Barbara Cantalupo
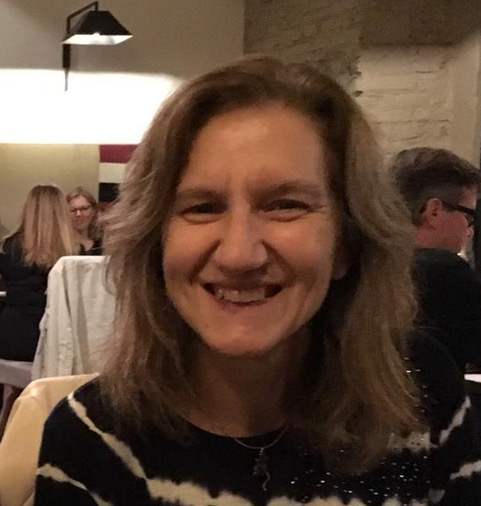
Research Engineer
Computer Science Department, University of Turin
Parallel Computing group
Via Pessinetto 12, 10149 Torino – Italy
E-mail: barbara.cantalupo AT unito.it
Short Bio
Barbara Cantalupo is a Research Engineer at the Computer Science Department of the University of Torino. She received her master’s degree in Computer Science from the University of Pisa (1994), and she has been a researcher in the parallel computing field at University and at the Italian National Research Council (CNR). Afterwards, she has worked for 15 years in several private companies in the area of supercomputing, mobile networks and space, acquiring knowledge on different application fields.
Fields of interest:
- Workflows, cloud and containers for HPC.
- European projects dissemination and exploitation activity.
Working on the following European Research project:
Publications
2023
Alberto Riccardo Martinelli, Massimo Torquati, Marco Aldinucci, Iacopo Colonnelli, Barbara Cantalupo
CAPIO: a Middleware for Transparent I/O Streaming in Data-Intensive Workflows Proceedings Article
In: 2023 IEEE 30th International Conference on High Performance Computing, Data, and Analytics (HiPC), IEEE, Goa, India, 2023.
Abstract | Links | BibTeX | Tags: admire, capio, eupex, icsc
@inproceedings{23:hipc:capio,
title = {CAPIO: a Middleware for Transparent I/O Streaming in Data-Intensive Workflows},
author = {Alberto Riccardo Martinelli and Massimo Torquati and Marco Aldinucci and Iacopo Colonnelli and Barbara Cantalupo},
url = {https://iris.unito.it/retrieve/27380f37-0978-409e-a9d8-2b5e95a4bb85/CAPIO-HiPC23-preprint.pdf},
doi = {10.1109/HiPC58850.2023.00031},
year = {2023},
date = {2023-12-01},
booktitle = {2023 IEEE 30th International Conference on High Performance Computing, Data, and Analytics (HiPC)},
publisher = {IEEE},
address = {Goa, India},
abstract = {With the increasing amount of digital data available for analysis and simulation, the class of I/O-intensive HPC workflows is fated to quickly expand, further exacerbating the performance gap between computing, memory, and storage technologies. This paper introduces CAPIO (Cross-Application Programmable I/O), a middleware capable of injecting I/O streaming capabilities into file-based workflows, improving the computation-I/O overlap without the need to change the application code. The contribution is twofold: 1) at design time, a new I/O coordination language allows users to annotate workflow data dependencies with synchronization semantics; 2) at run time, a user-space middleware automatically and transparently to the user turns a workflow batch execution into a streaming execution according to the semantics expressed in the configuration file. CAPIO has been tested on synthetic benchmarks simulating typical workflow I/O patterns and two real-world workflows. Experiments show that CAPIO reduces the execution time by 10% to 66% for data-intensive workflows that use the file system as a communication medium.},
keywords = {admire, capio, eupex, icsc},
pubstate = {published},
tppubtype = {inproceedings}
}
2022
Giovanni Agosta, Marco Aldinucci, Carlos Alvarez, Roberto Ammendola, Yasir Arfat, Olivier Beaumont, Massimo Bernaschi, Andrea Biagioni, Tommaso Boccali, Berenger Bramas, Carlo Brandolese, Barbara Cantalupo, Mauro Carrozzo, Daniele Cattaneo, Alessandro Celestini, Massimo Celino, Iacopo Colonnelli, Paolo Cretaro, Pasqua D'Ambra, Marco Danelutto, Roberto Esposito, Lionel Eyraud-Dubois, Antonio Filgueras, William Fornaciari, Ottorino Frezza, Andrea Galimberti, Francesco Giacomini, Brice Goglin, Daniele Gregori, Abdou Guermouche, Francesco Iannone, Michal Kulczewski, Francesca Lo Cicero, Alessandro Lonardo, Alberto R. Martinelli, Michele Martinelli, Xavier Martorell, Giuseppe Massari, Simone Montangero, Gianluca Mittone, Raymond Namyst, Ariel Oleksiak, Paolo Palazzari, Pier Stanislao Paolucci, Federico Reghenzani, Cristian Rossi, Sergio Saponara, Francesco Simula, Federico Terraneo, Samuel Thibault, Massimo Torquati, Matteo Turisini, Piero Vicini, Miquel Vidal, Davide Zoni, Giuseppe Zummo
Towards EXtreme scale technologies and accelerators for euROhpc hw/Sw supercomputing applications for exascale: The TEXTAROSSA approach Journal Article
In: Microprocessors and Microsystems, vol. 95, pp. 104679, 2022, ISSN: 0141-9331.
Abstract | Links | BibTeX | Tags: textarossa
@article{textarossa2022micpro:,
title = {Towards EXtreme scale technologies and accelerators for euROhpc hw/Sw supercomputing applications for exascale: The TEXTAROSSA approach},
author = {Giovanni Agosta and Marco Aldinucci and Carlos Alvarez and Roberto Ammendola and Yasir Arfat and Olivier Beaumont and Massimo Bernaschi and Andrea Biagioni and Tommaso Boccali and Berenger Bramas and Carlo Brandolese and Barbara Cantalupo and Mauro Carrozzo and Daniele Cattaneo and Alessandro Celestini and Massimo Celino and Iacopo Colonnelli and Paolo Cretaro and Pasqua D'Ambra and Marco Danelutto and Roberto Esposito and Lionel Eyraud-Dubois and Antonio Filgueras and William Fornaciari and Ottorino Frezza and Andrea Galimberti and Francesco Giacomini and Brice Goglin and Daniele Gregori and Abdou Guermouche and Francesco Iannone and Michal Kulczewski and Francesca Lo Cicero and Alessandro Lonardo and Alberto R. Martinelli and Michele Martinelli and Xavier Martorell and Giuseppe Massari and Simone Montangero and Gianluca Mittone and Raymond Namyst and Ariel Oleksiak and Paolo Palazzari and Pier Stanislao Paolucci and Federico Reghenzani and Cristian Rossi and Sergio Saponara and Francesco Simula and Federico Terraneo and Samuel Thibault and Massimo Torquati and Matteo Turisini and Piero Vicini and Miquel Vidal and Davide Zoni and Giuseppe Zummo},
doi = {10.1016/j.micpro.2022.104679},
issn = {0141-9331},
year = {2022},
date = {2022-01-01},
journal = {Microprocessors and Microsystems},
volume = {95},
pages = {104679},
abstract = {In the near future, Exascale systems will need to bridge three technology gaps to achieve high performance while remaining under tight power constraints: energy efficiency and thermal control; extreme computation efficiency via HW acceleration and new arithmetic; methods and tools for seamless integration of reconfigurable accelerators in heterogeneous HPC multi-node platforms. TEXTAROSSA addresses these gaps through a co-design approach to heterogeneous HPC solutions, supported by the integration and extension of HW and SW IPs, programming models, and tools derived from European research.},
keywords = {textarossa},
pubstate = {published},
tppubtype = {article}
}
Eduardo Quiñones, Jesus Perales, Jorge Ejarque, Asaf Badouh, Santiago Marco, Fabrice Auzanneau, François Galea, David González, José Ramón Hervás, Tatiana Silva, Iacopo Colonnelli, Barbara Cantalupo, Marco Aldinucci, Enzo Tartaglione, Rafael Tornero, José Flich, Jose Maria Martinez, David Rodriguez, Izan Catalán, Jorge Garcia, Carles Hernández
In: Terzo, Olivier, Martinovič, Jan (Ed.): HPC, Big Data, and AI Convergence Towards Exascale: Challenge and Vision, pp. 191–216, CRC Press, Boca Raton, Florida, 2022, ISBN: 978-1-0320-0984-1.
Abstract | Links | BibTeX | Tags: deephealth, streamflow
@incollection{22:deephealth:HPCbook,
title = {The DeepHealth HPC Infrastructure: Leveraging Heterogenous HPC and Cloud Computing Infrastructures for IA-based Medical Solutions},
author = {Eduardo Quiñones and Jesus Perales and Jorge Ejarque and Asaf Badouh and Santiago Marco and Fabrice Auzanneau and François Galea and David González and José Ramón Hervás and Tatiana Silva and Iacopo Colonnelli and Barbara Cantalupo and Marco Aldinucci and Enzo Tartaglione and Rafael Tornero and José Flich and Jose Maria Martinez and David Rodriguez and Izan Catalán and Jorge Garcia and Carles Hernández},
editor = {Olivier Terzo and Jan Martinovič},
url = {https://iris.unito.it/retrieve/handle/2318/1832050/912413/Preprint.pdf},
doi = {10.1201/9781003176664},
isbn = {978-1-0320-0984-1},
year = {2022},
date = {2022-01-01},
booktitle = {HPC, Big Data, and AI Convergence Towards Exascale: Challenge and Vision},
pages = {191–216},
publisher = {CRC Press},
address = {Boca Raton, Florida},
chapter = {10},
abstract = {This chapter presents the DeepHealth HPC toolkit for an efficient execution of deep learning (DL) medical application into HPC and cloud-computing infrastructures, featuring many-core, GPU, and FPGA acceleration devices. The toolkit offers to the European Computer Vision Library and the European Distributed Deep Learning Library (EDDL), developed in the DeepHealth project as well, the mechanisms to distribute and parallelize DL operations on HPC and cloud infrastructures in a fully transparent way. The toolkit implements workflow managers used to orchestrate HPC workloads for an efficient parallelization of EDDL training operations on HPC and cloud infrastructures, and includes the parallel programming models for an efficient execution EDDL inference and training operations on many-core, GPUs and FPGAs acceleration devices.},
keywords = {deephealth, streamflow},
pubstate = {published},
tppubtype = {incollection}
}
Dana Oniga, Barbara Cantalupo, Enzo Tartaglione, Daniele Perlo, Marco Grangetto, Marco Aldinucci, Federico Bolelli, Federico Pollastri, Michele Cancilla, Laura Canalini, Costantino Grana, Cristina Muñoz Alcalde, Franco Alberto Cardillo, Monica Florea
Applications of AI and HPC in the Health Domain Book Section
In: Terzo, Olivier, Martinovič, Jan (Ed.): HPC, Big Data, and AI Convergence Towards Exascale: Challenge and Vision, pp. 217–239, CRC Press, Boca Raton, Florida, 2022, ISBN: 978-1-0320-0984-1.
Abstract | Links | BibTeX | Tags: deephealth, streamflow
@incollection{22:applications:HPCbook,
title = {Applications of AI and HPC in the Health Domain},
author = {Dana Oniga and Barbara Cantalupo and Enzo Tartaglione and Daniele Perlo and Marco Grangetto and Marco Aldinucci and Federico Bolelli and Federico Pollastri and Michele Cancilla and Laura Canalini and Costantino Grana and Cristina Muñoz Alcalde and Franco Alberto Cardillo and Monica Florea},
editor = {Olivier Terzo and Jan Martinovič},
doi = {10.1201/9781003176664},
isbn = {978-1-0320-0984-1},
year = {2022},
date = {2022-01-01},
booktitle = {HPC, Big Data, and AI Convergence Towards Exascale: Challenge and Vision},
pages = {217–239},
publisher = {CRC Press},
address = {Boca Raton, Florida},
chapter = {11},
abstract = {This chapter presents the applications of artificial intelligence (AI) and high-computing performance (HPC) in the health domain, illustrated by the description of five of the use cases that are developed in the DeepHealth project. In the context of the European Commission supporting the use of AI and HPC in the health sector, DeepHealth Project is helping health experts process large quantities of images, putting at their disposal DeepLearning and computer vision techniques, combined in the DeepHealth toolkit and HPC infrastructures. The DeepHealth toolkit is tested and validated through 15 use cases, each of them representing a biomedical application. The most promising use cases are described in the chapter, which concludes with the value proposition and the benefits that DeepHealth toolkit offers to future end users.},
keywords = {deephealth, streamflow},
pubstate = {published},
tppubtype = {incollection}
}
Iacopo Colonnelli, Marco Aldinucci, Barbara Cantalupo, Luca Padovani, Sergio Rabellino, Concetto Spampinato, Roberto Morelli, Rosario Di Carlo, Nicolò Magini, Carlo Cavazzoni
Distributed workflows with Jupyter Journal Article
In: Future Generation Computer Systems, vol. 128, pp. 282–298, 2022, ISSN: 0167-739X.
Abstract | Links | BibTeX | Tags: across, deephealth, jupyter-workflow, streamflow
@article{21:FGCS:jupyflow,
title = {Distributed workflows with Jupyter},
author = {Iacopo Colonnelli and Marco Aldinucci and Barbara Cantalupo and Luca Padovani and Sergio Rabellino and Concetto Spampinato and Roberto Morelli and Rosario Di Carlo and Nicolò Magini and Carlo Cavazzoni},
url = {https://www.sciencedirect.com/science/article/pii/S0167739X21003976},
doi = {10.1016/j.future.2021.10.007},
issn = {0167-739X},
year = {2022},
date = {2022-01-01},
journal = {Future Generation Computer Systems},
volume = {128},
pages = {282–298},
abstract = {The designers of a new coordination interface enacting complex workflows have to tackle a dichotomy: choosing a language-independent or language-dependent approach. Language-independent approaches decouple workflow models from the host code's business logic and advocate portability. Language-dependent approaches foster flexibility and performance by adopting the same host language for business and coordination code. Jupyter Notebooks, with their capability to describe both imperative and declarative code in a unique format, allow taking the best of the two approaches, maintaining a clear separation between application and coordination layers but still providing a unified interface to both aspects. We advocate the Jupyter Notebooks' potential to express complex distributed workflows, identifying the general requirements for a Jupyter-based Workflow Management System (WMS) and introducing a proof-of-concept portable implementation working on hybrid Cloud-HPC infrastructures. As a byproduct, we extended the vanilla IPython kernel with workflow-based parallel and distributed execution capabilities. The proposed Jupyter-workflow (Jw) system is evaluated on common scenarios for High Performance Computing (HPC) and Cloud, showing its potential in lowering the barriers between prototypical Notebooks and production-ready implementations.},
keywords = {across, deephealth, jupyter-workflow, streamflow},
pubstate = {published},
tppubtype = {article}
}
2021
Giovanni Agosta, William Fornaciari, Andrea Galimberti, Giuseppe Massari, Federico Reghenzani, Federico Terraneo, Davide Zoni, Carlo Brandolese, Massimo Celino, Francesco Iannone, Paolo Palazzari, Giuseppe Zummo, Massimo Bernaschi, Pasqua D'Ambra, Sergio Saponara, Marco Danelutto, Massimo Torquati, Marco Aldinucci, Yasir Arfat, Barbara Cantalupo, Iacopo Colonnelli, Roberto Esposito, Alberto Riccardo Martinelli, Gianluca Mittone, Olivier Beaumont, Berenger Bramas, Lionel Eyraud-Dubois, Brice Goglin, Abdou Guermouche, Raymond Namyst, Samuel Thibault, Antonio Filgueras, Miquel Vidal, Carlos Alvarez, Xavier Martorell, Ariel Oleksiak, Michal Kulczewski, Alessandro Lonardo, Piero Vicini, Francesco Lo Cicero, Francesco Simula, Andrea Biagioni, Paolo Cretaro, Ottorino Frezza, Pier Stanislao Paolucci, Matteo Turisini, Francesco Giacomini, Tommaso Boccali, Simone Montangero, Roberto Ammendola
TEXTAROSSA: Towards EXtreme scale Technologies and Accelerators for euROhpc hw/Sw Supercomputing Applications for exascale Proceedings Article
In: Proc. of the 24th Euromicro Conference on Digital System Design (DSD), IEEE, Palermo, Italy, 2021.
Abstract | Links | BibTeX | Tags: streamflow, textarossa
@inproceedings{21:DSD:textarossa,
title = {TEXTAROSSA: Towards EXtreme scale Technologies and Accelerators for euROhpc hw/Sw Supercomputing Applications for exascale},
author = {Giovanni Agosta and William Fornaciari and Andrea Galimberti and Giuseppe Massari and Federico Reghenzani and Federico Terraneo and Davide Zoni and Carlo Brandolese and Massimo Celino and Francesco Iannone and Paolo Palazzari and Giuseppe Zummo and Massimo Bernaschi and Pasqua D'Ambra and Sergio Saponara and Marco Danelutto and Massimo Torquati and Marco Aldinucci and Yasir Arfat and Barbara Cantalupo and Iacopo Colonnelli and Roberto Esposito and Alberto Riccardo Martinelli and Gianluca Mittone and Olivier Beaumont and Berenger Bramas and Lionel Eyraud-Dubois and Brice Goglin and Abdou Guermouche and Raymond Namyst and Samuel Thibault and Antonio Filgueras and Miquel Vidal and Carlos Alvarez and Xavier Martorell and Ariel Oleksiak and Michal Kulczewski and Alessandro Lonardo and Piero Vicini and Francesco Lo Cicero and Francesco Simula and Andrea Biagioni and Paolo Cretaro and Ottorino Frezza and Pier Stanislao Paolucci and Matteo Turisini and Francesco Giacomini and Tommaso Boccali and Simone Montangero and Roberto Ammendola},
doi = {10.1109/DSD53832.2021.00051},
year = {2021},
date = {2021-08-01},
booktitle = {Proc. of the 24th Euromicro Conference on Digital System Design (DSD)},
publisher = {IEEE},
address = {Palermo, Italy},
abstract = {To achieve high performance and high energy effi- ciency on near-future exascale computing systems, three key technology gaps needs to be bridged. These gaps include: en- ergy efficiency and thermal control; extreme computation effi- ciency via HW acceleration and new arithmetics; methods and tools for seamless integration of reconfigurable accelerators in heterogeneous HPC multi-node platforms. TEXTAROSSA aims at tackling this gap through a co-design approach to heterogeneous HPC solutions, supported by the integration and extension of HW and SW IPs, programming models and tools derived from European research.},
keywords = {streamflow, textarossa},
pubstate = {published},
tppubtype = {inproceedings}
}
Marco Aldinucci, Valentina Cesare, Iacopo Colonnelli, Alberto Riccardo Martinelli, Gianluca Mittone, Barbara Cantalupo
Practical Parallelizazion of a Laplace Solver with MPI Proceedings Article
In: Iannone, Francesco (Ed.): ENEA CRESCO in the fight against COVID-19, pp. 21–24, ENEA, 2021.
Abstract | BibTeX | Tags: hpc4ai
@inproceedings{21:laplace:enea,
title = {Practical Parallelizazion of a Laplace Solver with MPI},
author = {Marco Aldinucci and Valentina Cesare and Iacopo Colonnelli and Alberto Riccardo Martinelli and Gianluca Mittone and Barbara Cantalupo},
editor = {Francesco Iannone},
year = {2021},
date = {2021-01-01},
booktitle = {ENEA CRESCO in the fight against COVID-19},
pages = {21–24},
publisher = {ENEA},
abstract = {This work exposes a practical methodology for the semi-automatic parallelization of existing code. We show how a scientific sequential code can be parallelized through our approach. The obtained parallel code is only slightly different from the starting sequential one, providing an example of how little re-designing our methodology involves. The performance of the parallelized code, executed on the CRESCO6 cluster, is then exposed and discussed. We also believe in the educational value of this approach and suggest its use as a teaching device for students.},
keywords = {hpc4ai},
pubstate = {published},
tppubtype = {inproceedings}
}
Iacopo Colonnelli, Barbara Cantalupo, Concetto Spampinato, Matteo Pennisi, Marco Aldinucci
Bringing AI pipelines onto cloud-HPC: setting a baseline for accuracy of COVID-19 diagnosis Proceedings Article
In: Iannone, Francesco (Ed.): ENEA CRESCO in the fight against COVID-19, ENEA, 2021.
Abstract | Links | BibTeX | Tags: streamflow
@inproceedings{21:covi:enea,
title = {Bringing AI pipelines onto cloud-HPC: setting a baseline for accuracy of COVID-19 diagnosis},
author = {Iacopo Colonnelli and Barbara Cantalupo and Concetto Spampinato and Matteo Pennisi and Marco Aldinucci},
editor = {Francesco Iannone},
url = {https://iris.unito.it/retrieve/handle/2318/1796029/779853/21_AI-pipelines_ENEA-COVID19.pdf},
doi = {10.5281/zenodo.5151511},
year = {2021},
date = {2021-01-01},
booktitle = {ENEA CRESCO in the fight against COVID-19},
publisher = {ENEA},
abstract = {HPC is an enabling platform for AI. The introduction of AI workloads in the HPC applications basket has non-trivial consequences both on the way of designing AI applications and on the way of providing HPC computing. This is the leitmotif of the convergence between HPC and AI. The formalized definition of AI pipelines is one of the milestones of HPC-AI convergence. If well conducted, it allows, on the one hand, to obtain portable and scalable applications. On the other hand, it is crucial for the reproducibility of scientific pipelines. In this work, we advocate the StreamFlow Workflow Management System as a crucial ingredient to define a parametric pipeline, called ``CLAIRE COVID-19 Universal Pipeline'', which is able to explore the optimization space of methods to classify COVID-19 lung lesions from CT scans, compare them for accuracy, and therefore set a performance baseline. The universal pipeline automatizes the training of many different Deep Neural Networks (DNNs) and many different hyperparameters. It, therefore, requires a massive computing power, which is found in traditional HPC infrastructure thanks to the portability-by-design of pipelines designed with StreamFlow. Using the universal pipeline, we identified a DNN reaching over 90% accuracy in detecting COVID-19 lesions in CT scans.},
keywords = {streamflow},
pubstate = {published},
tppubtype = {inproceedings}
}
Yasir Arfat, Gianluca Mittone, Roberto Esposito, Barbara Cantalupo, Gaetano Maria De Ferrari, Marco Aldinucci
A Review of Machine Learning for Cardiology Journal Article
In: Minerva cardiology and angiology, 2021.
Abstract | Links | BibTeX | Tags: deephealth, hpc4ai
@article{21:ai4numbers:minerva,
title = {A Review of Machine Learning for Cardiology},
author = {Yasir Arfat and Gianluca Mittone and Roberto Esposito and Barbara Cantalupo and Gaetano Maria De Ferrari and Marco Aldinucci},
url = {https://iris.unito.it/retrieve/handle/2318/1796298/780512/21_AI4numbers-preprint.pdf},
doi = {10.23736/s2724-5683.21.05709-4},
year = {2021},
date = {2021-01-01},
journal = {Minerva cardiology and angiology},
abstract = {This paper reviews recent cardiology literature and reports how Artificial Intelligence Tools (specifically, Machine Learning techniques) are being used by physicians in the field. Each technique is introduced with enough details to allow the understanding of how it works and its intent, but without delving into details that do not add immediate benefits and require expertise in the field. We specifically focus on the principal Machine Learning based risk scores used in cardiovascular research. After introducing them and summarizing their assumptions and biases, we discuss their merits and shortcomings. We report on how frequently they are adopted in the field and suggest why this is the case based on our expertise in Machine Learning. We complete the analysis by reviewing how corresponding statistical approaches compare with them. Finally, we discuss the main open issues in applying Machine Learning tools to cardiology tasks, also drafting possible future directions. Despite the growing interest in these tools, we argue that there are many still underutilized techniques: while Neural Networks are slowly being incorporated in cardiovascular research, other important techniques such as Semi-Supervised Learning and Federated Learning are still underutilized. The former would allow practitioners to harness the information contained in large datasets that are only partially labeled, while the latter would foster collaboration between institutions allowing building larger and better models.},
keywords = {deephealth, hpc4ai},
pubstate = {published},
tppubtype = {article}
}
Marco Aldinucci, Valentina Cesare, Iacopo Colonnelli, Alberto Riccardo Martinelli, Gianluca Mittone, Barbara Cantalupo, Carlo Cavazzoni, Maurizio Drocco
Practical Parallelization of Scientific Applications with OpenMP, OpenACC and MPI Journal Article
In: Journal of Parallel and Distributed Computing, vol. 157, pp. 13–29, 2021.
Abstract | Links | BibTeX | Tags: HPC
@article{21:jpdc:loop,
title = {Practical Parallelization of Scientific Applications with OpenMP, OpenACC and MPI},
author = {Marco Aldinucci and Valentina Cesare and Iacopo Colonnelli and Alberto Riccardo Martinelli and Gianluca Mittone and Barbara Cantalupo and Carlo Cavazzoni and Maurizio Drocco},
url = {https://iris.unito.it/retrieve/handle/2318/1792557/770851/Practical_Parallelization_JPDC_preprint.pdf},
doi = {10.1016/j.jpdc.2021.05.017},
year = {2021},
date = {2021-01-01},
journal = {Journal of Parallel and Distributed Computing},
volume = {157},
pages = {13–29},
abstract = {This work aims at distilling a systematic methodology to modernize existing sequential scientific codes with a little re-designing effort, turning an old codebase into emphmodern code, i.e., parallel and robust code. We propose a semi-automatic methodology to parallelize scientific applications designed with a purely sequential programming mindset, possibly using global variables, aliasing, random number generators, and stateful functions. We demonstrate that the same methodology works for the parallelization in the shared memory model (via OpenMP), message passing model (via MPI), and General Purpose Computing on GPU model (via OpenACC). The method is demonstrated parallelizing four real-world sequential codes in the domain of physics and material science. The methodology itself has been distilled in collaboration with MSc students of the Parallel Computing course at the University of Torino, that applied it for the first time to the project works that they presented for the final exam of the course. Every year the course hosts some special lectures from industry representatives, who present how they use parallel computing and offer codes to be parallelized.},
keywords = {HPC},
pubstate = {published},
tppubtype = {article}
}
Iacopo Colonnelli, Barbara Cantalupo, Roberto Esposito, Matteo Pennisi, Concetto Spampinato, Marco Aldinucci
HPC Application Cloudification: The StreamFlow Toolkit Proceedings Article
In: Bispo, João, Cherubin, Stefano, Flich, José (Ed.): 12th Workshop on Parallel Programming and Run-Time Management Techniques for Many-core Architectures and 10th Workshop on Design Tools and Architectures for Multicore Embedded Computing Platforms (PARMA-DITAM 2021), pp. 5:1–5:13, Schloss Dagstuhl – Leibniz-Zentrum für Informatik, Dagstuhl, Germany, 2021, ISSN: 2190-6807.
Abstract | Links | BibTeX | Tags: deephealth, hpc4ai, streamflow
@inproceedings{colonnelli_et_al:OASIcs.PARMA-DITAM.2021.5,
title = {HPC Application Cloudification: The StreamFlow Toolkit},
author = {Iacopo Colonnelli and Barbara Cantalupo and Roberto Esposito and Matteo Pennisi and Concetto Spampinato and Marco Aldinucci},
editor = {João Bispo and Stefano Cherubin and José Flich},
url = {https://drops.dagstuhl.de/opus/volltexte/2021/13641/pdf/OASIcs-PARMA-DITAM-2021-5.pdf},
doi = {10.4230/OASIcs.PARMA-DITAM.2021.5},
issn = {2190-6807},
year = {2021},
date = {2021-01-01},
booktitle = {12th Workshop on Parallel Programming and Run-Time Management Techniques for Many-core Architectures and 10th Workshop on Design Tools and Architectures for Multicore Embedded Computing Platforms (PARMA-DITAM 2021)},
volume = {88},
pages = {5:1–5:13},
publisher = {Schloss Dagstuhl – Leibniz-Zentrum für Informatik},
address = {Dagstuhl, Germany},
series = {Open Access Series in Informatics (OASIcs)},
abstract = {Finding an effective way to improve accessibility to High-Performance Computing facilities, still anchored to SSH-based remote shells and queue-based job submission mechanisms, is an open problem in computer science. This work advocates a cloudification of HPC applications through a cluster-as-accelerator pattern, where computationally demanding portions of the main execution flow hosted on a Cloud Finding an effective way to improve accessibility to High-Performance Computing facilities, still anchored to SSH-based remote shells and queue-based job submission mechanisms, is an open problem in computer science. This work advocates a cloudification of HPC applications through a cluster-as-accelerator pattern, where computationally demanding portions of the main execution flow hosted on a Cloud infrastructure can be offloaded to HPC environments to speed them up. We introduce StreamFlow, a novel Workflow Management System that supports such a design pattern and makes it possible to run the steps of a standard workflow model on independent processing elements with no shared storage. We validated the proposed approach's effectiveness on the CLAIRE COVID-19 universal pipeline, i.e. a reproducible workflow capable of automating the comparison of (possibly all) state-of-the-art pipelines for the diagnosis of COVID-19 interstitial pneumonia from CT scans images based on Deep Neural Networks (DNNs).},
keywords = {deephealth, hpc4ai, streamflow},
pubstate = {published},
tppubtype = {inproceedings}
}
Fabrizio D'Ascenzo, Ovidio De Filippo, Guglielmo Gallone, Gianluca Mittone, Marco Agostino Deriu, Mario Iannaccone, Albert Ariza-Solé, Christoph Liebetrau, Sergio Manzano-Fernández, Giorgio Quadri, Tim Kinnaird, Gianluca Campo, Jose Paulo Simao Henriques, James M Hughes, Alberto Dominguez-Rodriguez, Marco Aldinucci, Umberto Morbiducci, Giuseppe Patti, Sergio Raposeiras-Roubin, Emad Abu-Assi, Gaetano Maria De Ferrari, Francesco Piroli, Andrea Saglietto, Federico Conrotto, Pierluigi Omedé, Antonio Montefusco, Mauro Pennone, Francesco Bruno, Pier Paolo Bocchino, Giacomo Boccuzzi, Enrico Cerrato, Ferdinando Varbella, Michela Sperti, Stephen B. Wilton, Lazar Velicki, Ioanna Xanthopoulou, Angel Cequier, Andres Iniguez-Romo, Isabel Munoz Pousa, Maria Cespon Fernandez, Berenice Caneiro Queija, Rafael Cobas-Paz, Angel Lopez-Cuenca, Alberto Garay, Pedro Flores Blanco, Andrea Rognoni, Giuseppe Biondi Zoccai, Simone Biscaglia, Ivan Nunez-Gil, Toshiharu Fujii, Alessandro Durante, Xiantao Song, Tetsuma Kawaji, Dimitrios Alexopoulos, Zenon Huczek, Jose Ramon Gonzalez Juanatey, Shao-Ping Nie, Masa-aki Kawashiri, Iacopo Colonnelli, Barbara Cantalupo, Roberto Esposito, Sergio Leonardi, Walter Grosso Marra, Alaide Chieffo, Umberto Michelucci, Dario Piga, Marta Malavolta, Sebastiano Gili, Marco Mennuni, Claudio Montalto, Luigi Oltrona Visconti, Yasir Arfat
Machine learning-based prediction of adverse events following an acute coronary syndrome (PRAISE): a modelling study of pooled datasets Journal Article
In: The Lancet, vol. 397, no. 10270, pp. 199–207, 2021, ISSN: 0140-6736.
Abstract | Links | BibTeX | Tags: deephealth, hpc4ai
@article{21:lancet,
title = {Machine learning-based prediction of adverse events following an acute coronary syndrome (PRAISE): a modelling study of pooled datasets},
author = {Fabrizio D'Ascenzo and Ovidio De Filippo and Guglielmo Gallone and Gianluca Mittone and Marco Agostino Deriu and Mario Iannaccone and Albert Ariza-Solé and Christoph Liebetrau and Sergio Manzano-Fernández and Giorgio Quadri and Tim Kinnaird and Gianluca Campo and Jose Paulo Simao Henriques and James M Hughes and Alberto Dominguez-Rodriguez and Marco Aldinucci and Umberto Morbiducci and Giuseppe Patti and Sergio Raposeiras-Roubin and Emad Abu-Assi and Gaetano Maria De Ferrari and Francesco Piroli and Andrea Saglietto and Federico Conrotto and Pierluigi Omedé and Antonio Montefusco and Mauro Pennone and Francesco Bruno and Pier Paolo Bocchino and Giacomo Boccuzzi and Enrico Cerrato and Ferdinando Varbella and Michela Sperti and Stephen B. Wilton and Lazar Velicki and Ioanna Xanthopoulou and Angel Cequier and Andres Iniguez-Romo and Isabel Munoz Pousa and Maria Cespon Fernandez and Berenice Caneiro Queija and Rafael Cobas-Paz and Angel Lopez-Cuenca and Alberto Garay and Pedro Flores Blanco and Andrea Rognoni and Giuseppe Biondi Zoccai and Simone Biscaglia and Ivan Nunez-Gil and Toshiharu Fujii and Alessandro Durante and Xiantao Song and Tetsuma Kawaji and Dimitrios Alexopoulos and Zenon Huczek and Jose Ramon Gonzalez Juanatey and Shao-Ping Nie and Masa-aki Kawashiri and Iacopo Colonnelli and Barbara Cantalupo and Roberto Esposito and Sergio Leonardi and Walter Grosso Marra and Alaide Chieffo and Umberto Michelucci and Dario Piga and Marta Malavolta and Sebastiano Gili and Marco Mennuni and Claudio Montalto and Luigi Oltrona Visconti and Yasir Arfat},
url = {https://www.researchgate.net/profile/James_Hughes3/publication/348501148_Machine_learning-based_prediction_of_adverse_events_following_an_acute_coronary_syndrome_PRAISE_a_modelling_study_of_pooled_datasets/links/6002a81ba6fdccdcb858b6c2/Machine-learning-based-prediction-of-adverse-events-following-an-acute-coronary-syndrome-PRAISE-a-modelling-study-of-pooled-datasets.pdf},
doi = {10.1016/S0140-6736(20)32519-8},
issn = {0140-6736},
year = {2021},
date = {2021-01-01},
journal = {The Lancet},
volume = {397},
number = {10270},
pages = {199–207},
abstract = {Background The accuracy of current prediction tools for ischaemic and bleeding events after an acute coronary syndrome (ACS) remains insufficient for individualised patient management strategies. We developed a machine learning-based risk stratification model to predict all-cause death, recurrent acute myocardial infarction, and major bleeding after ACS. Methods Different machine learning models for the prediction of 1-year post-discharge all-cause death, myocardial infarction, and major bleeding (defined as Bleeding Academic Research Consortium type 3 or 5) were trained on a cohort of 19826 adult patients with ACS (split into a training cohort [80%] and internal validation cohort [20%]) from the BleeMACS and RENAMI registries, which included patients across several continents. 25 clinical features routinely assessed at discharge were used to inform the models. The best-performing model for each study outcome (the PRAISE score) was tested in an external validation cohort of 3444 patients with ACS pooled from a randomised controlled trial and three prospective registries. Model performance was assessed according to a range of learning metrics including area under the receiver operating characteristic curve (AUC). Findings The PRAISE score showed an AUC of 0.82 (95% CI 0.78-0.85) in the internal validation cohort and 0.92 (0.90-0.93) in the external validation cohort for 1-year all-cause death; an AUC of 0.74 (0.70-0.78) in the internal validation cohort and 0.81 (0.76-0.85) in the external validation cohort for 1-year myocardial infarction; and an AUC of 0.70 (0.66-0.75) in the internal validation cohort and 0.86 (0.82-0.89) in the external validation cohort for 1-year major bleeding. Interpretation A machine learning-based approach for the identification of predictors of events after an ACS is feasible and effective. The PRAISE score showed accurate discriminative capabilities for the prediction of all-cause death, myocardial infarction, and major bleeding, and might be useful to guide clinical decision making.},
keywords = {deephealth, hpc4ai},
pubstate = {published},
tppubtype = {article}
}
Iacopo Colonnelli, Barbara Cantalupo, Ivan Merelli, Marco Aldinucci
StreamFlow: cross-breeding cloud with HPC Journal Article
In: IEEE Transactions on Emerging Topics in Computing, vol. 9, no. 4, pp. 1723–1737, 2021.
Abstract | Links | BibTeX | Tags: deephealth, hpc4ai, streamflow
@article{20Lstreamflow:tetc,
title = {StreamFlow: cross-breeding cloud with HPC},
author = {Iacopo Colonnelli and Barbara Cantalupo and Ivan Merelli and Marco Aldinucci},
url = {https://arxiv.org/pdf/2002.01558},
doi = {10.1109/TETC.2020.3019202},
year = {2021},
date = {2021-01-01},
journal = {IEEE Transactions on Emerging Topics in Computing},
volume = {9},
number = {4},
pages = {1723–1737},
abstract = {Workflows are among the most commonly used tools in a variety of execution environments. Many of them target a specific environment; few of them make it possible to execute an entire workflow in different environments, e.g. Kubernetes and batch clusters. We present a novel approach to workflow execution, called StreamFlow, that complements the workflow graph with the declarative description of potentially complex execution environments, and that makes it possible the execution onto multiple sites not sharing a common data space. StreamFlow is then exemplified on a novel bioinformatics pipeline for single cell transcriptomic data analysis workflow.},
keywords = {deephealth, hpc4ai, streamflow},
pubstate = {published},
tppubtype = {article}
}
Talks
2023
Barbara Cantalupo
The Software Heritage Analytics Framework Miscellaneous
Admire User Meeting, 2023, 2023.
Abstract | Links | BibTeX | Tags: admire
@misc{23:ADMIRE:UG-SHA,
title = {The Software Heritage Analytics Framework},
author = {Barbara Cantalupo},
url = {https://datacloud.di.unito.it/index.php/s/NbNDPaGZkDc3KyN},
year = {2023},
date = {2023-12-01},
address = {Barcelona Supercomputing Centre, Barcelona, Spain},
abstract = {A framework for analytics on top of Software Heritage},
howpublished = {Admire User Meeting, 2023},
keywords = {admire},
pubstate = {published},
tppubtype = {misc}
}
Iacopo Colonnelli, Doriana Medić, Barbara Cantalupo, Marco Aldinucci
Università degli Studi di Torino: Alpha parallel research group Miscellaneous
HaMMon Kick-Off meeting, 2023.
Links | BibTeX | Tags: icsc, streamflow
@misc{23:HaMMonProject,
title = {Università degli Studi di Torino: Alpha parallel research group},
author = {Iacopo Colonnelli and Doriana Medić and Barbara Cantalupo and Marco Aldinucci},
url = {https://datacloud.di.unito.it/index.php/s/cmgy9BZ3nwCR2QJ},
year = {2023},
date = {2023-10-01},
address = {Bologna, Italy},
howpublished = {HaMMon Kick-Off meeting},
keywords = {icsc, streamflow},
pubstate = {published},
tppubtype = {misc}
}
2022
Iacopo Colonnelli, Barbara Cantalupo, Doriana Medić, Marco Aldinucci
Hybrid workflows for heterogeneous distributed computing Miscellaneous
3rd Italian Workshop on HPC (ITWSHPC), 2022.
Links | BibTeX | Tags: across, admire, eumaster4hpc, eupex, eupilot, textarossa
@misc{22:itwshpc,
title = {Hybrid workflows for heterogeneous distributed computing},
author = {Iacopo Colonnelli and Barbara Cantalupo and Doriana Medić and Marco Aldinucci},
url = {https://datacloud.di.unito.it/index.php/s/ienbcA2DJ26aioE},
year = {2022},
date = {2022-09-01},
address = {Torino, Italy},
howpublished = {3rd Italian Workshop on HPC (ITWSHPC)},
keywords = {across, admire, eumaster4hpc, eupex, eupilot, textarossa},
pubstate = {published},
tppubtype = {misc}
}