hybrid platforms.},
howpublished = {27th Euromicro International Conference on Parallel, Distributed and Network-based Processing (PDP 2019)},
keywords = {},
pubstate = {published},
tppubtype = {misc}
}