Gianluca Mittone
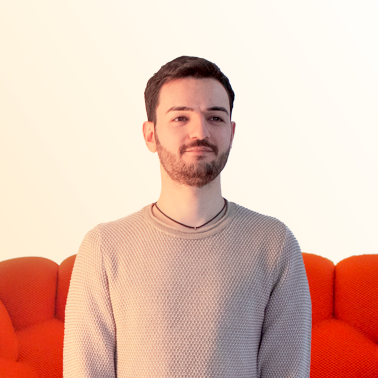
Gianluca Mittone – PhD Candidate
Computer Science Department, University of Turin, Italy
Parallel Computing group
Via Pessinetto 12, 10149 Torino – Italy
Email: gianluca.mittone@unito.it
Short Bio
Gianluca Mittone received his Bachelor’s Degree in Computer Science in 2017 from the University of Turin, Italy, with a thesis on the handling of exceptions in Description Logics, developed under the supervision of Professor Gian Luca Pozzato. He proposed the implementation of an algorithm for the automatic revision of ontologies exploiting a Typicality operator. Then, Gianluca also received a Master‘s Degree in Computer Science in 2019 from the University of Turin, Italy, under the supervision of Professor Marco Aldinucci. He proposed a master’s thesis on a novel distributed approach for deep learning named NNT (Nearest Neighbours Training), which uses a locally synchronous approach to achieve a better trade-off between computational time and learning results.
Subsequently, he spent eight months as a research engineer at the Computer Science Department of the University of Turin, Italy, working on different projects involving HPC and Machine Learning techniques. Notably, he played a crucial role in developing the PRAISE Score, a cardiological, ML-based risk score included in the European Society of Cardiology’s guidelines for the Acute coronary syndrome treatment.
He then became a PhD student in Modeling and Data Science in 2020 at the same university. His work focuses on the Federated Learning methodology and its extensions. Some example of his research are OpenFL-x, an open-source extension of Intel’s OpenFL allowing to build federation using any ML model, and FastFL, and open-source, HPC-oriented FL framework allowing customisable communication topologies. He is now actively working on LLM-oriented FL techniques, such as xFFL.
Gianluca Mittone is currently a PhD candidate and has been a member of the Parallel Computing group alpha since 2020.
Fields of interest:
- High-Performance Computing (HPC)
- Deep learning
- Machine Learning
- Federated Learning
- RISC-V
- SGX
Publications
2024
Gianluca Mittone, Giulio Malenza, Marco Aldinucci, Robert Birke
Distributed Edge Inference: an Experimental Study on Multiview Detection Proceedings Article
In: Proc. of the 16th IEEE/ACM Intl. Conference on Utility and Cloud Computing Companion (UCC), pp. 1-6, ACM, Taormina, Italy, 2024, (eupilot, icsc).
Abstract | Links | BibTeX | Tags: ai, eupilot, icsc
@inproceedings{23:mittone:multiview,
title = {Distributed Edge Inference: an Experimental Study on Multiview Detection},
author = {Gianluca Mittone and Giulio Malenza and Marco Aldinucci and Robert Birke},
url = {https://iris.unito.it/handle/2318/1950083},
doi = {10.1145/3603166.3632561},
year = {2024},
date = {2024-12-01},
booktitle = {Proc. of the 16th IEEE/ACM Intl. Conference on Utility and Cloud Computing Companion (UCC)},
volume = {30},
pages = {1-6},
publisher = {ACM},
address = {Taormina, Italy},
institution = {Computer Science Department, University of Torino},
abstract = {Computing is evolving rapidly to cater to the increasing demand for sophisticated services, and Cloud computing lays a solid foundation for flexible on-demand provisioning. However, as the size of applications grows, the centralised client-server approach used by Cloud computing increasingly limits the applications' scalability. To achieve ultra-scalability, cloud/edge/fog computing converges into the compute continuum, completely decentralising the infrastructure to encompass universal, pervasive resources. The compute continuum makes devising applications benefitting from this complex environment a challenging research problem. We put the opportunities the compute continuum others to the test through a real-world multi-view detection model (MvDet) implemented with the FastFL C/C++ high-performance edge inference framework. Computational performance is discussed considering many experimental scenarios, encompassing different edge computational capabilities and network bandwidths. We obtain up to 1.92x speedup in inference time over a centralised solution using the same devices.},
note = {eupilot, icsc},
keywords = {ai, eupilot, icsc},
pubstate = {published},
tppubtype = {inproceedings}
}
Bruno Casella, Iacopo Colonnelli, Gianluca Mittone, Robert Birke, Walter Riviera, Antonio Sciarappa, Carlo Cavazzoni, Marco Aldinucci
A Performance Analysis for Confidential Federated Learning Proceedings Article
In: Proceedings of the 2024 Deep Learning Security and Privacy Workshop, IEEE Symposium on Security and Privacy 2024, San Francisco, CA, 2024.
Abstract | Links | BibTeX | Tags: ai, confidential, epi, icsc
@inproceedings{24:casella:sgx,
title = {A Performance Analysis for Confidential Federated Learning},
author = {Bruno Casella and Iacopo Colonnelli and Gianluca Mittone and Robert Birke and Walter Riviera and Antonio Sciarappa and Carlo Cavazzoni and Marco Aldinucci},
url = {https://iris.unito.it/retrieve/b5877a97-2d8d-4e95-8791-0aa4a1b953b3/DLSP___CONFIDENTIAL_FL.pdf},
doi = {10.1109/SPW63631.2024.00009},
year = {2024},
date = {2024-05-01},
booktitle = {Proceedings of the 2024 Deep Learning Security and Privacy Workshop, IEEE Symposium on Security and Privacy 2024},
address = {San Francisco, CA},
abstract = {Federated Learning (FL) has emerged as a solution to preserve data privacy by keeping the data locally on each participant's device. However, FL alone is still vulnerable to attacks that can cause privacy leaks. Therefore, it becomes necessary to take additional security measures at the cost of increasing runtimes. The Trusted Execution Environment (TEE) approach promises to offer the highest degree of security during execution. However, TEEs suffer from memory limits which prevent safe end-to-end FL training of modern deep models. State-of- the-art approaches limit secure training to selected layers, failing to avert the full spectrum of attacks or adopt layer-wise training affecting model performance. We benchmark the usage of a library OS (LibOS) to run the full, unmodified end-to-end FL training inside the TEE. We extensively evaluate and model the overhead of the different security mechanisms needed to protect the data and model during computation (TEE), communication (TLS), and storage (disk encryption). The obtained results across three datasets and two models demonstrate that LibOSes are a viable way to seamlessly inject security into FL with limited overhead (at most 2x), offering valuable guidance for researchers and developers aiming to apply FL in data-security-focused contexts.},
keywords = {ai, confidential, epi, icsc},
pubstate = {published},
tppubtype = {inproceedings}
}
Oussama Harrak, Bruno Casella, Samuele Fonio, Piero Fariselli, Gianluca Mittone, Tiziana Sanavia, Marco Aldinucci
Federated AdaBoost for Survival Analysis Proceedings Article
In: Proceedings of the ECML-PKDD Workshop, 2nd workshop on advancements in Federated Learning, Vilnius, Lithuania, 2024.
Abstract | BibTeX | Tags: epi, icsc
@inproceedings{harrak2024fedsurvboost,
title = {Federated AdaBoost for Survival Analysis},
author = {Oussama Harrak and Bruno Casella and Samuele Fonio and Piero Fariselli and Gianluca Mittone and Tiziana Sanavia and Marco Aldinucci},
year = {2024},
date = {2024-01-01},
booktitle = {Proceedings of the ECML-PKDD Workshop, 2nd workshop on advancements in Federated Learning},
address = {Vilnius, Lithuania},
abstract = {This work proposes FedSurvBoost, a federated learning pipeline for survival analysis based on the AdaBoost.F algorithm, which iteratively aggregates the best local weak hypotheses. Our method extends AdaBoost.F by removing the dependence on the number of classes coefficient from the computation of the weights of the best model. This makes it suitable for regression tasks, such as survival analysis. We show the effectiveness of our approach by comparing it with state-of-the-art methods, specifically developed for survival analysis problems, on two common survival datasets. Our code is available at https://github.com/oussamaHarrak/FedSurvBoost.},
keywords = {epi, icsc},
pubstate = {published},
tppubtype = {inproceedings}
}
Iacopo Colonnelli, Robert Birke, Giulio Malenza, Gianluca Mittone, Alberto Mulone, Jeroen Galjaard, Lydia Y. Chen, Sanzio Bassini, Gabriella Scipione, Jan Martinovič, Vit Vondrák, Marco Aldinucci
Cross-Facility Federated Learning Journal Article
In: Procedia Computer Science, vol. 240, pp. 3–12, 2024, ISSN: 1877-0509.
Abstract | Links | BibTeX | Tags: icsc, space, streamflow
@article{24:eurohpc:xffl,
title = {Cross-Facility Federated Learning},
author = {Iacopo Colonnelli and Robert Birke and Giulio Malenza and Gianluca Mittone and Alberto Mulone and Jeroen Galjaard and Lydia Y. Chen and Sanzio Bassini and Gabriella Scipione and Jan Martinovič and Vit Vondrák and Marco Aldinucci},
url = {https://www.sciencedirect.com/science/article/pii/S1877050924016909},
doi = {10.1016/j.procs.2024.07.003},
issn = {1877-0509},
year = {2024},
date = {2024-01-01},
booktitle = {Proceedings of the First EuroHPC user day},
journal = {Procedia Computer Science},
volume = {240},
pages = {3–12},
publisher = {Elsevier},
address = {Bruxelles, Belgium},
abstract = {In a decade, AI frontier research transitioned from the researcher's workstation to thousands of high-end hardware-accelerated compute nodes. This rapid evolution shows no signs of slowing down in the foreseeable future. While top cloud providers may be able to keep pace with this growth rate, obtaining and efficiently exploiting computing resources at that scale is a daunting challenge for universities and SMEs. This work introduces the Cross-Facility Federated Learning (XFFL) framework to bridge this compute divide, extending the opportunity to efficiently exploit multiple independent data centres for extreme-scale deep learning tasks to data scientists and domain experts. XFFL relies on hybrid workflow abstractions to decouple tasks from environment-specific technicalities, reducing complexity and enhancing reusability. In addition, Federated Learning (FL) algorithms eliminate the need to move large amounts of data between different facilities, reducing time-to-solution and preserving data privacy. The XFFL approach is empirically evaluated by training a full LLaMAv2 7B instance on two facilities of the EuroHPC JU, showing how the increased computing power completely compensates for the additional overhead introduced by two data centres.},
keywords = {icsc, space, streamflow},
pubstate = {published},
tppubtype = {article}
}
2023
Gianluca Mittone, Samuele Fonio
Benchmarking Federated Learning Scalability Proceedings Article
In: Proceedings of the 2nd Italian Conference on Big Data and Data Science, ITADATA 2023, September 11-13, 2023, CEUR, Naples, Italy, 2023.
Abstract | Links | BibTeX | Tags: eupilot, HPC, icsc
@inproceedings{23:itadata:extabstract:mittone:fonio,
title = {Benchmarking Federated Learning Scalability},
author = {Gianluca Mittone and Samuele Fonio},
url = {https://hdl.handle.net/2318/1933852},
year = {2023},
date = {2023-09-01},
booktitle = {Proceedings of the 2nd Italian Conference on Big Data and Data Science, ITADATA 2023, September 11-13, 2023},
publisher = {CEUR},
address = {Naples, Italy},
abstract = {Federated Learning (FL) is a widespread Machine Learning paradigm handling distributed Big Data. In this work, we demonstrate that different FL frameworks expose different scaling performances despite adopting the same technologies, highlighting the need for a more comprehensive study on the topic.},
keywords = {eupilot, HPC, icsc},
pubstate = {published},
tppubtype = {inproceedings}
}
Gianluca Mittone, Walter Riviera, Iacopo Colonnelli, Robert Birke, Marco Aldinucci
Model-Agnostic Federated Learning Proceedings Article
In: Euro-Par 2023: Parallel Processing, pp. 383–396, Springer, Limassol, Cyprus, 2023.
Abstract | Links | BibTeX | Tags: ai, confidential, eupilot, icsc, riscv
@inproceedings{23:mittone:mafl,
title = {Model-Agnostic Federated Learning},
author = {Gianluca Mittone and Walter Riviera and Iacopo Colonnelli and Robert Birke and Marco Aldinucci},
url = {https://doi.org/10.1007/978-3-031-39698-4_26},
doi = {10.1007/978-3-031-39698-4_26},
year = {2023},
date = {2023-08-01},
booktitle = {Euro-Par 2023: Parallel Processing},
volume = {14100},
pages = {383–396},
publisher = {Springer},
address = {Limassol, Cyprus},
institution = {Computer Science Department, University of Torino},
abstract = {Since its debut in 2016, Federated Learning (FL) has been tied to the inner workings of Deep Neural Networks (DNNs). On the one hand, this allowed its development and widespread use as DNNs proliferated. On the other hand, it neglected all those scenarios in which using DNNs is not possible or advantageous. The fact that most current FL frameworks only allow training DNNs reinforces this problem. To address the lack of FL solutions for non-DNN-based use cases, we propose MAFL (Model-Agnostic Federated Learning). MAFL marries a model-agnostic FL algorithm, AdaBoost.F, with an open industry-grade FL framework: Intel OpenFL. MAFL is the first FL system not tied to any specific type of machine learning model, allowing exploration of FL scenarios beyond DNNs and trees. We test MAFL from multiple points of view, assessing its correctness, flexibility and scaling properties up to 64 nodes. We optimised the base software achieving a 5.5x speedup on a standard FL scenario. MAFL is compatible with x86-64, ARM-v8, Power and RISC-V.},
keywords = {ai, confidential, eupilot, icsc, riscv},
pubstate = {published},
tppubtype = {inproceedings}
}
Gianluca Mittone, Nicolò Tonci, Robert Birke, Iacopo Colonnelli, Doriana Medić, Andrea Bartolini, Roberto Esposito, Emanuele Parisi, Francesco Beneventi, Mirko Polato, Massimo Torquati, Luca Benini, Marco Aldinucci
Experimenting with Emerging RISC-V Systems for Decentralised Machine Learning Proceedings Article
In: 20th ACM International Conference on Computing Frontiers (CF '23), ACM, Bologna, Italy, 2023, ISBN: 979-8-4007-0140-5/23/05, (https://arxiv.org/abs/2302.07946).
Abstract | Links | BibTeX | Tags: ai, confidential, eupilot, HPC, icsc, riscv
@inproceedings{23:mittone:fl-riscv,
title = {Experimenting with Emerging RISC-V Systems for Decentralised Machine Learning},
author = {Gianluca Mittone and Nicolò Tonci and Robert Birke and Iacopo Colonnelli and Doriana Medić and Andrea Bartolini and Roberto Esposito and Emanuele Parisi and Francesco Beneventi and Mirko Polato and Massimo Torquati and Luca Benini and Marco Aldinucci},
url = {https://dl.acm.org/doi/pdf/10.1145/3587135.3592211},
doi = {10.1145/3587135.3592211},
isbn = {979-8-4007-0140-5/23/05},
year = {2023},
date = {2023-05-01},
booktitle = {20th ACM International Conference on Computing Frontiers (CF '23)},
publisher = {ACM},
address = {Bologna, Italy},
institution = {Computer Science Department, University of Torino},
abstract = {Decentralised Machine Learning (DML) enables collaborative machine learning without centralised input data. Federated Learning (FL) and Edge Inference are examples of DML. While tools for DML (especially FL) are starting to flourish, many are not flexible and portable enough to experiment with novel systems (e.g., RISC-V), non-fully connected topologies, and asynchronous collaboration schemes. We overcome these limitations via a domain-specific language allowing to map DML schemes to an underlying middleware, i.e. the FastFlow parallel programming library. We experiment with it by generating different working DML schemes on two emerging architectures (ARM-v8, RISC-V) and the x86-64 platform. We characterise the performance and energy efficiency of the presented schemes and systems. As a byproduct, we introduce a RISC-V porting of the PyTorch framework, the first publicly available to our knowledge.},
note = {https://arxiv.org/abs/2302.07946},
keywords = {ai, confidential, eupilot, HPC, icsc, riscv},
pubstate = {published},
tppubtype = {inproceedings}
}
Gianluca Mittone, Filip Svoboda, Marco Aldinucci, Nicholas D. Lane, Pietro Lio
A Federated Learning Benchmark for Drug-Target Interaction Proceedings Article
In: Companion Proceedings of the ACM Web Conference 2023 (WWW '23 Companion), ACM, Austin, Texas, 2023, ISBN: 978-1-4503-9419-2/23/04, (https://arxiv.org/abs/2302.07684).
Abstract | Links | BibTeX | Tags: ai, confidential, eupilot, icsc
@inproceedings{23:mittone:dti,
title = {A Federated Learning Benchmark for Drug-Target Interaction},
author = {Gianluca Mittone and Filip Svoboda and Marco Aldinucci and Nicholas D. Lane and Pietro Lio},
url = {https://hdl.handle.net/2318/1898472},
doi = {10.1145/3543873.3587687},
isbn = {978-1-4503-9419-2/23/04},
year = {2023},
date = {2023-04-01},
booktitle = {Companion Proceedings of the ACM Web Conference 2023 (WWW '23 Companion)},
publisher = {ACM},
address = {Austin, Texas},
institution = {Computer Science Department, University of Torino},
abstract = {Aggregating pharmaceutical data in the drug-target interaction (DTI) domain has the potential to deliver life-saving breakthroughs. It is, however, notoriously difficult due to regulatory constraints and commercial interests. This work proposes the application of federated learning, which we argue to be reconcilable with the industry's constraints, as it does not require sharing of any information that would reveal the entities' data or any other high-level summary of it. When used on a representative GraphDTA model and the KIBA dataset it achieves up to 15 percent improved performance relative to the best available non-privacy preserving alternative. Our extensive battery of experiments shows that, unlike in other domains, the non-IID data distribution in the DTI datasets does not deteriorate FL performance. Additionally, we identify a material trade-off between the benefits of adding new data, and the cost of adding more clients.},
note = {https://arxiv.org/abs/2302.07684},
keywords = {ai, confidential, eupilot, icsc},
pubstate = {published},
tppubtype = {inproceedings}
}
William Fornaciari, Federico Reghenzani, Federico Terraneo, Davide Baroffio, Cecilia Metra, Martin Omana, Josie E. Rodriguez Condia, Matteo Sonza Reorda, Robert Birke, Iacopo Colonnelli, Gianluca Mittone, Marco Aldinucci, Gabriele Mencagli, Francesco Iannone, Filippo Palombi, Giuseppe Zummo, Daniele Cesarini, Federico Tesser
RISC-V-based Platforms for HPC: Analyzing Non-functional Properties for Future HPC and Big-Data Clusters Proceedings Article
In: Embedded Computer Systems: Architectures, Modeling, and Simulation - 23rd International Conference, SAMOS 2023, Samos, Greece, 2023, (icsc).
Abstract | Links | BibTeX | Tags: icsc, riscv
@inproceedings{23:SAMOS,
title = {RISC-V-based Platforms for HPC: Analyzing Non-functional Properties for Future HPC and Big-Data Clusters},
author = {William Fornaciari and Federico Reghenzani and Federico Terraneo and Davide Baroffio and Cecilia Metra and Martin Omana and Josie E. Rodriguez Condia and Matteo Sonza Reorda and Robert Birke and Iacopo Colonnelli and Gianluca Mittone and Marco Aldinucci and Gabriele Mencagli and Francesco Iannone and Filippo Palombi and Giuseppe Zummo and Daniele Cesarini and Federico Tesser},
url = {https://iris.unito.it/retrieve/b627eab0-3aa1-4fd7-8685-f47c62c792b3/SAMOS_2023_CN_HPC_FL1.pdf},
doi = {10.1007/978-3-031-46077-7_26},
year = {2023},
date = {2023-01-01},
booktitle = {Embedded Computer Systems: Architectures, Modeling, and Simulation - 23rd International Conference, SAMOS 2023},
address = {Samos, Greece},
abstract = {High-PerformanceComputing(HPC)haveevolvedtobeused to perform simulations of systems where physical experimentation is pro- hibitively impractical, expensive, or dangerous. This paper provides a general overview and showcases the analysis of non-functional properties in RISC-V-based platforms for HPCs. In particular, our analyses target the evaluation of power and energy control, thermal management, and reliability assessment of promising systems, structures, and technologies devised for current and future generation of HPC machines. The main set of design methodologies and technologies developed within the activ- ities of the Future and HPC & Big Data spoke of the National Centre of HPC, Big Data and Quantum Computing project are described along with the description of the testbed for experimenting two-phase cooling approaches.},
note = {icsc},
keywords = {icsc, riscv},
pubstate = {published},
tppubtype = {inproceedings}
}
Iacopo Colonnelli, Bruno Casella, Gianluca Mittone, Yasir Arfat, Barbara Cantalupo, Roberto Esposito, Alberto Riccardo Martinelli, Doriana Medić, Marco Aldinucci
Federated Learning meets HPC and cloud Proceedings Article
In: Bufano, Filomena, Riggi, Simone, Sciacca, Eva, Schillirò, Francesco (Ed.): Astrophysics and Space Science Proceedings, pp. 193–199, Springer, Catania, Italy, 2023, ISBN: 978-3-031-34167-0, (Keynote talk).
Abstract | Links | BibTeX | Tags: across, eupilot, streamflow
@inproceedings{22:ml4astro,
title = {Federated Learning meets HPC and cloud},
author = {Iacopo Colonnelli and Bruno Casella and Gianluca Mittone and Yasir Arfat and Barbara Cantalupo and Roberto Esposito and Alberto Riccardo Martinelli and Doriana Medić and Marco Aldinucci},
editor = {Filomena Bufano and Simone Riggi and Eva Sciacca and Francesco Schillirò},
url = {https://iris.unito.it/retrieve/3ac66baa-9d9a-4e9f-94a5-13700694d8aa/ML4Astro.pdf},
doi = {10.1007/978-3-031-34167-0_39},
isbn = {978-3-031-34167-0},
year = {2023},
date = {2023-01-01},
booktitle = {Astrophysics and Space Science Proceedings},
volume = {60},
pages = {193–199},
publisher = {Springer},
address = {Catania, Italy},
abstract = {HPC and AI are fated to meet for several reasons. This article will discuss some of them and argue why this will happen through the set of methods and technologies that underpin cloud computing. As a paradigmatic example, we present a new federated learning system that collaboratively trains a deep learning model in different supercomputing centers. The system is based on the StreamFlow workflow manager designed for hybrid cloud-HPC infrastructures.},
howpublished = {Machine Learning for Astrophysics (ML4ASTRO)},
note = {Keynote talk},
keywords = {across, eupilot, streamflow},
pubstate = {published},
tppubtype = {inproceedings}
}
Yasir Arfat, Gianluca Mittone, Iacopo Colonnelli, Fabrizio D'Ascenzo, Roberto Esposito, Marco Aldinucci
Pooling critical datasets with Federated Learning Proceedings Article
In: 31st Euromicro International Conference on Parallel, Distributed and Network-Based Processing, PDP 2023, pp. 329–337, IEEE, Napoli, Italy, 2023.
Abstract | Links | BibTeX | Tags: admire, ai, cardio, confidential, hpc4ai
@inproceedings{23:praise-fl:pdp,
title = {Pooling critical datasets with Federated Learning},
author = {Yasir Arfat and Gianluca Mittone and Iacopo Colonnelli and Fabrizio D'Ascenzo and Roberto Esposito and Marco Aldinucci},
url = {https://iris.unito.it/retrieve/491e22ec-3db5-4989-a063-085a199edd20/23_pdp_fl.pdf},
doi = {10.1109/PDP59025.2023.00057},
year = {2023},
date = {2023-01-01},
booktitle = {31st Euromicro International Conference on Parallel, Distributed and Network-Based Processing, PDP 2023},
pages = {329–337},
publisher = {IEEE},
address = {Napoli, Italy},
abstract = {Federated Learning (FL) is becoming popular in different industrial sectors where data access is critical for security, privacy and the economic value of data itself. Unlike traditional machine learning, where all the data must be globally gathered for analysis, FL makes it possible to extract knowledge from data distributed across different organizations that can be coupled with different Machine Learning paradigms. In this work, we replicate, using Federated Learning, the analysis of a pooled dataset (with AdaBoost) that has been used to define the PRAISE score, which is today among the most accurate scores to evaluate the risk of a second acute myocardial infarction. We show that thanks to the extended-OpenFL framework, which implements AdaBoost.F, we can train a federated PRAISE model that exhibits comparable accuracy and recall as the centralised model. We achieved F1 and F2 scores which are consistently comparable to the PRAISE score study of a 16- parties federation but within an order of magnitude less time.},
keywords = {admire, ai, cardio, confidential, hpc4ai},
pubstate = {published},
tppubtype = {inproceedings}
}
2022
Giovanni Agosta, Marco Aldinucci, Carlos Alvarez, Roberto Ammendola, Yasir Arfat, Olivier Beaumont, Massimo Bernaschi, Andrea Biagioni, Tommaso Boccali, Berenger Bramas, Carlo Brandolese, Barbara Cantalupo, Mauro Carrozzo, Daniele Cattaneo, Alessandro Celestini, Massimo Celino, Iacopo Colonnelli, Paolo Cretaro, Pasqua D'Ambra, Marco Danelutto, Roberto Esposito, Lionel Eyraud-Dubois, Antonio Filgueras, William Fornaciari, Ottorino Frezza, Andrea Galimberti, Francesco Giacomini, Brice Goglin, Daniele Gregori, Abdou Guermouche, Francesco Iannone, Michal Kulczewski, Francesca Lo Cicero, Alessandro Lonardo, Alberto R. Martinelli, Michele Martinelli, Xavier Martorell, Giuseppe Massari, Simone Montangero, Gianluca Mittone, Raymond Namyst, Ariel Oleksiak, Paolo Palazzari, Pier Stanislao Paolucci, Federico Reghenzani, Cristian Rossi, Sergio Saponara, Francesco Simula, Federico Terraneo, Samuel Thibault, Massimo Torquati, Matteo Turisini, Piero Vicini, Miquel Vidal, Davide Zoni, Giuseppe Zummo
Towards EXtreme scale technologies and accelerators for euROhpc hw/Sw supercomputing applications for exascale: The TEXTAROSSA approach Journal Article
In: Microprocessors and Microsystems, vol. 95, pp. 104679, 2022, ISSN: 0141-9331.
Abstract | Links | BibTeX | Tags: textarossa
@article{textarossa2022micpro:,
title = {Towards EXtreme scale technologies and accelerators for euROhpc hw/Sw supercomputing applications for exascale: The TEXTAROSSA approach},
author = {Giovanni Agosta and Marco Aldinucci and Carlos Alvarez and Roberto Ammendola and Yasir Arfat and Olivier Beaumont and Massimo Bernaschi and Andrea Biagioni and Tommaso Boccali and Berenger Bramas and Carlo Brandolese and Barbara Cantalupo and Mauro Carrozzo and Daniele Cattaneo and Alessandro Celestini and Massimo Celino and Iacopo Colonnelli and Paolo Cretaro and Pasqua D'Ambra and Marco Danelutto and Roberto Esposito and Lionel Eyraud-Dubois and Antonio Filgueras and William Fornaciari and Ottorino Frezza and Andrea Galimberti and Francesco Giacomini and Brice Goglin and Daniele Gregori and Abdou Guermouche and Francesco Iannone and Michal Kulczewski and Francesca Lo Cicero and Alessandro Lonardo and Alberto R. Martinelli and Michele Martinelli and Xavier Martorell and Giuseppe Massari and Simone Montangero and Gianluca Mittone and Raymond Namyst and Ariel Oleksiak and Paolo Palazzari and Pier Stanislao Paolucci and Federico Reghenzani and Cristian Rossi and Sergio Saponara and Francesco Simula and Federico Terraneo and Samuel Thibault and Massimo Torquati and Matteo Turisini and Piero Vicini and Miquel Vidal and Davide Zoni and Giuseppe Zummo},
doi = {10.1016/j.micpro.2022.104679},
issn = {0141-9331},
year = {2022},
date = {2022-01-01},
journal = {Microprocessors and Microsystems},
volume = {95},
pages = {104679},
abstract = {In the near future, Exascale systems will need to bridge three technology gaps to achieve high performance while remaining under tight power constraints: energy efficiency and thermal control; extreme computation efficiency via HW acceleration and new arithmetic; methods and tools for seamless integration of reconfigurable accelerators in heterogeneous HPC multi-node platforms. TEXTAROSSA addresses these gaps through a co-design approach to heterogeneous HPC solutions, supported by the integration and extension of HW and SW IPs, programming models, and tools derived from European research.},
keywords = {textarossa},
pubstate = {published},
tppubtype = {article}
}
Guglielmo Gallone, Jeehoon Kang, Francesco Bruno, Jung-Kyu Han, Ovidio De Filippo, Han-Mo Yang, Mattia Doronzo, Kyung-Woo Park, Gianluca Mittone, Hyun-Jae Kang, Radoslaw Parma, Hyeon-Cheol Gwon, Enrico Cerrato, Woo Jung Chun, Grzegorz Smolka, Seung-Ho Hur, Gerard Helft, Seung Hwan Han, Saverio Muscoli, Young Bin Song, Filippo Figini, Ki Hong Choi, Giacomo Boccuzzi, Soon-Jun Hong, Daniela Trabattoni, Chang-Wook Nam, Massimo Giammaria, Hyo-Soo Kim, Federico Conrotto, Javier Escaned, Carlo Di Mario, Fabrizio D'Ascenzo, Bon-Kwon Koo, Gaetano Maria Ferrari
Impact of Left Ventricular Ejection Fraction on Procedural and Long-Term Outcomes of Bifurcation Percutaneous Coronary Intervention Journal Article
In: The American Journal of Cardiology, vol. 172, pp. 18–25, 2022, ISSN: 0002-9149.
Abstract | Links | BibTeX | Tags: ai, cardio
@article{GALLONE202218,
title = {Impact of Left Ventricular Ejection Fraction on Procedural and Long-Term Outcomes of Bifurcation Percutaneous Coronary Intervention},
author = {Guglielmo Gallone and Jeehoon Kang and Francesco Bruno and Jung-Kyu Han and Ovidio De Filippo and Han-Mo Yang and Mattia Doronzo and Kyung-Woo Park and Gianluca Mittone and Hyun-Jae Kang and Radoslaw Parma and Hyeon-Cheol Gwon and Enrico Cerrato and Woo Jung Chun and Grzegorz Smolka and Seung-Ho Hur and Gerard Helft and Seung Hwan Han and Saverio Muscoli and Young Bin Song and Filippo Figini and Ki Hong Choi and Giacomo Boccuzzi and Soon-Jun Hong and Daniela Trabattoni and Chang-Wook Nam and Massimo Giammaria and Hyo-Soo Kim and Federico Conrotto and Javier Escaned and Carlo Di Mario and Fabrizio D'Ascenzo and Bon-Kwon Koo and Gaetano Maria Ferrari},
url = {https://www.sciencedirect.com/science/article/pii/S0002914922001692},
doi = {https://doi.org/10.1016/j.amjcard.2022.02.015},
issn = {0002-9149},
year = {2022},
date = {2022-01-01},
journal = {The American Journal of Cardiology},
volume = {172},
pages = {18–25},
abstract = {The association of left ventricular ejection fraction (LVEF) with procedural and long-term outcomes after state-of-the-art percutaneous coronary intervention (PCI) of bifurcation lesions remains unsettled. A total of 5,333 patients who underwent contemporary coronary bifurcation PCI were included in the intercontinental retrospective combined insights from the unified RAIN (veRy thin stents for patients with left mAIn or bifurcatioN in real life) and COBIS (COronary BIfurcation Stenting) III bifurcation registries. Of 5,003 patients (93.8%) with known baseline LVEF, 244 (4.9%) had LVEF <40% (bifurcation with reduced ejection fraction [BIFrEF] group), 430 (8.6%) had LVEF 40% to 49% (bifurcation with mildly reduced ejection fraction [BIFmEF] group) and 4,329 (86.5%) had ejection fraction (EF) ≥50% (bifurcation with preserved ejection fraction [BIFpEF] group). The primary end point was the Kaplan-Meier estimate of major adverse cardiac events (MACEs) (a composite of all-cause death, myocardial infarction, and target vessel revascularization). Patients with BIFrEF had a more complex clinical profile and coronary anatomy. No difference in procedural (30 days) MACE was observed across EF categories, also after adjustment for in-study outcome predictors (BIFrEF vs BIFmEF: adjusted hazard ratio [adj-HR] 1.39, 95% confidence interval [CI] 0.37 to 5.21},
keywords = {ai, cardio},
pubstate = {published},
tppubtype = {article}
}
2021
Giovanni Agosta, William Fornaciari, Andrea Galimberti, Giuseppe Massari, Federico Reghenzani, Federico Terraneo, Davide Zoni, Carlo Brandolese, Massimo Celino, Francesco Iannone, Paolo Palazzari, Giuseppe Zummo, Massimo Bernaschi, Pasqua D'Ambra, Sergio Saponara, Marco Danelutto, Massimo Torquati, Marco Aldinucci, Yasir Arfat, Barbara Cantalupo, Iacopo Colonnelli, Roberto Esposito, Alberto Riccardo Martinelli, Gianluca Mittone, Olivier Beaumont, Berenger Bramas, Lionel Eyraud-Dubois, Brice Goglin, Abdou Guermouche, Raymond Namyst, Samuel Thibault, Antonio Filgueras, Miquel Vidal, Carlos Alvarez, Xavier Martorell, Ariel Oleksiak, Michal Kulczewski, Alessandro Lonardo, Piero Vicini, Francesco Lo Cicero, Francesco Simula, Andrea Biagioni, Paolo Cretaro, Ottorino Frezza, Pier Stanislao Paolucci, Matteo Turisini, Francesco Giacomini, Tommaso Boccali, Simone Montangero, Roberto Ammendola
TEXTAROSSA: Towards EXtreme scale Technologies and Accelerators for euROhpc hw/Sw Supercomputing Applications for exascale Proceedings Article
In: Proc. of the 24th Euromicro Conference on Digital System Design (DSD), IEEE, Palermo, Italy, 2021.
Abstract | Links | BibTeX | Tags: streamflow, textarossa
@inproceedings{21:DSD:textarossa,
title = {TEXTAROSSA: Towards EXtreme scale Technologies and Accelerators for euROhpc hw/Sw Supercomputing Applications for exascale},
author = {Giovanni Agosta and William Fornaciari and Andrea Galimberti and Giuseppe Massari and Federico Reghenzani and Federico Terraneo and Davide Zoni and Carlo Brandolese and Massimo Celino and Francesco Iannone and Paolo Palazzari and Giuseppe Zummo and Massimo Bernaschi and Pasqua D'Ambra and Sergio Saponara and Marco Danelutto and Massimo Torquati and Marco Aldinucci and Yasir Arfat and Barbara Cantalupo and Iacopo Colonnelli and Roberto Esposito and Alberto Riccardo Martinelli and Gianluca Mittone and Olivier Beaumont and Berenger Bramas and Lionel Eyraud-Dubois and Brice Goglin and Abdou Guermouche and Raymond Namyst and Samuel Thibault and Antonio Filgueras and Miquel Vidal and Carlos Alvarez and Xavier Martorell and Ariel Oleksiak and Michal Kulczewski and Alessandro Lonardo and Piero Vicini and Francesco Lo Cicero and Francesco Simula and Andrea Biagioni and Paolo Cretaro and Ottorino Frezza and Pier Stanislao Paolucci and Matteo Turisini and Francesco Giacomini and Tommaso Boccali and Simone Montangero and Roberto Ammendola},
doi = {10.1109/DSD53832.2021.00051},
year = {2021},
date = {2021-08-01},
booktitle = {Proc. of the 24th Euromicro Conference on Digital System Design (DSD)},
publisher = {IEEE},
address = {Palermo, Italy},
abstract = {To achieve high performance and high energy effi- ciency on near-future exascale computing systems, three key technology gaps needs to be bridged. These gaps include: en- ergy efficiency and thermal control; extreme computation effi- ciency via HW acceleration and new arithmetics; methods and tools for seamless integration of reconfigurable accelerators in heterogeneous HPC multi-node platforms. TEXTAROSSA aims at tackling this gap through a co-design approach to heterogeneous HPC solutions, supported by the integration and extension of HW and SW IPs, programming models and tools derived from European research.},
keywords = {streamflow, textarossa},
pubstate = {published},
tppubtype = {inproceedings}
}
Marco Aldinucci, Valentina Cesare, Iacopo Colonnelli, Alberto Riccardo Martinelli, Gianluca Mittone, Barbara Cantalupo
Practical Parallelizazion of a Laplace Solver with MPI Proceedings Article
In: Iannone, Francesco (Ed.): ENEA CRESCO in the fight against COVID-19, pp. 21–24, ENEA, 2021.
Abstract | BibTeX | Tags: hpc4ai
@inproceedings{21:laplace:enea,
title = {Practical Parallelizazion of a Laplace Solver with MPI},
author = {Marco Aldinucci and Valentina Cesare and Iacopo Colonnelli and Alberto Riccardo Martinelli and Gianluca Mittone and Barbara Cantalupo},
editor = {Francesco Iannone},
year = {2021},
date = {2021-01-01},
booktitle = {ENEA CRESCO in the fight against COVID-19},
pages = {21–24},
publisher = {ENEA},
abstract = {This work exposes a practical methodology for the semi-automatic parallelization of existing code. We show how a scientific sequential code can be parallelized through our approach. The obtained parallel code is only slightly different from the starting sequential one, providing an example of how little re-designing our methodology involves. The performance of the parallelized code, executed on the CRESCO6 cluster, is then exposed and discussed. We also believe in the educational value of this approach and suggest its use as a teaching device for students.},
keywords = {hpc4ai},
pubstate = {published},
tppubtype = {inproceedings}
}
Yasir Arfat, Gianluca Mittone, Roberto Esposito, Barbara Cantalupo, Gaetano Maria De Ferrari, Marco Aldinucci
A Review of Machine Learning for Cardiology Journal Article
In: Minerva cardiology and angiology, 2021.
Abstract | Links | BibTeX | Tags: deephealth, hpc4ai
@article{21:ai4numbers:minerva,
title = {A Review of Machine Learning for Cardiology},
author = {Yasir Arfat and Gianluca Mittone and Roberto Esposito and Barbara Cantalupo and Gaetano Maria De Ferrari and Marco Aldinucci},
url = {https://iris.unito.it/retrieve/handle/2318/1796298/780512/21_AI4numbers-preprint.pdf},
doi = {10.23736/s2724-5683.21.05709-4},
year = {2021},
date = {2021-01-01},
journal = {Minerva cardiology and angiology},
abstract = {This paper reviews recent cardiology literature and reports how Artificial Intelligence Tools (specifically, Machine Learning techniques) are being used by physicians in the field. Each technique is introduced with enough details to allow the understanding of how it works and its intent, but without delving into details that do not add immediate benefits and require expertise in the field. We specifically focus on the principal Machine Learning based risk scores used in cardiovascular research. After introducing them and summarizing their assumptions and biases, we discuss their merits and shortcomings. We report on how frequently they are adopted in the field and suggest why this is the case based on our expertise in Machine Learning. We complete the analysis by reviewing how corresponding statistical approaches compare with them. Finally, we discuss the main open issues in applying Machine Learning tools to cardiology tasks, also drafting possible future directions. Despite the growing interest in these tools, we argue that there are many still underutilized techniques: while Neural Networks are slowly being incorporated in cardiovascular research, other important techniques such as Semi-Supervised Learning and Federated Learning are still underutilized. The former would allow practitioners to harness the information contained in large datasets that are only partially labeled, while the latter would foster collaboration between institutions allowing building larger and better models.},
keywords = {deephealth, hpc4ai},
pubstate = {published},
tppubtype = {article}
}
Marco Aldinucci, Valentina Cesare, Iacopo Colonnelli, Alberto Riccardo Martinelli, Gianluca Mittone, Barbara Cantalupo, Carlo Cavazzoni, Maurizio Drocco
Practical Parallelization of Scientific Applications with OpenMP, OpenACC and MPI Journal Article
In: Journal of Parallel and Distributed Computing, vol. 157, pp. 13–29, 2021.
Abstract | Links | BibTeX | Tags: HPC
@article{21:jpdc:loop,
title = {Practical Parallelization of Scientific Applications with OpenMP, OpenACC and MPI},
author = {Marco Aldinucci and Valentina Cesare and Iacopo Colonnelli and Alberto Riccardo Martinelli and Gianluca Mittone and Barbara Cantalupo and Carlo Cavazzoni and Maurizio Drocco},
url = {https://iris.unito.it/retrieve/handle/2318/1792557/770851/Practical_Parallelization_JPDC_preprint.pdf},
doi = {10.1016/j.jpdc.2021.05.017},
year = {2021},
date = {2021-01-01},
journal = {Journal of Parallel and Distributed Computing},
volume = {157},
pages = {13–29},
abstract = {This work aims at distilling a systematic methodology to modernize existing sequential scientific codes with a little re-designing effort, turning an old codebase into emphmodern code, i.e., parallel and robust code. We propose a semi-automatic methodology to parallelize scientific applications designed with a purely sequential programming mindset, possibly using global variables, aliasing, random number generators, and stateful functions. We demonstrate that the same methodology works for the parallelization in the shared memory model (via OpenMP), message passing model (via MPI), and General Purpose Computing on GPU model (via OpenACC). The method is demonstrated parallelizing four real-world sequential codes in the domain of physics and material science. The methodology itself has been distilled in collaboration with MSc students of the Parallel Computing course at the University of Torino, that applied it for the first time to the project works that they presented for the final exam of the course. Every year the course hosts some special lectures from industry representatives, who present how they use parallel computing and offer codes to be parallelizeda.},
keywords = {HPC},
pubstate = {published},
tppubtype = {article}
}
Fabrizio D'Ascenzo, Ovidio De Filippo, Guglielmo Gallone, Gianluca Mittone, Marco Agostino Deriu, Mario Iannaccone, Albert Ariza-Solé, Christoph Liebetrau, Sergio Manzano-Fernández, Giorgio Quadri, Tim Kinnaird, Gianluca Campo, Jose Paulo Simao Henriques, James M Hughes, Alberto Dominguez-Rodriguez, Marco Aldinucci, Umberto Morbiducci, Giuseppe Patti, Sergio Raposeiras-Roubin, Emad Abu-Assi, Gaetano Maria De Ferrari, Francesco Piroli, Andrea Saglietto, Federico Conrotto, Pierluigi Omedé, Antonio Montefusco, Mauro Pennone, Francesco Bruno, Pier Paolo Bocchino, Giacomo Boccuzzi, Enrico Cerrato, Ferdinando Varbella, Michela Sperti, Stephen B. Wilton, Lazar Velicki, Ioanna Xanthopoulou, Angel Cequier, Andres Iniguez-Romo, Isabel Munoz Pousa, Maria Cespon Fernandez, Berenice Caneiro Queija, Rafael Cobas-Paz, Angel Lopez-Cuenca, Alberto Garay, Pedro Flores Blanco, Andrea Rognoni, Giuseppe Biondi Zoccai, Simone Biscaglia, Ivan Nunez-Gil, Toshiharu Fujii, Alessandro Durante, Xiantao Song, Tetsuma Kawaji, Dimitrios Alexopoulos, Zenon Huczek, Jose Ramon Gonzalez Juanatey, Shao-Ping Nie, Masa-aki Kawashiri, Iacopo Colonnelli, Barbara Cantalupo, Roberto Esposito, Sergio Leonardi, Walter Grosso Marra, Alaide Chieffo, Umberto Michelucci, Dario Piga, Marta Malavolta, Sebastiano Gili, Marco Mennuni, Claudio Montalto, Luigi Oltrona Visconti, Yasir Arfat
Machine learning-based prediction of adverse events following an acute coronary syndrome (PRAISE): a modelling study of pooled datasets Journal Article
In: The Lancet, vol. 397, no. 10270, pp. 199–207, 2021, ISSN: 0140-6736.
Abstract | Links | BibTeX | Tags: ai, cardio, deephealth, hpc4ai
@article{21:lancet,
title = {Machine learning-based prediction of adverse events following an acute coronary syndrome (PRAISE): a modelling study of pooled datasets},
author = {Fabrizio D'Ascenzo and Ovidio De Filippo and Guglielmo Gallone and Gianluca Mittone and Marco Agostino Deriu and Mario Iannaccone and Albert Ariza-Solé and Christoph Liebetrau and Sergio Manzano-Fernández and Giorgio Quadri and Tim Kinnaird and Gianluca Campo and Jose Paulo Simao Henriques and James M Hughes and Alberto Dominguez-Rodriguez and Marco Aldinucci and Umberto Morbiducci and Giuseppe Patti and Sergio Raposeiras-Roubin and Emad Abu-Assi and Gaetano Maria De Ferrari and Francesco Piroli and Andrea Saglietto and Federico Conrotto and Pierluigi Omedé and Antonio Montefusco and Mauro Pennone and Francesco Bruno and Pier Paolo Bocchino and Giacomo Boccuzzi and Enrico Cerrato and Ferdinando Varbella and Michela Sperti and Stephen B. Wilton and Lazar Velicki and Ioanna Xanthopoulou and Angel Cequier and Andres Iniguez-Romo and Isabel Munoz Pousa and Maria Cespon Fernandez and Berenice Caneiro Queija and Rafael Cobas-Paz and Angel Lopez-Cuenca and Alberto Garay and Pedro Flores Blanco and Andrea Rognoni and Giuseppe Biondi Zoccai and Simone Biscaglia and Ivan Nunez-Gil and Toshiharu Fujii and Alessandro Durante and Xiantao Song and Tetsuma Kawaji and Dimitrios Alexopoulos and Zenon Huczek and Jose Ramon Gonzalez Juanatey and Shao-Ping Nie and Masa-aki Kawashiri and Iacopo Colonnelli and Barbara Cantalupo and Roberto Esposito and Sergio Leonardi and Walter Grosso Marra and Alaide Chieffo and Umberto Michelucci and Dario Piga and Marta Malavolta and Sebastiano Gili and Marco Mennuni and Claudio Montalto and Luigi Oltrona Visconti and Yasir Arfat},
url = {https://www.researchgate.net/profile/James_Hughes3/publication/348501148_Machine_learning-based_prediction_of_adverse_events_following_an_acute_coronary_syndrome_PRAISE_a_modelling_study_of_pooled_datasets/links/6002a81ba6fdccdcb858b6c2/Machine-learning-based-prediction-of-adverse-events-following-an-acute-coronary-syndrome-PRAISE-a-modelling-study-of-pooled-datasets.pdf},
doi = {10.1016/S0140-6736(20)32519-8},
issn = {0140-6736},
year = {2021},
date = {2021-01-01},
journal = {The Lancet},
volume = {397},
number = {10270},
pages = {199–207},
abstract = {Background The accuracy of current prediction tools for ischaemic and bleeding events after an acute coronary syndrome (ACS) remains insufficient for individualised patient management strategies. We developed a machine learning-based risk stratification model to predict all-cause death, recurrent acute myocardial infarction, and major bleeding after ACS. Methods Different machine learning models for the prediction of 1-year post-discharge all-cause death, myocardial infarction, and major bleeding (defined as Bleeding Academic Research Consortium type 3 or 5) were trained on a cohort of 19826 adult patients with ACS (split into a training cohort [80%] and internal validation cohort [20%]) from the BleeMACS and RENAMI registries, which included patients across several continents. 25 clinical features routinely assessed at discharge were used to inform the models. The best-performing model for each study outcome (the PRAISE score) was tested in an external validation cohort of 3444 patients with ACS pooled from a randomised controlled trial and three prospective registries. Model performance was assessed according to a range of learning metrics including area under the receiver operating characteristic curve (AUC). Findings The PRAISE score showed an AUC of 0.82 (95% CI 0.78-0.85) in the internal validation cohort and 0.92 (0.90-0.93) in the external validation cohort for 1-year all-cause death; an AUC of 0.74 (0.70-0.78) in the internal validation cohort and 0.81 (0.76-0.85) in the external validation cohort for 1-year myocardial infarction; and an AUC of 0.70 (0.66-0.75) in the internal validation cohort and 0.86 (0.82-0.89) in the external validation cohort for 1-year major bleeding. Interpretation A machine learning-based approach for the identification of predictors of events after an ACS is feasible and effective. The PRAISE score showed accurate discriminative capabilities for the prediction of all-cause death, myocardial infarction, and major bleeding, and might be useful to guide clinical decision making.},
keywords = {ai, cardio, deephealth, hpc4ai},
pubstate = {published},
tppubtype = {article}
}
Talks
2024
Gianluca Mittone
Benchmarking HPC Performance for State-of-the-Art AI Workloads Miscellaneous
2024.
Abstract | Links | BibTeX | Tags: ai, eupilot, icsc
@misc{24:mittone:itadata:shpcpee,
title = {Benchmarking HPC Performance for State-of-the-Art AI Workloads},
author = {Gianluca Mittone},
url = {https://datacloud.di.unito.it/index.php/s/5Ep3W7cPW5baZfr},
year = {2024},
date = {2024-09-01},
address = {Pisa, Italy},
abstract = {Benchmarking the performance of modern High-Performance Computing (HPC) infrastructure on Artificial Intelligence (AI) workloads is a hot topic in the supercomputing community. While research communities and big-tech companies actively invest in larger, more powerful data centres to support AI research, the standard computational performance benchmarking tools (e.g., LINPACK) are increasingly becoming outdated since they are not specifically tailored for AI workloads. Some tools, such as MLPerf, are trying to bridge this gap, but the HPC community still has not adopted them as standards. Since this trend became particularly evident with the advent of Large Language Models (LLMs), this work will delve into LLM training at scale as a way to benchmark Top500 HPC infrastructures on current AI workloads. The scalability performances of a major LLM model (i.e., Meta's LLaMA) on different HPCs (Leonardo, LUMI, MeluXina, Karolina) are exposed and discussed along with their Top500 positioning.
However, it should be noted that state-of-the-art LLM models are not trained on thousands of computing nodes but on hundreds. This choice is due to multiple factors, such as the influence of the training scaling on the model's convergence and the instability of large-scale deployments due to hardware/software failure. A benchmarking approach based on the next-generation LLM training approach is proposed to bypass all these issues. State-of-the-art LLMs are not monolithic structures but Mixture-of-Experts (MoE) models; this design implies innovative frontiers for the distributed training of such models due to the experts' training being potentially more parallelisable than a single monolithic model. We thus propose to create an AI-oriented HPC benchmark suite based on the parallel training of MoE models to measure the throughput performance of HPC systems on state-of-the-art AI workloads.},
keywords = {ai, eupilot, icsc},
pubstate = {published},
tppubtype = {misc}
}
However, it should be noted that state-of-the-art LLM models are not trained on thousands of computing nodes but on hundreds. This choice is due to multiple factors, such as the influence of the training scaling on the model's convergence and the instability of large-scale deployments due to hardware/software failure. A benchmarking approach based on the next-generation LLM training approach is proposed to bypass all these issues. State-of-the-art LLMs are not monolithic structures but Mixture-of-Experts (MoE) models; this design implies innovative frontiers for the distributed training of such models due to the experts' training being potentially more parallelisable than a single monolithic model. We thus propose to create an AI-oriented HPC benchmark suite based on the parallel training of MoE models to measure the throughput performance of HPC systems on state-of-the-art AI workloads.
Gianluca Mittone
Pushing Federated Learning Boundaries: Three Innovative Distributed Intelligence Approaches Miscellaneous
2024.
Abstract | Links | BibTeX | Tags: eupilot, fl, icsc
@misc{24:mittone:bighpc,
title = {Pushing Federated Learning Boundaries: Three Innovative Distributed Intelligence Approaches},
author = {Gianluca Mittone},
url = {https://datacloud.di.unito.it/index.php/s/eKbRtSAEdmSFJYW},
year = {2024},
date = {2024-09-01},
address = {Pisa, Italy},
abstract = {Federated learning is a distributed, privacy-preserving machine learning technique used on private, decentralised data. It allows multiple parties to cooperatively solve a common machine learning problem without sharing the local data. Three assumptions of state-of-the-art federated learning software constitute the starting points for this research work: 1) their inner workings being strictly tied to deep learning models, 2) the centralised structure currently implemented by many commercial frameworks, and 3) their assumption of being deployed on private, specialised computing infrastructures. The proposed research expands the federated learning paradigm to handle scenarios in which these three conditions do not hold. Such research problems are addressed methodologically and practically, and three open-source, proof-of-concept software are made freely available as tangible research results: OpenFL-x, FastFL, and xFFL.},
keywords = {eupilot, fl, icsc},
pubstate = {published},
tppubtype = {misc}
}
Iacopo Colonnelli, Robert Birke, Giulio Malenza, Gianluca Mittone, Alberto Mulone, Marco Aldinucci
Cross-Facility Federated Learning - Part II Miscellaneous
2024, (Invited talk).
Links | BibTeX | Tags: eupex, icsc, space
@misc{24:ic:elise:xffl,
title = {Cross-Facility Federated Learning - Part II},
author = {Iacopo Colonnelli and Robert Birke and Giulio Malenza and Gianluca Mittone and Alberto Mulone and Marco Aldinucci},
url = {https://datacloud.di.unito.it/index.php/s/7HonBpcWPxotXLX},
year = {2024},
date = {2024-06-01},
address = {Helsinki, Finland},
note = {Invited talk},
keywords = {eupex, icsc, space},
pubstate = {published},
tppubtype = {misc}
}
Gianluca Mittone
Into to Federated Learning Miscellaneous
2024.
Abstract | Links | BibTeX | Tags: fl, icsc
@misc{24:mittone:ictp,
title = {Into to Federated Learning},
author = {Gianluca Mittone},
url = {https://datacloud.di.unito.it/index.php/s/nSwwmedjqe2jbWJ},
year = {2024},
date = {2024-05-01},
address = {Trieste, Italy},
abstract = {Machine Learning (ML) is the branch of Artificial Intelligence focused on developing algorithms capable of adapting and improving their predictive or generative performance by feeding on data. Adapting or improving the system’s behaviour based on the provided data is called learning since it is similar to the human learning process in many aspects. The same ML algorithm, usually referred to as a model, trained on different data will thus expose different capabilities and can, therefore, solve different tasks. FL is a relatively recent distributed ML methodology aiming to bridge the gap between the need to train ever bigger ML models on ever larger datasets and the individual and companies’ will to protect and not share their private data. From another point of view, FL is also a way to distribute the training of an ML model even more than before. However, it should be considered that the learning performance of FL is usually lower than that of traditional centralised learning. This course will start from Kairouz ad McMahan’s definition of FL: ”Federated learning is a machine learning setting where multiple entities (clients) collaborate in solving a machine learning problem, under the coordination of a central server or service provider. Each client’s raw data is stored locally and not exchanged or transferred; instead, focused updates intended for immediate aggregation are used to achieve the learning objective.” From this starting point, the most significant aspects of FL will be exposed and discussed. This tutorial will particularly explore FL from both the learning and computational [5] performance perspectives, investigating its pros and cons in a distributed ML setting. Since FL natively targets data privacy, some insights on how the FL process can be attacked and protected will also be discussed from a high-level perspective. Finally, a hands-on session will guide the participants in building a basic FL system, providing a better understanding of the major implementational difficulties of such a technique.},
keywords = {fl, icsc},
pubstate = {published},
tppubtype = {misc}
}
Gianluca Mittone
RISC-V for AI Miscellaneous
High Performance, Edge And Cloud computing Conference 2024 (HiPEAC 2024), 2024.
Abstract | Links | BibTeX | Tags: eupilot, icsc
@misc{24:HiPEAC:riscv,
title = {RISC-V for AI},
author = {Gianluca Mittone},
url = {https://datacloud.di.unito.it/index.php/s/rFtxT7zryoKNGbP},
year = {2024},
date = {2024-01-01},
address = {Garching bei München, München, Germany},
abstract = {AI-focused RISC-V-based hardware accelerators},
howpublished = {High Performance, Edge And Cloud computing Conference 2024 (HiPEAC 2024)},
keywords = {eupilot, icsc},
pubstate = {published},
tppubtype = {misc}
}
2023
Iacopo Colonnelli, Robert Birke, Giulio Malenza, Gianluca Mittone, Alberto Mulone, Marco Aldinucci, Valerio Basile, Marco Antonio Stranisci, Viviana Patti, Jeroen Galjaard, Lydia Y. Chen, Sanzio Bassini, Massimiliano Guarrasi, Gabriella Scipione, Jan Martinovič, Vit Vondrák
Cross-Facility Federated Learning Miscellaneous
1st EuroHPC User Day, 2023.
Links | BibTeX | Tags: across, ai, eupex, eupilot, HPC
@misc{23:eurohpc,
title = {Cross-Facility Federated Learning},
author = {Iacopo Colonnelli and Robert Birke and Giulio Malenza and Gianluca Mittone and Alberto Mulone and Marco Aldinucci and Valerio Basile and Marco Antonio Stranisci and Viviana Patti and Jeroen Galjaard and Lydia Y. Chen and Sanzio Bassini and Massimiliano Guarrasi and Gabriella Scipione and Jan Martinovič and Vit Vondrák},
url = {https://datacloud.di.unito.it/index.php/s/DDAz4QkJP3WZ68M},
year = {2023},
date = {2023-12-01},
address = {Bruxelles, Belgium},
howpublished = {1st EuroHPC User Day},
keywords = {across, ai, eupex, eupilot, HPC},
pubstate = {published},
tppubtype = {misc}
}
Gianluca Mittone, Giulio Malenza, Marco Aldinucci, Robert Birke
Distributed Edge Inference: an Experimental Study on Multiview Detection Miscellaneous
The 16th IEEE/ACM International Conference on Utility and Cloud Computing (UCC 2023), 2023.
Abstract | Links | BibTeX | Tags: ai, eupilot, icsc
@misc{23:ucc:multiview,
title = {Distributed Edge Inference: an Experimental Study on Multiview Detection},
author = {Gianluca Mittone and Giulio Malenza and Marco Aldinucci and Robert Birke},
url = {https://datacloud.di.unito.it/index.php/s/XfjNZEPSNfSKPFr},
year = {2023},
date = {2023-12-01},
address = {Taormina, Italy},
abstract = {Computing is evolving rapidly to cater to the increasing demand for sophisticated services, and Cloud computing lays a solid foundation for flexible on-demand provisioning. However, as the size of applications grows, the centralised client-server approach used by Cloud computing increasingly limits the applications scalability. To achieve ultra-scalability, cloud/edge/fog computing converges into the compute continuum, completely decentralising the infrastructure to encompass universal, pervasive resources. The compute continuum makes devising applications benefitting from this complex environment a challenging research problem. We put the opportunities the compute continuum others to the test through a real-world multi-view detection model (MvDet) implemented with the FastFL C/C++ high-performance edge inference framework. Computational performance is discussed considering many experimental scenarios, encompassing different edge computational capabilities and network bandwidths. We obtain up to 1.92x speedup in inference time over a centralised solution using the same devices.},
howpublished = {The 16th IEEE/ACM International Conference on Utility and Cloud Computing (UCC 2023)},
keywords = {ai, eupilot, icsc},
pubstate = {published},
tppubtype = {misc}
}
Gianluca Mittone, Samuele Fonio
Benchmarking Federated Learning Scalability Miscellaneous
2nd Italian Conference on Big Data and Data Science (ITADATA 2023), 2023.
Abstract | Links | BibTeX | Tags: ai, eupilot, fl, icsc
@misc{23:itadata:fl_scaling,
title = {Benchmarking Federated Learning Scalability},
author = {Gianluca Mittone and Samuele Fonio},
url = {https://datacloud.di.unito.it/index.php/s/QZGxC4X3s5LG5oT},
year = {2023},
date = {2023-09-01},
address = {Naples, Italy},
abstract = {Federated Learning (FL) is a widespread Machine Learning paradigm handling distributed Big Data. In this work, we demonstrate that different FL frameworks expose different scaling performances despite adopting the same technologies, highlighting the need for a more comprehensive study on the topic.},
howpublished = {2nd Italian Conference on Big Data and Data Science (ITADATA 2023)},
keywords = {ai, eupilot, fl, icsc},
pubstate = {published},
tppubtype = {misc}
}
Gianluca Mittone, Walter Riviera, Iacopo Colonnelli, Robert Birke, Marco Aldinucci
Model-Agnostic Federated Learning Miscellaneous
29th International European Conference on Parallel and Distributed Computing (Euro-Par '23), 2023.
Abstract | Links | BibTeX | Tags: ai, eupilot, icsc
@misc{23:europar:mafl,
title = {Model-Agnostic Federated Learning},
author = {Gianluca Mittone and Walter Riviera and Iacopo Colonnelli and Robert Birke and Marco Aldinucci},
url = {https://datacloud.di.unito.it/index.php/s/9T6G2tRreRomBAE},
year = {2023},
date = {2023-09-01},
address = {Limassol, Cyprus},
abstract = {Since its debut in 2016, Federated Learning (FL) has been tied to the inner workings of Deep Neural Networks (DNNs); this allowed its development as DNNs proliferated but neglected those scenarios in which using DNNs is not possible or advantageous. The fact that most current FL frameworks only support DNNs reinforces this problem. To address the lack of non-DNN-based FL solutions, we propose MAFL (Model-Agnostic Federated Learning). MAFL merges a model-agnostic FL algorithm, AdaBoost.F, with an open industry-grade FL framework: Intel® OpenFL. MAFL is the first FL system not tied to any machine learning model, allowing exploration of FL beyond DNNs. We test MAFL from multiple points of view, assessing its correctness, flexibility, and scaling properties up to 64 nodes of an HPC cluster. We also show how we optimised OpenFL achieving a 5.5x speedup over a standard FL scenario. MAFL is compatible with x86-64, ARM-v8, Power and RISC-V.},
howpublished = {29th International European Conference on Parallel and Distributed Computing (Euro-Par '23)},
keywords = {ai, eupilot, icsc},
pubstate = {published},
tppubtype = {misc}
}
Gianluca Mittone, Robert Birke, Marco Aldinucci
Model-Agnostic Federated Learning Miscellaneous
29th International European Conference on Parallel and Distributed Computing (Euro-Par '23), 2023.
Abstract | Links | BibTeX | Tags: eupilot, icsc
@misc{23:europar:phdtalk,
title = {Model-Agnostic Federated Learning},
author = {Gianluca Mittone and Robert Birke and Marco Aldinucci},
url = {https://datacloud.di.unito.it/index.php/s/pT3qxkwzzsHR3nS},
year = {2023},
date = {2023-08-01},
address = {Limassol, Cyprus},
abstract = {Since its debut in 2016, Federated Learning (FL) has been tied to the inner workings of Deep Neural Networks (DNNs); this allowed its development as DNNs proliferated but neglected those scenarios in which using DNNs is not possible or advantageous. The fact that most current FL frameworks only support DNNs reinforces this problem. To address the lack of non-DNN-based FL solutions, we propose MAFL (Model-Agnostic Federated Learning). Decentralised Machine Learning (DML) enables collaborative machine learning without centralised input data. Federated Learning (FL) and Edge Inference are examples of DML. While tools for DML (especially FL) are starting to flourish, many are not flexible and portable enough to experiment with novel processors (e.g., RISC-V), non-fully connected network topologies, and asynchronous collaboration schemes. We overcome these limitations via a domain-specific language allowing us to map DML schemes to an underlying middleware, i.e. the FastFlow parallel programming library.},
howpublished = {29th International European Conference on Parallel and Distributed Computing (Euro-Par '23)},
keywords = {eupilot, icsc},
pubstate = {published},
tppubtype = {misc}
}
Gianluca Mittone, Nicolò Tonci, Robert Birke, Iacopo Colonnelli, Doriana Medić, Andrea Bartolini, Roberto Esposito, Emanuele Parisi, Francesco Beneventi, Mirko Polato, Massimo Torquati, Luca Benini, Marco Aldinucci
Experimenting with Emerging RISC-V Systems for Decentralised Machine Learning Miscellaneous
20th ACM international conference on computing frontiers (CF '23), 2023, (Invited talk).
Abstract | Links | BibTeX | Tags: ai, eupilot, icsc
@misc{23:ACMCF,
title = {Experimenting with Emerging RISC-V Systems for Decentralised Machine Learning},
author = {Gianluca Mittone and Nicolò Tonci and Robert Birke and Iacopo Colonnelli and Doriana Medić and Andrea Bartolini and Roberto Esposito and Emanuele Parisi and Francesco Beneventi and Mirko Polato and Massimo Torquati and Luca Benini and Marco Aldinucci},
url = {https://datacloud.di.unito.it/index.php/s/BYyqZbHzzN4DL8Z},
year = {2023},
date = {2023-05-01},
abstract = {Decentralised Machine Learning (DML) enables collaborative machine learning without centralised input data. Federated Learning (FL) and Edge Inference are examples of DML. While tools for DML (especially FL) are starting to flourish, many are not flexible and portable enough to experiment with novel processors (e.g., RISC-V), non-fully connected network topologies, and asynchronous collaboration schemes. We overcome these limitations via a domain-specific language allowing us to map DML schemes to an underlying middleware, i.e. the FastFlow parallel programming library. We experiment with it by generating different working DML schemes on x86-64 and ARM platforms and an emerging RISC-V one. We characterise the performance and energy efficiency of the presented schemes and systems. As a byproduct, we introduce a RISC-V porting of the PyTorch framework, the first publicly available to our knowledge.},
howpublished = {20th ACM international conference on computing frontiers (CF '23)},
note = {Invited talk},
keywords = {ai, eupilot, icsc},
pubstate = {published},
tppubtype = {misc}
}
Gianluca Mittone, Filip Svoboda, Marco Aldinucci, Nicholas D. Lane, Pietro Lio'
A Federated Learning Benchmark for Drug-Target Interaction Miscellaneous
2023 ACM international Web Conference (WWW '23), 2023, (Invited talk).
Abstract | Links | BibTeX | Tags: eupilot, icsc
@misc{23:WWW,
title = {A Federated Learning Benchmark for Drug-Target Interaction},
author = {Gianluca Mittone and Filip Svoboda and Marco Aldinucci and Nicholas D. Lane and Pietro Lio'},
url = {https://datacloud.di.unito.it/index.php/s/js7go3EorZxSLn9},
year = {2023},
date = {2023-05-01},
abstract = {Aggregating pharmaceutical data in the drug-target interaction (DTI) domain can potentially deliver life-saving breakthroughs. It is, however, notoriously difficult due to regulatory constraints and commercial interests. This work proposes the application of federated learning, which is reconcilable with the industry's constraints. It does not require sharing any information that would reveal the entities' data or any other high-level summary. When used on a representative GraphDTA model and the KIBA dataset, it achieves up to 15% improved performance relative to the best available non-privacy preserving alternative. Our extensive battery of experiments shows that, unlike in other domains, the non-IID data distribution in the DTI datasets does not deteriorate FL performance. Additionally, we identify a material trade-off between the benefits of adding new data and the cost of adding more clients.},
howpublished = {2023 ACM international Web Conference (WWW '23)},
note = {Invited talk},
keywords = {eupilot, icsc},
pubstate = {published},
tppubtype = {misc}
}
Gianluca Mittone
Paving the way to innovative tools for Federated Learning Miscellaneous
2023 HiPEAC Conference, 2023, (Invited talk).
Abstract | Links | BibTeX | Tags: eupilot
@misc{23:hipeac,
title = {Paving the way to innovative tools for Federated Learning},
author = {Gianluca Mittone},
url = {https://datacloud.di.unito.it/index.php/s/2GtxPidHq79RTzA},
year = {2023},
date = {2023-02-01},
address = {Toulouse, France},
abstract = {Since its debut in 2016, Federated Learning (FL) has been tied to the inner workings of Deep Neural Networks (DNNs). On the one hand, this allowed its development and widespread use as DNNs proliferated. On the other hand, it neglected all those scenarios in which using DNNs is not possible or advantageous. The fact that most current FL frameworks only allow training DNNs reinforces this problem. To address the lack of FL solutions for non-DNN-based use cases, we propose MAFL (Model-Agnostic Federated Learning). MAFL marries a model-agnostic FL algorithm, AdaBoost.F, with an open industry-grade FL framework: Intel® OpenFL. MAFL is the first FL system not tied to any specific type of machine learning model, allowing exploration of FL scenarios beyond DNNs and trees. Furthermore, tools for DML (especially FL) are starting to flourish, many are not flexible and portable enough to experiment with novel systems (e.g., RISC-V), non-fully connected topologies, and asynchronous collaboration schemes. We overcome these limitations via a domain-specific language allowing to map DML schemes to an underlying middleware, i.e. the ff parallel programming library. As a byproduct, we introduce a RISC-V porting of the PyTorch framework, the first publicly available to our knowledge.},
howpublished = {2023 HiPEAC Conference},
note = {Invited talk},
keywords = {eupilot},
pubstate = {published},
tppubtype = {misc}
}
2022
Gianluca Mittone
Python for the human heart: a case study Miscellaneous
2022 Python Conference, 2022, (Invited talk).
Abstract | Links | BibTeX | Tags:
@misc{22:PyCon,
title = {Python for the human heart: a case study},
author = {Gianluca Mittone},
url = {https://datacloud.di.unito.it/index.php/s/fERJB5xeE6cXm4N},
year = {2022},
date = {2022-05-01},
address = {Florence, Italy},
abstract = {Presentation of the "Machine learning-based prediction of adverse events following an acute coronary syndrome (PRAISE): a modelling study of pooled datasets" paper at the PyCon22 DataBeer event.},
howpublished = {2022 Python Conference},
note = {Invited talk},
keywords = {},
pubstate = {published},
tppubtype = {misc}
}